Key Privacy Concerns Identified
1. Scale of Data Collection
- AI systems require massive amounts of data, making existing privacy issues worse.
- Data collection is more intransparent than ever before
- Nearly impossible for individuals to escape systematic digital surveillance
- Users have minimal control over what information is collected, how it’s used, or how to correct/remove it
2. Misuse of Personal Information
- Generative AI may memorise personal data and relational information about family/friends.
- Enables more sophisticated forms of spear-phishing, identity theft, and fraud
- Voice cloning technology is being used for extortion and impersonation.
3. Data Repurposing Without Consent
- Information shared for one purpose (like resumes or photos) is repurposed for AI training.
- Often happens without the knowledge or consent of individuals
- Can have direct civil rights implications
4. Algorithmic Bias and Discrimination
- AI systems trained on biased data reproduce and amplify those biases
- Examples include:
- Employment screening tools discriminate against women
- Facial recognition systems misidentify Black men, leading to false arrests
5. Lack of Control Over Training Data
- Personal information scraped from the internet may be included in AI training datasets
- Currently dependent on AI companies voluntarily removing personal information
- No guarantee against private information being revealed in AI outputs
Proposed Solutions
1. Shift from Opt-Out to Opt-In Data Sharing
- Make data collection require affirmative consent rather than being the default
- Examples of successful implementation:
- Apple’s App Tracking Transparency (80-90% of users choose to opt out)
- Global Privacy Control browser signals
- Proposed legislative changes that would require browsers to respect opt-out signals
2. Supply Chain Approach to Data Privacy
- Address privacy concerns across the entire AI data pipeline:
- Input side: Control what personal data can be used for training
- Output side: Prevent AI systems from revealing personal information
- Current regulatory approaches often miss this comprehensive view
- Even the EU’s AI Act only addresses data in the context of high-risk systems
3. Data Minimization and Purpose Limitation
- Companies should only collect data they genuinely need
