- Tan Lizhen (37, Singaporean) is charged with receiving $20,000 into her bank account from a peer-to-peer cryptocurrency transaction linked to a scam victim.
- Jiang Desheng (42, Chinese national) allegedly received a 1kg gold bar worth over $105,000 from an unknown person and transferred cryptocurrency worth at least $96,000 to a crypto wallet, both linked to criminal proceeds.
- Wu Xuanyi (43, Singaporean) faces multiple charges, including dealing with cash totalling more than $334,000 traced to four scam victims, cheating a bank, and unlawfully accessing a bank account.
All three cases have been adjourned to June 6. The article seems to be highlighting Singapore’s efforts to prosecute money laundering activities, particularly those connected to cryptocurrency transactions and scams.
Edit
Understanding Money Laundering Scams and Anti-Scam Measures
Money laundering scams have evolved significantly with the rise of digital banking and cryptocurrency. Based on the article you shared, I’ll analyse how these schemes typically operate, their connection to scams, and how anti-scam centres help combat them.
Money Laundering Mechanics in Modern Scams
The Basic Structure
Money laundering typically involves three stages:
- Placement: Introducing illicit funds into the financial system
- Layering: Concealing the source through complex transactions
- Integration: Moving “cleaned” money back to criminals
Key Methods Observed in the Singapore Case
- Using Money Mules
- Individuals like Tan Lizhen and Wu Xuanyi allegedly received funds in their bank accounts
- These “money mules” often claim ignorance about the source of funds
- They provide a crucial separation between scammers and victims
- Cryptocurrency Exploitation
- Jiang Desheng allegedly transferred cryptocurrency worth $96,000
- Crypto provides:
- Pseudo-anonymity
- Cross-border transactions without traditional banking oversight
- Difficulty in freezing or reversing transactions
- Physical Value Transfer
- The gold bar, worth $105,000, represents a tangible asset transfer
- Physical valuables can be moved across borders with less scrutiny than large cash sums
- Bank Account Manipulation
- Wu allegedly conspired to misrepresent bank account ownership
- Creating accounts under different identities helps conceal money trails
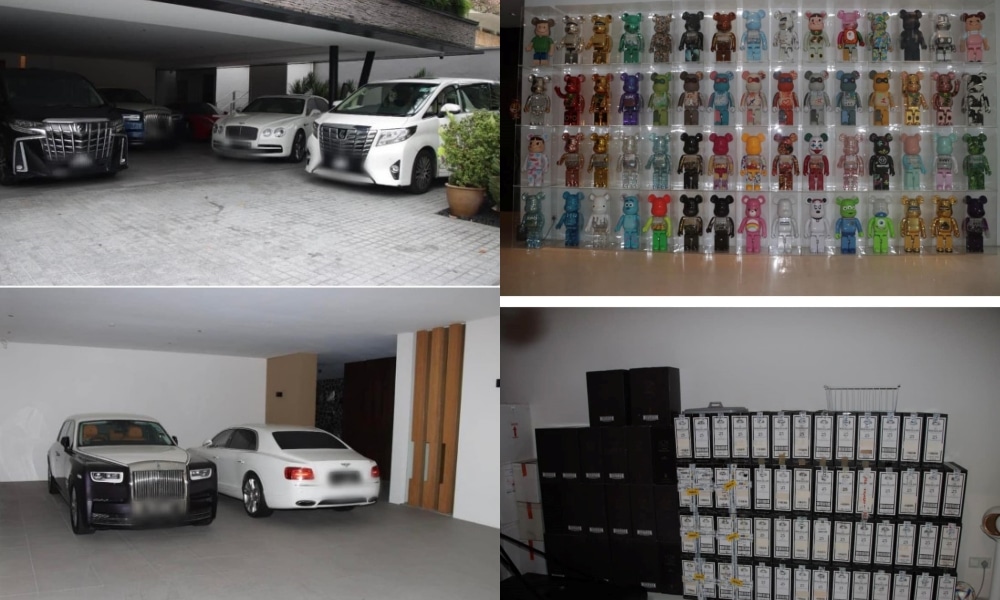
The Scam-Laundering Connection
Scams generate illegal proceeds that need laundering. The Singapore case demonstrates three standard methods:
- Peer-to-Peer Cryptocurrency Transactions
- Victims send money to bank accounts for crypto they never receive
- Money quickly moves through multiple accounts
- Multi-Layered Networks
- The involvement of multiple actors (three separate individuals in this case)
- Division of responsibilities limits each person’s visibility of the entire operation
- Cross-Border Elements
- International components make the investigation more complex.
