Timothy recruited three people (his father Patrick Lourdasamy, his father’s friend Kamaraj Gopal Krishnan, and his friend Daniel Lars Stevenson) to open bank accounts that received over $1.3 million in scam proceeds.
The scheme worked as follows:
- Timothy met a man named “Harry Turner” at a Boat Quay pub around 2017-2018
- Turner proposed a scheme where “consultants” would set up sole proprietorships and open bank accounts
- Money would be transferred to these accounts, and the consultants would withdraw it, taking a 3% cut
- Timothy would take another 2% and pass the remaining funds to Turner’s associates
The victims included two Australians who were defrauded in an investment scam. The scam transferred nearly $913,000 to Patrick’s company account, over $63,000 to Kamaraj’s company account, and more than $338,000 to Stevenson’s company account.
The sentences handed down were:
- Timothy Solomon Patrick: 3½ years in jail
- Patrick Lourdasamy (father): 10 months in jail
- Kamaraj Gopal Krishnan: 12 weeks in jail
- Daniel Lars Stevenson: case still pending
Timothy was aware of the suspicious nature of the arrangement and collaborated with Stevenson to submit fake documents to a bank when questioned about a large withdrawal. He’s currently on bail for $20,000 and expected to begin his sentence on April 17, 2025.
Analysis of the Bank Account Money Mule Scam
Scam Structure and Mechanics
This case represents a classic money mule operation with several distinct elements:
- Recruitment Chain:
- A “mastermind” (Harry Turner) who likely doesn’t exist under that name
- A primary recruiter (Timothy) who brings in secondary mules
- Multiple account holders (Patrick, Kamaraj, and Daniel) who provide the banking infrastructure
- Corporate Legitimacy Layer:
- Creation of business entities like “Frontier Global Trade & Consultancy” and “Premier International Trade & Consultancy”
- These companies provided a veneer of legitimacy for large international transfers
- Financial Flow:
- Victims abroad (Australians) transferred funds to Singapore-based accounts
- Money was quickly withdrawn and dispersed to minimize tracing
- A commission structure (3% for account holders, 2% for the recruiter) ensured all participants had financial incentives
- Documentation Fraud:
- When questioned by banks, participants created fictitious documents to explain suspicious transactions
- This demonstrates premeditated intent to deceive financial institutions
Red Flags Present in This Case
Several warning signs were apparent:
- The vague “consultancy services” with no transparent business model
- The recruiter’s acknowledgment that the earnings were “too good to be true”
- The rapid movement of large sums through accounts
- The need to submit fabricated documentation to banks
- The use of multiple companies and accounts for similar transactions
Anti-Scam Measures in Singapore
Singapore has implemented robust measures to combat such scams:
Legal Framework
- Penalties and Enforcement:
- Severe jail terms for money mule activities, as seen in this case
- The Organised Crime Act provides tools to prosecute criminal networks
- The Payment Services Act regulates digital payment services
- Anti-Money Laundering Regulations:
- Monetary Authority of Singapore (MAS) imposes strict Know Your Customer (KYC) requirements
- Banks must report suspicious transactions under the Corruption, Drug Trafficking and Other Serious Crimes Act
Technological Solutions
- ScamShield App:
- Government-developed app that blocks known scam calls and messages
- Uses machine learning to identify potential scam attempts
- Bank Transaction Controls:
- Default transaction limits on digital banking platforms
- Cooling-off periods for adding new payees
- SMS/app notifications for unusual transactions
Public Education Initiatives
- National Crime Prevention Council Campaigns:
- “Spot the Signs, Stop the Crimes” awareness campaign
- Educational resources explaining standard scam methodologies
- Inter-Ministry Collaboration:
- Anti-Scam Centre coordinates between police, financial institutions, and telecommunications companies
- Public-private partnerships for faster information sharing
- Scam Alert Website and Hotline:
- Centralized reporting system for potential scams
- Regular updates on emerging scam trends
Financial Industry Safeguards
- Enhanced Transaction Monitoring:
- As evidenced in this case when the bank flagged a large withdrawal
- AI-powered systems to detect unusual patterns in account activity
- Account Opening Due Diligence:
- Stricter verification processes for business accounts
- Regular review of high-risk accounts
Areas for Improvement
Despite these measures, this case highlights ongoing challenges:
- Cross-Border Coordination:
- International scams require better cooperation between jurisdictions
- The Australian victims had limited recourse once money left their country
- Company Registration Scrutiny:
- More thorough vetting of newly formed companies with international transactions
- Regular audits of small consultancies with vast cash flows
- Public Awareness:
- Continued education about the legal consequences of being a money mule
- Clear communication that “too good to be true” financial opportunities are typically fraudulent
This type of sophisticated scam demonstrates why Singapore continues to strengthen its anti-scam ecosystem through a combination of legislation, technology, public education, and international cooperation.