- Data should only be used for clearly defined purposes
- Challenge: Regulators must determine what constitutes “too much data” for a given purpose
- Particularly difficult with tech giants that offer numerous services
4. Collective Solutions Instead of Individual Rights
- The individual rights approach puts too much burden on consumers
- Most people don’t know their rights or have time to exercise them
- Need for “data intermediaries” that negotiate data rights at scale
- Possible forms: data steward, trust, cooperative, collaborative, or commons
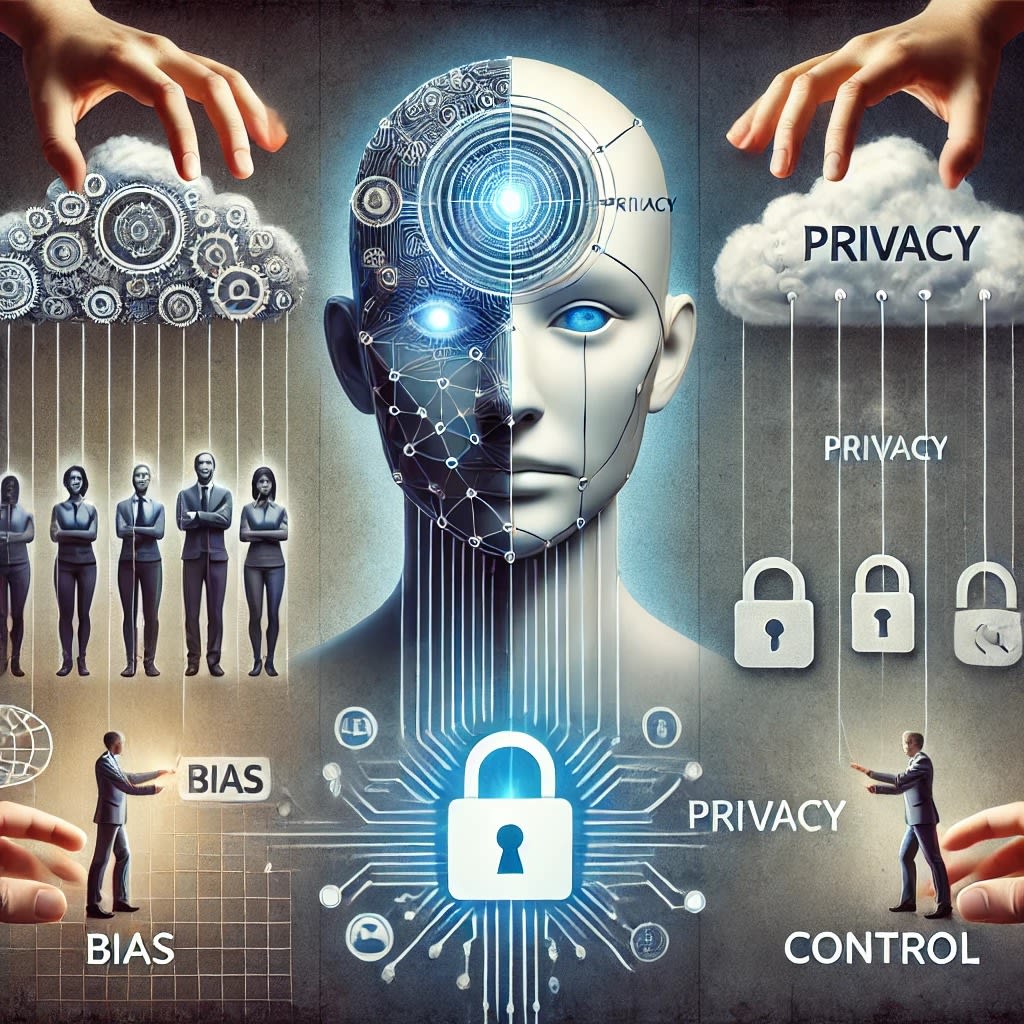
5. Stronger Regulatory Frameworks
- Need for regulatory systems that can require deletion of misused data
- Default rules and practices around data collection can be changed
- Current regulatory discussions often neglect the data aspects of AI
Implementation Challenges
- Defining appropriate boundaries for data collection in complex tech ecosystems
- Enforcing opt-in consent without disrupting user experience
- Creating effective data intermediaries for consumer contexts
- Balancing innovation with privacy protection
- Ensuring regulatory oversight keeps pace with rapidly evolving AI technologies
The core message from the article is that while AI exacerbates existing privacy concerns, there are viable solutions that could help protect personal information. Still, they require rethinking our approach to data privacy at both the individual and collective levels.
Privacy Concerns in the AI Era and Proposed Solutions: Singapore Context
Singapore’s AI and Data Privacy Landscape
Singapore has established itself as a regional leader in AI development and regulation, creating a framework that attempts to balance innovation with appropriate safeguards. Here’s how the privacy concerns and solutions identified in the article apply to Singapore’s specific context:
Privacy Concerns in Singapore’s AI Environment
1. Scale of Data Collection
- Singapore’s Smart Nation initiatives generate vast amounts of data across transportation, healthcare, and public services
- The city-state’s high digital connectivity means citizens leave substantial digital footprints.
- Government initiatives like TraceTogether during COVID-19 raised questions about data collection boundaries.