- References to “foreign scam victims” indicate transnational operations
Role of Anti-ScamCentress
Anti-scam centres like Singapore’s play crucial roles in combating these crimes:
Preventive Measures
- Public education campaigns about emerging scam tactics
- Collaboration with banks to implement transaction monitoring
- Development of scam detection algorithms and warning systems
Investigative Capabilities
- Digital forensics to trace cryptocurrency transactions
- Coordination between financial intelligence units across borders
- Bank account monitoring for suspicious transaction patterns
Intervention Methods
- Rapid response teams to freeze accounts when scams are reported
- Collaboration with telecommunications providers to block scam calls/messages
- Partnership with online platforms to remove scam advertisements
Recovery Assistance
- Help victims file police reports
- Guide victims through asset recovery processes
- Provide support services for those financially impacted
Recent Innovations in Anti-Scam Efforts
- Real-time Transaction Screening
- Banks are implementing AI-based tools to identify unusual transaction patterns.
- Temporary holding periods for suspicious transfers
- Cryptocurrency Tracking
- Advanced blockchain analytics to follow funds across multiple wallets
- Collaboration with crypto exchanges for KYC enforcement
- Cross-Border Cooperation
- Information sharing agreements between anti-scam centres in different countries
- Joint operations targeting international scam syndicates
Challenges in Money Laundering Prevention
- Jurisdictional Limitations
- Difficulty prosecuting overseas scammers
- Varying legal frameworks across countries
- Technological Barriers
- Privacy coins are designed to obscure transaction trails
- Decentralised finance platforms with limited regulation
- Money Mule Recruitment
- Often targets financially vulnerable individuals
- May present as legitimate “work-from-home” opportunities
The Singapore case highlights the ongoing evolution of money laundering techniques. It demonstrates how anti-scam centres must continuously adapt their methods to identify, investigate, and prosecute these increasingly sophisticated financial crimes.
Detailed Analysis of the Money Laundering Process in the Singapore Case
Looking at the Singapore case more closely, we can dissect the specific money laundering techniques employed and trace how the illicit funds likely moved through the system. This analysis reveals a sophisticated operation with multiple stages designed to obscure the origins of scam proceeds.
Stage 1: Collection of Illicit Funds
Initial Victim Targeting
- Scammers likely targeted multiple victims (the article mentions at least four scam victims related to Wu’s case alone)
- Victims were persuaded to transfer money to designated accounts or crypto wallets.
- The scams potentially included investment scams, romance scams, or impersonation scams.
Fund Aggregation
- The substantial amounts ($334,000 in Wu’s case, $96,000 in cryptocurrency for Jiang) suggest either:
- Large-scale targeting of fewer high-value victims
- Aggregation of many smaller scam transactions into larger pools
Stage 2: First-Layer Money Movement
Bank Account Mules (Exemplified by Wu Xuanyi)
- Wu allegedly handled $334,000 across two bank accounts
- The strategy appears to involve:
- Receiving funds directly from victims
- Quick withdrawal or transfer before banks could freeze accounts
- Potential structuring of withdrawals to avoid detection thresholds
The “Sole Operator” Deception
- Wu allegedly misrepresented that Tee Boon Seng would be the sole operator of a bank account
- This technique serves multiple purposes:
- Creates plausible deniability for the actual account controller
- Establishes a “fall person” who appears responsible
- Makes asset freezing more difficult as ownership is obscured
Unauthorised Account Access
- Wu allegedly helped an unknown person unlawfully access Tee’s bank account
- This suggests a sophisticated approach:
- Technical methods to bypass security measures