Methods to Prevent Money Muling in Singapore
Regulatory and Banking Measures
Transaction Monitoring Systems
- Real-time transaction monitoring utilizing AI to detect unusual patterns
- Intelligent systems flagging rapid deposits followed by withdrawals
- Automated alerts for transactions involving high-risk jurisdictions
- Velocity checks that identify unusual account activity rates
Account Opening Procedures
- Enhanced due diligence for new accounts, especially business accounts
- Biometric verification requirements for both in-person and digital onboarding
- Document authentication technology to verify identification documents
- Risk-based approach with additional scrutiny for higher-risk profiles
Banking Controls
- Delayed processing for extensive transactions
- Mandatory cooling periods before new payees can receive large transfers
- Default transaction limits requiring additional verification to increase
- Call-back verification for transactions above certain thresholds
Public Education and Awareness
Targeted Campaigns
- “Don’t Be A Money Mule” awareness programs in schools and universities
- Community outreach focusing on vulnerable populations (students, elderly, job seekers)
- Multi-language campaigns reaching diverse communities
- Specific education on the legal consequences, including jail time
Warning Systems
- SMS alerts about standard money mule recruitment techniques
- In-app banking notifications about the risks of allowing account access
- Digital banking platform pop-up warnings about suspicious transaction patterns
- Community alerts when new money mule recruitment tactics are detected
Law Enforcement Strategies
Proactive Investigation
- Dedicated Anti-Scam Centre resources for money mule network detection
- Collaboration between police and financial intelligence units
- Data analytics to identify connected accounts and transaction patterns
- Regular auditing of newly formed companies with unusual transaction patterns
Deterrence Through Prosecution
- Publicized cases showing severe penalties for money mules
- Prosecution of all participants in the chain, not just ring leaders
- Asset recovery procedures to trace and seize criminal proceeds
- Clear communication that ignorance is not an acceptable legal defense
Industry Collaboration
Information Sharing Networks
- Bank consortium sharing intelligence on suspicious patterns
- Cross-industry collaboration between telcos, banks, and payment providers
- A centralized database of known mule accounts and recruitment techniques
- Public-private partnerships for faster response to emerging threats
Technology Solutions
- Shared fraud detection engines across multiple financial institutions
- Digital footprint analysis (device, location, and behavioral data)
- Blockchain analytics to trace fund movements across platforms
- API-based systems allowing real-time information exchange between institutions
Targeted Vulnerability Reduction
Job Seeker Protection
- Partnership with job platforms to detect and remove suspicious job listings
- Education about legitimate versus suspicious job offers
- Warning systems on employment platforms
- Verification processes for companies advertising “financial agent” positions
Student-Focused Programs
- Campus awareness campaigns highlighting the risks
- Financial literacy modules covering scams and money mule awareness
- University partnerships with financial institutions for education
- Peer-to-peer awareness programs led by students themselves
International Cooperation
Cross-Border Coordination
- Information sharing agreements with other jurisdictions
- Joint investigation teams for transnational cases
- Standardized reporting formats for suspicious transaction reporting
- Rapid freeze mechanisms for cross-border fund movements
Regional Intelligence Framework
- ASEAN-wide cooperation on money laundering typologies
- Coordinated action against known crime groups operating regionally
- Harmonized KYC standards across regional financial institutions
- Shared blacklists of suspicious entities across borders
The most effective prevention strategy combines these approaches with continuous adaptation as criminals evolve their tactics. Singapore’s multi-agency approach involving the MAS, Singapore Police Force, and private sector partners has shown promising results, but ongoing vigilance remains essential.
Essential Fraud Detection Strategies
1. Multi-layered Authentication Systems
Modern authentication must go beyond passwords. A robust system should incorporate:
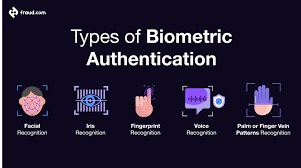
Biometric Verification using fingerprints, facial recognition, or voice authentication adds a physical dimension to security that’s difficult to replicate.