2. Data Protection Framework
- Singapore relies primarily on the Personal Data Protection Act (PDPA), last significantly amended in 2020
- PDPA operates on a consent-based model but includes numerous exceptions
- The Act may not fully address AI-specific concerns like algorithmic transparency and inference-based privacy violations
3. Public-Private Data Sharing
- Singapore actively encourages data sharing between government agencies and with private companies
- This creates unique privacy challenges not fully addressed in the original article
- GovTech and other agencies develop AI applications using combined datasets
4. Cross-Border Data Flows
- As a global business hub, Singapore handles significant international data transfers
- AI systems developed or deployed in Singapore may process data across jurisdictions
- Creates complexity in determining applicable privacy standards
Applying Proposed Solutions to Singapore
1. Opt-In Data Sharing in the Singapore Context
- Singapore could strengthen the consent provisions in the PDPA
- The “legitimate interests” exception could be narrowed for AI applications
- The PDIC (Personal Data Protection Commission) could require more explicit consent mechanisms for AI systems
2. Supply Chain Approach
- Singapore is well-positioned to implement a supply chain approach given its comprehensive governance systems
- The Model AI Governance Framework could be expanded to include full data lifecycle considerations
- Singapore’s AI Verify testing framework could incorporate privacy assessments across the AI data supply chain
3. Data Minimization
- Singapore’s public sector could lead by example through stricter data minimization practices
- Financial services (a key sector in Singapore) would benefit from clearer guidelines on necessary data for AI applications
- The concept of “data portability” in the PDPA could be strengthened
4. Collective Solutions
- Singapore’s community-oriented governance model aligns well with collective data rights approaches
- Could establish data trusts or cooperatives managed through existing community structures
- The Singapore Digital Trust Centre could potentially serve as a platform for collective data rights management
5. Regulatory Enhancements
- IMDA’s AI Governance Framework could be evolved from voluntary to mandatory standards
- Singapore’s sectoral regulators (MAS for finance, MOH for healthcare) could develop AI-specific privacy requirements
- The newly established AI Verify Foundation could incorporate stronger privacy evaluation metrics
Singapore-Specific Opportunities
- Trusted Data Sharing Framework: Building on Singapore’s existing data sharing frameworks to create privacy-preserving AI development environments
- AI Sandbox Environments: Expanding regulatory sandboxes to test privacy-protective AI systems before deployment
- Public Education: Leveraging Singapore’s strong educational infrastructure to improve AI literacy and privacy awareness
- International Leadership: Positioning Singapore as a model for balanced AI regulation that protects privacy while enabling innovation
- AI Ethics Committees: Expanding the role of ethics committees to include privacy impact assessments for both public and private AI deployments
Implementation Considerations for Singapore
- Cultural attitudes toward government and institutional trust may affect acceptance of privacy solutions
- Singapore’s size and governance structure enable potentially faster implementation of comprehensive solutions
- Need to balance Singapore’s AI ambitions with appropriate privacy safeguards
- Economic considerations as a technology hub and financial center require pragmatic approaches
Singapore has the potential to implement many of the solutions proposed in the original article while adapting them to its unique governance model, technological infrastructure, and strategic priorities as an AI leader in Southeast Asia.
Comprehensive Analysis of Privacy Issues and Protection Methods in the AI Era
Privacy Issues in the AI Era
1. Unprecedented Scale of Data Collection
- AI systems require massive training datasets, intensifying data collection
- Individuals face difficulty tracking what personal information is collected
- Continuous data gathering creates comprehensive digital profiles of users
- Behavioral data is collected across devices and contexts without clear boundaries
2. Lack of Transparency and Control
- AI systems often operate as “black boxes” with unclear data processing practices
- Difficulty exercising rights to access, correct, or delete personal information
- Limited understanding of how AI systems use collected data to make inferences
- Challenges in identifying when personal data is included in training datasets
3. Data Repurposing Without Consent
- Information shared for specific purposes being used for AI training without notice
- Historical data collected under different privacy expectations now used for AI
- Creative works and personal expressions scraped without creator permission
- Difficulty enforcing purpose limitation across complex data ecosystems
4. Advanced Privacy Threats
- Identity Inference: AI connecting disparate data points to reveal identities
- Re-identification: Anonymized data becoming identifiable through AI analysis
- Memorization: Large language models potentially exposing training data verbatim
- Voice/Image Synthesis: Creating convincing deepfakes for impersonation