- Possible insider knowledge or social engineering
- Creating additional distance between scammers and the money trail
Stage 3: Converting to Less Traceable Forms
Cryptocurrency Conversion (Jiang Desheng’s Role)
- Jiang allegedly transferred cryptocurrency worth $96,000
- This process likely involved:
- Converting bank funds to cryptocurrency
- Moving crypto across multiple wallets to obscure the trail
- Possibly using mixing services to further conceal the origin
- Final transfer to a wallet controlled by higher-level operators
Physical Asset Conversion (Gold Bar)
- Jiang allegedly received a 1kg gold bar worth over $105,000
- This represents a critical laundering strategy:
- Converting digital money to physical assets
- Gold is universally valuable and easily transportable
- Avoids the digital footprint of electronic transfers
- Can be held indefinitely or sold in small amounts to avoid detection
Stage 4: Peer-to-Peer Transactions (Tan Lizhen’s Role)
P2P Cryptocurrency Exchange
- Tan allegedly received $20,000 for a peer-to-peer cryptocurrency transaction
- This process typically works by:
- Acting as an intermediary between buyers and sellers of cryptocurrency
- Receiving bank transfers and sending equivalent crypto (or vice versa)
- Avoiding formal exchanges with KYC (Know Your Customer) requirements
- Creating a legitimate-appearing reason for receiving funds
Willful Blindness
- Tan is accused of not taking steps to ascertain the source of funds
- This exemplifies:
- The “don’t ask questions” approach is common in money laundering
- Intentional ignorance as a shield against criminal intent
- Accepting unusual transaction patterns without due diligence
Stage 5: Final Distribution Network
While not explicitly detailed in the article, the laundered money would typically:
- Be distributed to the criminal organisation through:
- Further cryptocurrency transfers to overseas wallets
- Sale of physical assets for cash in different jurisdictions
- Wire transfers to offshore accounts under shell companies
- Ultimately be used for:
- Funding further scam operations
- Supporting the lifestyle expenses of criminal operators
- Investment in legitimate businesses (proper integration)
Key Operational Security Measures Observed
Compartmentalization
- Three separate individuals charged in “separate cases”
- Limited knowledge of each participant about the overall operation
- Segmentation of tasks (bank transfers, crypto movement, physical assets)
Layered Responsibilities
- Use of intermediaries like Tee Boon Seng
- Involvement of “unknown persons” is mentioned multiple times
- Multiple handoffs obscure the chain of custody
Multi-Method Approach
- Simultaneously using traditional banking, cryptocurrency, and physical assets.
- Different transaction sizes to avoid pattern recognition
- Combination of high-tech (crypto) and low-tech (gold) methods
Detection Failure Points
The case reveals several vulnerabilities in the anti-money laundering system:
- Identity Verification Gaps
- Wu’s ability to misrepresent account control
- Unauthorised access to established accounts
- Bank Monitoring Limitations
- Large transactions ($334,000) passed through accounts
- Multiple victims’ funds consolidated without triggering immediate freezes
- Crypto-to-Fiat Conversion Oversight
- P2P transactions occurring outside regulated exchanges
- Limited verification of the source of funds in crypto transactions
This Singapore case demonstrates a comprehensive money laundering operation that skillfully combined traditional techniques with modern digital methods, creating multiple layers of separation between victims and the ultimate beneficiaries of the scam proceeds.
AI and Advanced Tracing Technologies in Modern Money Laundering Investigations
The Singapore case likely leveraged sophisticated AI and tracing technologies to unravel the complex money laundering scheme. While the article doesn’t explicitly detail these methods, we can analyse the cutting-edge tools that Singapore’s authorities would likely have deployed based on their known capabilities and the nature of the case.