Device Intelligence examines the devices used to access accounts, flagging suspicious logins from unfamiliar devices or locations.
Behavioral Biometrics analyzes patterns in how users interact with devices—how they type, swipe, or navigate—creating a behavioral fingerprint that’s hard for fraudsters to mimic.
2. Machine Learning and AI Detection Systems
Artificial intelligence has transformed fraud detection from rules-based systems to sophisticated pattern recognition:
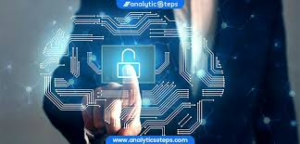
Anomaly Detection algorithms establish baseline behaviours for users and flag deviations from standard patterns. For example, if a user who typically makes small, local purchases suddenly attempts large international transactions, the system can automatically flag this for review.
Predictive Analytics examines historical fraud patterns to forecast potential vulnerabilities. These systems become increasingly accurate over time as they process more data and fraud scenarios.
Adaptive Authentication dynamically adjusts security requirements based on risk assessment. Low-risk transactions might proceed with minimal friction, while high-risk activities trigger additional verification steps.
3. Real-time Transaction Monitoring
Modern fraud detection must operate at the speed of digital transactions:
Velocity Checks look for suspicious patterns in the frequency of activities, such as multiple account creation attempts or rapid-fire transactions.
Network Analysis examines connections between accounts, identifying clusters of potentially fraudulent activity that might indicate organized fraud rings.
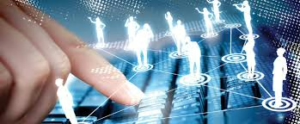
Geolocation Verification checks whether transaction locations make logical sense given a user’s history and profile.
4. Data Integration and Cross-channel Analysis
Effective fraud detection requires a holistic view across all channels and touchpoints:
Unified Customer Profiles combine data from various sources—mobile apps, websites, call centres, and physical locations—to create a comprehensive view of customer behaviour.
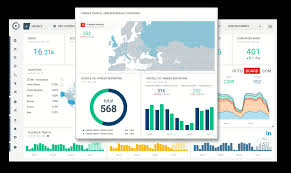
Cross-channel Pattern Recognition identifies suspicious activities that might appear normal when viewed in isolation but reveal fraud patterns when examined across channels.
Third-party Data Enrichment augments internal data with external information such as device reputation databases, known fraud networks, and compromised credential lists.
5. Advanced Analytics Tools: Implementation Examples
Let’s look at how these strategies might be implemented in practice with code examples:
Machine Learning Fraud Detection System
Click to open the code
Tap to open
This code example demonstrates several key concepts in fraud detection:
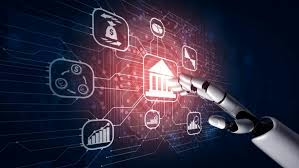
- Feature Engineering: The system calculates derived features that are strong fraud indicators, such as distance from home location, unusual transaction amounts compared to user history, and temporal patterns.
- Risk-based Decision Making: Rather than a binary approve/decline decision, the system implements a spectrum of responses based on both the risk score and transaction context.
- Machine Learning Implementation: The Random Forest model can capture complex, non-linear relationships between features and fraud likelihood, making it practical for detecting sophisticated fraud patterns.
- Explainability: The system analyzes feature importance, providing insight into which factors most strongly indicate fraud—crucial for improving the system and explaining decisions to customers and regulators.
6. Behavioral Analytics
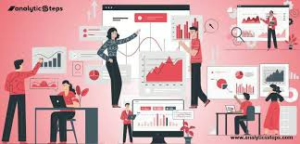
Beyond transaction details, modern fraud systems analyze how users behave:
Session Analysis examines user interaction patterns during a session, such as navigation paths, interaction speed, and hesitation points. Fraudsters often exhibit different behaviours than legitimate users when navigating financial interfaces.
Typing Patterns can reveal when a different person is using familiar credentials. Legitimate users develop consistent typing rhythms and patterns that are difficult to replicate.
Usage Consistency looks at whether behaviour matches patterns. For example, a user who constantly carefully reviews transaction details before confirming might raise flags if they suddenly rush through multiple high-value transactions.
7. Collaborative Security Approaches
Fraud detection is strengthened through industry cooperation:
Consortium Data Sharing allows financial institutions to pool anonymized fraud data, creating a more comprehensive picture of emerging threats while preserving customer privacy.
Regulatory Cooperation enables institutions to work with government agencies to identify large-scale fraud operations and money laundering networks.