5. Algorithmic Discrimination and Bias
- Models trained on biased data reproducing systemic discrimination
- Privacy harms disproportionately affecting marginalized communities
- Algorithmic profiling leading to differential treatment and opportunities
- Loss of privacy enabling various forms of social and economic discrimination
6. Commercial Surveillance Ecosystems
- Advertising technology creating extensive profiling capabilities
- Data brokers aggregating and selling personal information across contexts
- AI enabling more precise targeting and prediction of consumer behavior
- Economic incentives favoring maximum data collection and retention
7. Surveillance and Power Asymmetries
- AI amplifying surveillance capabilities of governments and corporations
- Facial recognition and biometric tracking in public spaces
- Predictive policing and risk assessment systems raising privacy concerns
- Employee monitoring through AI-powered productivity tools
Protection Methods and Solutions
1. Legal and Regulatory Frameworks
Comprehensive Privacy Legislation
- Data protection laws with AI-specific provisions
- Rights of access, deletion, correction, and data portability
- Limitations on automated decision-making
- Mandatory privacy impact assessments for high-risk AI systems
Sector-Specific Regulations
- Healthcare privacy protections for medical AI applications
- Financial data protection for AI-powered financial services
- Special protections for children’s data in AI systems
- Employment data protection for workplace AI
2. Technical Protection Measures
Privacy-Preserving Machine Learning
- Federated Learning: Training models across devices without centralizing data
- Differential Privacy: Adding noise to datasets to protect individual records
- Homomorphic Encryption: Computing on encrypted data without decryption
- Synthetic Data: Using artificially generated data for training purposes
User-Controlled Privacy Tools
- Personal privacy management dashboards
- Browser extensions blocking trackers and data collection
- Privacy-focused devices and operating systems
- Encrypted communications platforms
3. Organizational Practices
Privacy by Design
- Integrating privacy considerations into AI development lifecycle
- Data minimization and purpose limitation principles
- De-identification techniques when processing personal data
- Retention limits and automatic deletion policies
Transparency Mechanisms
- Clear privacy notices about AI data processing
- Algorithmic impact assessments
- Explainable AI approaches
- Data provenance tracking and documentation
4. Consent and Control Paradigms
Meaningful Consent Models
- Contextual consent based on actual use cases
- Layered consent notices with varying levels of detail
- Just-in-time consent at the moment data is needed
- Opt-in requirements for sensitive data processing
Revocable Permissions
- Right to withdraw consent for specific uses
- Data deletion upon request
- User-controlled data permissions
- Consent expiration and renewal requirements
5. Collective Approaches
Data Intermediaries
- Data trusts managing personal information on behalf of groups
- Data cooperatives giving collective bargaining power
- Privacy advocates representing consumer interests
- Industry consortiums establishing privacy standards
Accountability Mechanisms
- Independent auditing of AI systems
- Certification programs for privacy-protective AI
- Civil society oversight and watchdog organizations
- Whistleblower protections for reporting privacy violations
6. Education and Awareness
Digital Literacy Programs
- Public education about AI and data privacy risks
- Tools for detecting privacy vulnerabilities
- Resources for exercising privacy rights
- Professional training on privacy-preserving AI development
Privacy-Focused Design Standards
- User interface patterns promoting privacy awareness
- Privacy indicators in consumer products
- Standardized privacy labels and certifications
- Privacy-enhancing competition as market differentiator
Implementation Challenges
1. Economic and Innovation Tensions
- Balancing privacy protection with beneficial AI innovation
- Cost of implementing privacy safeguards in AI systems
- Competitive disadvantages for privacy-focused companies
- Market incentives favoring data maximization
2. Technical Complexity
- Difficulty applying traditional privacy concepts to AI systems
- Challenges in detecting privacy violations in complex algorithms
- Trade-offs between model accuracy and privacy protection
- Implementation difficulties for privacy-preserving techniques
3. Global Governance Gaps
- Inconsistent privacy regulations across jurisdictions
- Cross-border data flows challenging enforcement
- Varying cultural and social norms around privacy
- Difficulty establishing global privacy standards for AI
4. Practical Implementation
- Legacy systems and infrastructure limitations
- Resource constraints for privacy compliance
- Shortage of expertise in privacy-preserving AI techniques
- Challenges in measuring privacy protection effectiveness
This analysis provides a comprehensive overview of the privacy challenges in the AI era and the range of potential protection methods, from legal frameworks to technical solutions and organizational practices. Effective privacy protection will likely require a combination of approaches tailored to specific contexts, alongside ongoing evaluation and adaptation as AI technologies continue to evolve.