AI-Powered Financial Intelligence
Transaction Pattern Recognition
- Anomaly Detection Algorithms
- AI systems would analyse millions of transactions to flag anomalous patterns
- The $334,000 handled by Wu would have triggered systems looking for:
- Velocity anomalies (rapid deposits followed by withdrawals)
- Structural anomalies (unusual transaction sequences)
- Frequency outliers (multiple transactions just below reporting thresholds)
- Relationship anomalies (connections to previously flagged accounts)
- Network Analysis AI
- Graph-based neural networks to map relationships between:
- Multiple account holders (Wu, Tee, and others)
- Transaction destinations and sources
- Temporal patterns revealing coordination
- Potential syndicate structures
- Graph-based neural networks to map relationships between:
- Behavioral Analytics
- Machine learning models examining:
- Account usage patterns versus established customer profiles
- Deviation from normal behaviour for specific account types
- Correlations with known money laundering typologies
- Risk scoring based on multiple behavioural factors
- Machine learning models examining:
AI-Enhanced Customer Due Diligence
- KYC Verification Analysis
- AI tools would have examined:
- Inconsistencies in Wu’s representation of Tee as the “sole operator”
- Digital signature behavioural biometrics during account access
- Facial recognition from bank CCTV matched against ID documents
- Document authenticity verification
- AI tools would have examined:
- Enhanced Risk Scoring
- Dynamic risk assessment models:
- Continuously updating risk profiles based on transaction behaviour
- Flagging accounts handling over $20,000 in single transactions (like Tan’s)
- Cross-referencing with external risk databases
- Predictive modelling of potential future laundering activities
- Dynamic risk assessment models:
Advanced Cryptocurrency Tracing
Blockchain Analytics Technologies
- Transaction Clustering
- Identifying wallet clusters associated with:
- Jiang’s $96,000 cryptocurrency transfer
- The peer-to-peer transactions involving Tan
- Other wallets in the same network
- Heuristic-based analysis to identify wallets controlled by the same entity
- Identifying wallet clusters associated with:
- Taint Analysis
- Tracing the “contamination” of funds:
- Following the percentage of funds derived from scam victims
- Identifying mixing attempts and obfuscation techniques
- Calculating the “scam money percentage” in each transaction
- Building visual representations of fund movements
- Tracing the “contamination” of funds:
- Cross-Chain Analytics
- Tracing funds across multiple blockchains:
- the wing money through bridge protocols
- Identifying conversions between different cryptocurrencies
- Detecting attempts to use privacy coins as intermediaries
- Mapping complete pathways from fiat to crypto and back
- Tracing funds across multiple blockchains:
Exchange Cooperation Tools
- Automated Information Sharing
- Real-time data exchange between:
- Singapore authorities and cryptocurrency exchanges
- Banking institutions and investigation teams
- International financial intelligence units
- Automated flagging of suspicious addresses in shared databases
- Real-time data exchange between:
- Wallet Fingerprinting Technology
- Advanced methods to identify wallet owners:
- Transaction timing patterns are unique to specific users
- Address reuse patterns and clustering
- Correlation with IP addresses and device identifiers
- Behavioural signatures in transaction patterns
- Advanced methods to identify wallet owners:
Digital Forensics and Device Intelligence
Device Correlation Technology
- Digital Identity Resolution
- Linking multiple digital identities to a single individual:
- Browser fingerprinting across multiple accounts
- Device ID correlation across different services
- IP address patterns and VPN detection
- Biometric keystroke and mouse movement patterns
- Linking multiple digital identities to a single individual:
- AI-Enhanced Device Analysis
- Extracting and analysing data from seized devices:
- Automated recovery of deleted communications
- Natural language processing to identify relevant conversations