Vendor Integration leverages specialized third-party security services that focus exclusively on specific types of fraud detection, adding another layer of protection.
Implementation Challenges and Solutions
Implementing fraud detection systems comes with significant challenges:
False Positives create friction for legitimate customers and can damage trust. Solutions include:
- Implementing risk-based authentication that adds friction only when necessary
- Using ensemble models that combine multiple detection approaches for greater accuracy
- Continuously tuning systems based on customer feedback and false positive analysis
Data Privacy Regulations such as GDPR and CCPA restrict how customer data can be used. Consider:
- Implementing privacy-by-design principles in fraud systems
- Using anonymization and pseudonymization techniques
- Creating clear data governance frameworks with documented legitimate interest in fraud prevention
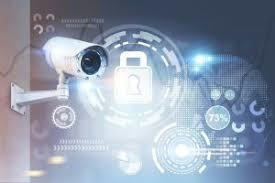
Integration Complexity across legacy and modern systems can impede effectiveness. Address this by:
- Using API-first approaches for system integration
- Implementing data transformation layers to normalize inputs from different systems
- Creating real-time event streams for fraud data rather than batch processing
Building a Fraud Prevention Culture
Technical solutions are only part of effective fraud prevention:
Employee Training should ensure that all staff members understand their role in preventing fraud, recognizing warning signs, and following security protocols.
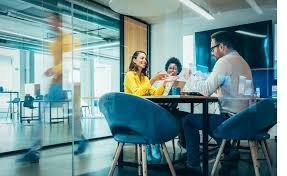
Customer Education helps users protect themselves by recognizing phishing attempts, using strong authentication methods, and understanding how to report suspicious activities.
Regular Testing through penetration testing, red team exercises, and fraud simulations helps identify vulnerabilities before criminals can exploit them.
Measuring and Improving Your Fraud Detection System
Continuous improvement requires careful measurement:
Key Performance Indicators should include:
- False positive rate: Legitimate transactions incorrectly flagged
- False negative rate: Fraudulent transactions missed
- Detection speed: Time from fraud attempt to detection
- Customer impact metrics: Authentication success rates and friction points
A/B Testing allows you to compare different detection approaches and fine-tune systems based on real-world results rather than theoretical models.
Post-incident analysis should thoroughly examine confirmed fraud cases to identify how detection could have happened earlier or more efficiently.
Conclusion
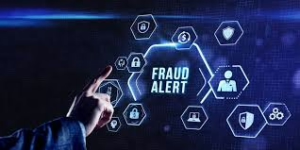
As fintech continues to transform the financial landscape, fraud detection must remain a top priority for businesses. By implementing multi-layered approaches that combine advanced technologies with human expertise, fintech companies can protect both their customers and their bottom line.
Remember that effective fraud prevention is not a static solution but an ongoing process that must continuously evolve to address new threats. By staying vigilant and investing in robust detection systems, your business can build customer trust while minimizing losses in an increasingly digital financial world.
Secure browsing
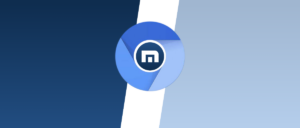
When it comes to staying safe online, using a secure and private browser is crucial. Such a browser can help protect your personal information and keep you safe from cyber threats. One option that offers these features is the Maxthon Browser, which is available for free. It comes with built-in Adblock and anti-tracking software to enhance your browsing privacy.
By utilising the Maxthon Browser, users can browse the internet confidently, knowing that their online activities are shielded from prying eyes. The integrated security features alleviate concerns about potential privacy breaches and ensure a safer browsing environment. Furthermore, the browser’s user-friendly interface makes it easy for individuals to customise their privacy settings according to their preferences.
Maxthon Browser not only delivers a seamless browsing experience but also prioritises the privacy and security of its users through its efficient ad-blocking and anti-tracking capabilities. With these protective measures in place, users can enjoy the internet while feeling reassured about their online privacy.
In addition, the desktop version of Maxthon Browser works seamlessly with their VPN, providing an extra layer of security. By using this browser, you can minimise the risk of encountering online threats and enjoy a safer internet experience. With its combination of security features, Maxthon Browser aims to provide users with peace of mind while they browse.
Maxthon Browser is a reliable choice for users who prioritise privacy and security. With its robust encryption measures and extensive privacy settings, it offers a secure browsing experience that gives users peace of mind. The browser’s commitment to protecting user data and preventing unauthorised access sets it apart in the competitive web browser market.