Innovative Privacy Solutions for AI Privacy Issues
Advanced Technical Solutions
1. Zero-Knowledge Proofs for AI Systems
- Allow AI systems to verify information without accessing the underlying data
- Enable authentication and verification while preserving complete privacy
- Particularly useful for financial AI applications and identity verification
- Can help prove compliance with regulations without exposing sensitive data
2. Personal AI Privacy Guardians
- AI systems that work on behalf of individuals to protect their privacy
- Automated negotiation of privacy terms with other AI systems
- Continuous monitoring for privacy violations across platforms
- Preemptive detection and blocking of data collection that violates user preferences
3. Distributed AI Governance
- Blockchain-based governance systems for AI data usage
- Immutable audit trails of how personal data is accessed and used
- Smart contracts that automatically enforce privacy preferences
- Decentralized identity systems giving users control over their digital identities
4. Privacy-Preserving Data Markets
- Secure environments where data value can be extracted without transferring actual data
- Compensation models for individuals whose data contributes to AI training
- Data licensing frameworks with granular permissions and revocation rights
- Privacy-preserving APIs that return insights rather than raw data
5. Attribute-Based Encryption for Data Access
- Cryptographic enforcement of complex privacy policies
- Access to data determined by specific attributes of the requesting entity
- Fine-grained control over which AI systems can use which pieces of personal data
- Cryptographic guarantees rather than policy-based promises
Policy and Regulatory Innovations
1. Dynamic Consent Frameworks
- Evolving consent based on changing context and use cases
- AI systems that adapt privacy protections based on sensitivity of processing
- Regular consent “check-ins” to ensure ongoing alignment with user preferences
- Graduated consent levels for different types of AI processing
2. Algorithmic Impact Bonds
- Financial instruments that tie company profit to responsible privacy practices
- Investment vehicles that pay returns when privacy goals are met
- Creates market incentives for privacy-preserving AI development
- Similar to social impact bonds but focused on privacy outcomes
3. Privacy Commons Licensing
- Standardized licensing framework for personal data similar to Creative Commons
- Clear visual indicators of permitted AI uses of personal information
- Machine-readable privacy terms that AI systems can automatically respect
- Enables individuals to share data while maintaining control
4. Sectoral Privacy Codes of Practice
- Industry-specific privacy standards with enforcement mechanisms
- Certification requirements for AI systems in sensitive domains
- Peer governance models within industry verticals
- Specialized frameworks for healthcare, finance, education, etc.
5. Privacy Rights Advocacy Organizations
- Funded entities with legal standing to enforce privacy rights
- Collective representation in regulatory proceedings
- Class action capabilities for systematic privacy violations
- Technical expertise to evaluate AI systems for privacy compliance
Organizational and Cultural Approaches
1. Privacy Ethics Committees
- Diverse stakeholder oversight of AI privacy practices
- Regular review of emerging privacy risks and mitigation strategies
- Public reporting on privacy impacts and protection measures
- Integration with broader AI ethics governance
2. Privacy-Centered Design Competitions
- Rewards for innovative privacy-preserving AI architectures
- Open challenges to solve specific privacy problems
- Public recognition for privacy leadership
- Creating a culture of privacy innovation
3. Privacy Impact Scoring
- Standardized metrics for evaluating AI privacy impacts
- Public disclosure of privacy scores for consumer-facing AI systems
- Comparative benchmarking across similar products
- Integration with procurement decisions
4. Privacy Personnel Requirements
- Mandatory privacy expertise on AI development teams
- Certification programs for AI privacy professionals
- Privacy advocates embedded within product teams