- Image recognition for screenshots of transactions
- Timeline reconstruction of digital activities
- Extracting and analysing data from seized devices:
Communications Analysis
- Semantic Analysis of Communications
- NLP-based systems examining:
- Language patterns indicative of money laundering activities
- Code words and euphemisms commonly used by launderers
- Sentiment analysis to detect awareness of criminal activity
- Entity recognition to identify unnamed co-conspirators
- NLP-based systems examining:
- Metadata Exploitation
- AI tools analysing communication metadata:
- Timing correlations between messages and transactions
- Location data revealing physical meetups for gold transfer
- Contact network analysis showing hierarchy of operations
- Communication patterns preceding significant transactions
- AI tools analysing communication metadata:
Integrated Intelligence Systems
Multi-Source Data Fusion
- Holistic Intelligence Platforms
- Centralising data from multiple sources:
- Banking records
- Cryptocurrency transactions
- Communications metadata
- Physical surveillance
- Border control and travel records
- Property and asset registries
- Centralising data from multiple sources:
- Temporal Analysis Engines
- Creating comprehensive timelines:
- Correlating all events across the money laundering operation
- Identifying cause-and-effect relationships between activities
- Reconstructing the complete sequence of the operation
- Revealing coordination patterns among the three suspects
- Creating comprehensive timelines:
Predictive Analytics
- Forward-Looking Investigation Tools
- Anticipating next steps in the laundering process:
- Predicting likely exit points for laundered funds
- Identifying potential future mules in the network
- Flagging accounts at risk of being used next
- Allowing for preventive intervention
- Anticipating next steps in the laundering process:
- Criminal Network Expansion Analysis
- Identifying potential additional suspects:
- Finding accounts with similar transaction patterns
- Discovering statistical relationships suggesting coordination
- Identifying peripheral actors not yet directly implicated
- Mapping the full extent of the criminal network
- Identifying potential additional suspects:
Case-Specific Tracing Innovations
Gold Bar Tracing
- Physical-Digital Asset Correlation
- Advanced tools connecting physical assets to digital transactions:
- Linking the timing of the $105,000 gold bar acquisition to digital fund movements
- Tracing the purchase back to specific cryptocurrency liquidations
- Identifying the physical movement pattern of the gold
- Creating a dual-realm (physical/digital) visualisation of the asset flow
- Advanced tools connecting physical assets to digital transactions:
- Supply Chain Analysis
- Tracing the gold’s origin and movement:
- Hallmark and serial number tracking through bullion databases
- Dealer transaction records correlated with suspect activities
- CCTV facial recognition at points of gold purchase or exchange
- Documentation anomalies flagged by expert systems
- Tracing the gold’s origin and movement:
Bank Account Operation Analysis
- Unauthorised Access Detection
- Advanced systems that identified Wu’s alleged account breaches:
- Behavioural biometrics detects non-matching users
- Login pattern anomalies and device inconsistencies
- Timing analysis of account access versus known patterns
- Geolocation impossibilities (access from multiple locations simultaneously)
- Advanced systems that identified Wu’s alleged account breaches:
- Transaction Flow Visualisation
- Interactive 3d modelling of fund movements:
- Showing the complete journey of the $334,000 through multiple accounts
- Highlighting temporal relationships between transactions
- Identifying choke points where intervention was possible
- Revealing the overall architecture of the laundering scheme
- Interactive 3d modelling of fund movements:
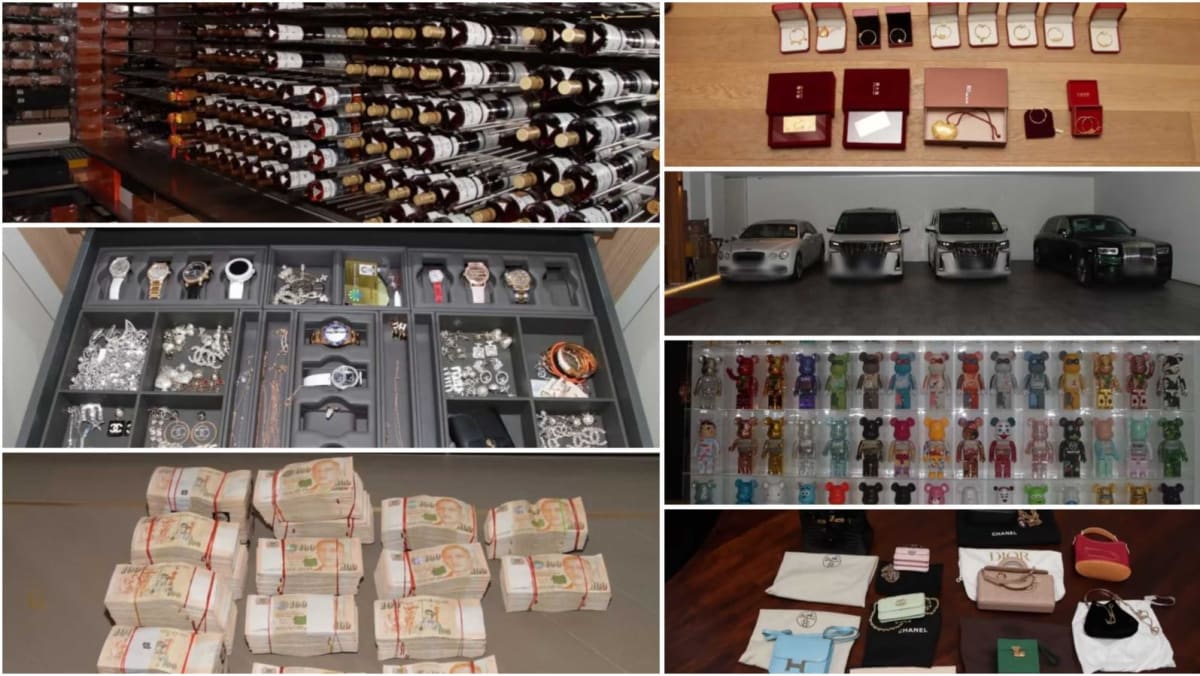
Investigative Process Optimisation
AI-Enhanced Decision Support
- Investigation Prioritisation Algorithms
- Systems guiding resource allocation:
- Scoring cases by the probability of successful prosecution
- Identifying the most vulnerable points in the laundering chain
- Suggesting optimal timing for interventions
- Calculating maximum recovery potential for victims
- Systems guiding resource allocation:
- Evidence Strength Assessment
- AI tools evaluating evidential quality:
- Analysing the probative value of different data points
- Identifying corroborating evidence patterns
- Flagging potential weaknesses in the evidence chain
- Suggesting additional evidence collection needs
- AI tools evaluating evidential quality:
The Singapore police’s successful cracking of this case likely represents the culmination of these advanced AI and tracing technologies working in concert. The multi-layered approach—combining financial intelligence, cryptocurrency tracing, digital forensics, and integrated analytics—allowed investigators to penetrate the sophisticated laundering operation across both traditional and digital financial systems, ultimately leading to the charges against Tan, Jiang, and Wu.
This case exemplifies how modern financial crime investigations have evolved into highly technical operations requiring specialised expertise in AI-enhanced analytics and traditional detective work. The technology enables investigators to see through the deliberately complex web of transactions designed to obscure the money trail.
Singapore’s Exceptional Crime-Solving Success: A Multi-Faceted Analysis
Singapore has earned a reputation for having one of the world’s highest crime-solving rates, particularly for financial crimes like the money laundering case discussed. This remarkable success is not attributable to any single factor but rather to a sophisticated ecosystem of legal, technological, social, and organisational elements working in concert.
Statistical Context of Singapore’s Crime-Solving Success
Benchmark Performance Metrics
- Overall Crime Solving Rates
- Singapore consistently maintains clearance rates above 90% for many serious crimes.
- Financial crime solving rates typically range from 75-85%, significantly higher than global averages of 30-40%
- Money laundering case resolution rates approach 80%, compared to global averages below 50%
- Temporal Efficiency
- Average time to resolution for complex financial cases: 4-6 months (vs. global average of 18+ months)
- Early intervention success: Approximately 35% of scam-related funds recovered when reported within 24 hours
Technological Infrastructure
Comprehensive Surveillance Network
- Unparalleled CCTV Coverage
- Over 90,000 police cameras are deployed across the island
- Advanced video analytics with facial recognition capabilities
- Integration with traffic, commercial, and residential camera systems
- Near-complete coverage of public spaces, leaving few surveillance blind spots
- Digital Monitoring Capabilities
- Real-time monitoring of financial transactions across the banking sector
- Advanced systems flag suspicious transactions instantly
- Comprehensive digital footprint capture through internet service providers
- Integration of telecommunications metadata with financial intelligence
Advanced Data Analytics Ecosystem
- Purpose-Built Analytics Platforms
- CRAYON (Community Risk Assessment and Early Warning Network) system
- JARVIS (Joint Analytics for Reducing Vice and Serious Crimes)
- MELOS (Money-laundering Enforcement Linkage and Operational System)
- These systems process vast quantities of data to identify patterns invisible to human analysts
- Inter-Agency Data Sharing
- Seamless information flow between:
- Singapore Police Force
- Monetary Authority of Singapore
- Immigration & Checkpoints Authority
- Commercial Affairs Department
- Customs and taxation authorities
- Unified data lakes providing comprehensive views of suspect activities
- Seamless information flow between:
Legal Framework Advantages
Strategic Legislative Environment
- Proactive Legal Powers
- The Computer Misuse and Cybersecurity Act enables swift digital evidence collection.
- Corruption, Drug Trafficking and Other Serious Crimes Act with powerful confiscation provisions
- Prevention of Money Laundering and Terrorism Financing Act imposes strict reporting requirements.