- Executive accountability for privacy outcomes
5. Community Data Trusts
- Local governance of data resources by affected communities
- Democratic decision-making about acceptable AI uses
- Protection of collective privacy interests beyond individual consent
- Particularly valuable for marginalized communities
Educational and Consumer Empowerment
1. AI Privacy Literacy Programs
- Curriculum development for schools and universities
- Public awareness campaigns about AI data collection
- Interactive tools demonstrating privacy risks and protections
- Targeted education for vulnerable populations
2. Privacy Nutrition Labels
- Standardized disclosure of AI data practices
- Simple visual indicators of privacy protections
- Comparable metrics across products and services
- Third-party verification of claims
3. Privacy Simulation Tools
- Software that demonstrates potential privacy impacts of data sharing
- Visualization of inference capabilities from seemingly innocuous data
- “What-if” scenarios showing potential privacy harms
- Training environments for privacy-protective behaviors
4. Privacy Rights Chatbots
- AI assistants specialized in explaining and enforcing privacy rights
- Automated filing of access and deletion requests
- Help navigating complex privacy settings
- Translation of legal privacy terms into plain language
5. Cross-Platform Privacy Management
- Centralized dashboards to control privacy across services
- Data flow visualization showing connections between services
- One-click privacy preferences applied across ecosystems
- Automated detection of policy changes affecting privacy
Implementation Strategy
Effective privacy protection requires a layered approach combining multiple solutions:
- Immediate Term: Focus on transparency, consent improvements, and education
- Medium Term: Implement technical safeguards and accountability mechanisms
- Long Term: Develop advanced infrastructure for privacy-preserving AI ecosystems
The most promising approach involves combining technical safeguards with appropriate governance frameworks and empowering individuals through both tools and knowledge. This creates a comprehensive privacy protection system that addresses the unique challenges of AI while enabling beneficial innovation to continue.Retry
Changing Expectations
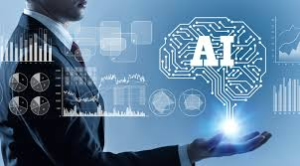
In 2018, 76% of senior banking leaders believed AI would be critical for market differentiation.
Early predictions were dramatic, with some suggesting AI could replace half of bank staff.
The actual impact is turning out to be more nuanced and less disruptive than initially feared.
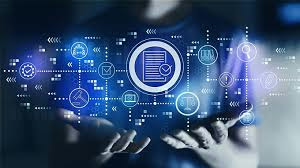
Primary AI Applications in Banking
Customer service improvement
Personalized recommendations based on payment history
Chatbots for handling common customer queries
Seamless handover to human representatives when needed
Optimizing cash flow management
Helping less tech-savvy customers navigate digital banking services
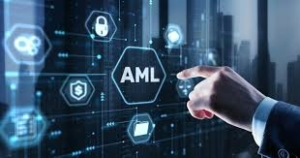
Key Challenges in AI Adoption
Integrating AI with existing legacy banking systems
Accessing and leveraging existing customer data
Avoiding creation of new technological silos
Ensuring smooth, frictionless customer experiences
Innovative Approach Example
The article highlights Auriga’s WinWebServer (WWS) AI module as an innovative solution that:
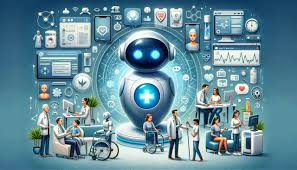
Seamlessly integrates with legacy systems.
Enables enhanced customer experiences
Speeds up strategic decision-making
Helps optimize cash management across bank branches and ATMs
Subtle but Significant Impact
Rather than replacing human workers, AI is primarily being used to augment and improve banking services, making them more personalized, efficient, and accessible.
The article suggests that AI in banking is less about disruption and more about incremental, strategic improvements to existing banking processes and customer interactions.RetryCanalyse ai as transformation not disruptionEditI’ll provide an analysis of AI as a transformative force in banking, expanding on the themes from the article and drawing insights about how AI is fundamentally reshaping the banking landscape.
AI: A Transformative Force in Banking
Conceptual Shift: From Disruption to Transformation
AI represents a fundamental transformation of banking, not merely a disruptive technology. This transformation is characterized by:
1. Intelligent Personalization
- Beyond traditional customer segmentation
- Real-time, individual-level personalization of financial services
- Predictive understanding of customer needs before they arise
- Customized financial advice and product recommendations tailored to individual financial behaviors and life stages
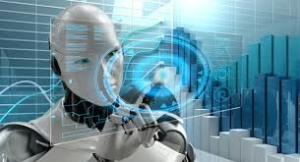
2. Operational Intelligence
- Reimagining banking processes through cognitive automation
- Moving from rule-based systems to adaptive learning systems
- Continuous optimization of internal operations
- Predictive maintenance of financial infrastructure
- Dynamic risk management and compliance monitoring
3. Customer Experience Reimagined
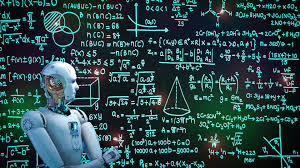
- Shift from transactional interactions to contextual, anticipatory engagement
- 24/7 intelligent support systems
- Seamless omnichannel experiences
- Proactive financial guidance
- Accessibility-driven design that serves diverse customer capabilities
4. Strategic Decision Making
- AI as a strategic partner in executive decision-making
- Advanced scenario modeling
- Real-time market trend analysis
- Enhanced predictive capabilities for investment and risk strategies
- Democratization of sophisticated financial insights
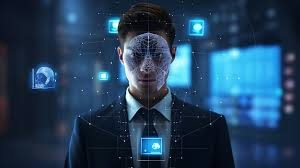
5. Ethical and Inclusive Innovation
- Using AI to address historical banking inequities
- Creating more transparent and fair financial assessment models
- Developing inclusive financial products
- Reducing human bias in financial decision-making
- Supporting underserved financial populations through intelligent design
Transformation Characteristics
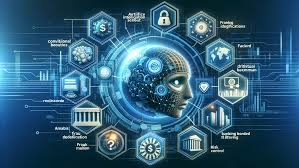
- Evolutionary, Not Revolutionary: Gradual integration that builds upon existing systems
- Augmentative Intelligence: Enhancing human capabilities, not replacing them
- Adaptive Learning: Continuous improvement through sophisticated machine learning
- Holistic Approach: Addressing multiple banking dimensions simultaneously
Future Outlook
The true power of AI in banking lies not in replacing human workers but in creating a symbiotic ecosystem where technological intelligence and human expertise collaborate to deliver unprecedented value.
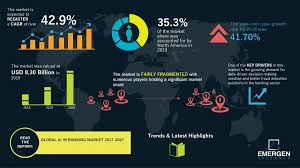
Maxthon
Maxthon has set out on an ambitious journey aimed at significantly bolstering the security of web applications, fueled by a resolute commitment to safeguarding users and their confidential data. At the heart of this initiative lies a collection of sophisticated encryption protocols, which act as a robust barrier for the information exchanged between individuals and various online services. Every interaction—be it the sharing of passwords or personal information—is protected within these encrypted channels, effectively preventing unauthorised access attempts from intruders.
Maxthon private browser for online privacyThis meticulous emphasis on encryption marks merely the initial phase of Maxthon’s extensive security framework. Acknowledging that cyber threats are constantly evolving, Maxthon adopts a forward-thinking approach to user protection. The browser is engineered to adapt to emerging challenges, incorporating regular updates that promptly address any vulnerabilities that may surface. Users are strongly encouraged to activate automatic updates as part of their cybersecurity regimen, ensuring they can seamlessly take advantage of the latest fixes without any hassle.
In today’s rapidly changing digital environment, Maxthon’s unwavering commitment to ongoing security enhancement signifies not only its responsibility toward users but also its firm dedication to nurturing trust in online engagements. With each new update rolled out, users can navigate the web with peace of mind, assured that their information is continuously safeguarded against ever-emerging threats lurking in cyberspace.
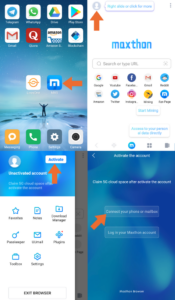