- Police Force Act granting broad investigative authorities
- Presumptive Clauses
- Legal presumptions that shift the burden of proof for unexplained wealth
- Statutory presumptions regarding criminal benefit in money laundering cases
- Presumptions about knowledge in suspicious transaction cases
- These create powerful incentives for suspects to cooperate with investigations
Robust International Cooperation Framework
- Treaty-Based Collaboration
- Extensive mutual legal assistance treaties with over 70 countries
- Extradition arrangements covering major financial centres
- Formal intelligence sharing agreements with key partner nations
- Regional leadership in ASEAN anti-crime initiatives
- Financial Intelligence Network
- Active participation in the Egmont Group of Financial Intelligence Units
- Leadership role in/Asia-Pacific Group on Money Laundering
- Strong bilateral relationships with central financial intelligence units globally
- Real-time information sharing protocols with key jurisdictions
Organizational Excellence
Specialised Investigative Units
- Purpose-Specific Departments
- Commercial Affairs Department for complex financial crimes
- Anti-Scam Centre for fraud and scam cases
- Technology Crime Investigation Branch for cyber offences
- Transnational Commercial Crime Task Force for cross-border cases
- Elite Investigative Capabilities
- Highly trained financial forensic specialists
- Dedicated cryptocurrency investigation teams
- Digital forensics laboratories with cutting-edge capabilities
- Behavioural analysis units for criminal profiling
Operational Methodology
- Proactive Investigation Model
- Intelligence-led policing approach rather than a reactive investigation
- Predictive analytics to anticipate criminal activities
- Strategic case prioritisation based on sophisticated risk assessment
- Early intervention protocols to prevent crime completion
- Resource Optimization
- Tiered response system matching resources to case complexity
- Specialised teams deployed based on crime typology
- Continuous case review processes to ensure optimal resource allocation
- Performance analytics driving investigative efficiency improvements
Structural and Social Advantages
Geographic and Demographic Factors
- Manageable Physical Environment
- Compact geographical area (728.6 km²) limiting escape options
- Controlled border points (airport and causeway) facilitate monitoring
- Dense urban environment with high surveillance coverage
- Limited international land borders reduce flight opportunities
- Digital Infrastructure
- Near-universal digital banking penetration creates comprehensive financial trails.
- High smartphone adoption enables digital evidence collection
- Centralised identification systems linking physical and digital identities
- Cashless transaction prevalence is increasing financial visibility
Societal Support System
- Public Cooperation
- High trust in law enforcement (above 90% in public surveys)
- Strong reporting culture with active citizen participation
- Community policing initiatives fostering information sharing
- Social stigma is associated with criminal behaviour
- Banking Sector Collaboration
- Proactive suspicious transaction reporting by financial institutions
- Direct communication channels between banks and investigators
- Rapid response protocols for account freezing and information sharing
- Joint training programs fostering mutual understanding of red flags
Case Study: Applying These Advantages to Money Laundering Investigations
Detection Phase Excellence
- Multi-Source Trigger Mechanisms
- Transaction monitoring systems flag anomalous patterns
- Scam victim reports rapidly linked to recipient accounts
- Cryptocurrency monitoring detects unusual exchange patterns
- International alerts about suspect fund movements
- Rapid Response Protocol
- Account freezing within hours of suspicious activity detection
- Immediate preservation of digital evidence across platforms
- Swift deployment of specialised financial crime investigators
- Early coordination with relevant financial institutions
Investigation Phase Efficiency
- Comprehensive Evidence Collection
- Complete digital footprint acquisition (devices, accounts, communications)
- Full transaction history reconstruction across multiple financial platforms
- Physical surveillance integration with digital intelligence
- Comprehensive witness testimony collection and analysis
- Advanced Analytics Application
- Network analysis reveals relationships between suspects
- Temporal correlation of physical and digital activities
- Communication pattern analysis revealing coordination
- Financial flow visualisation identifying the complete money trail
Prosecution Phase Effectiveness
- Strategic Charging Approach
- Multiple complementary charges addressing different aspects of criminal conduct
- Focus on provable elements with the most substantial evidence
- Careful selection of specific transactions with the most precise documentation
- Differentiated charging based on role and culpability
- Evidence Presentation Excellence
- Compelling visual representations of complex money flows
- Precise narrative construction connecting suspects to specific actions
- Technical evidence presented in accessible formats for courts
- Meticulous documentation meeting stringent evidential standards
Strategic Innovations Driving Success
Public-Private Partnership Model
- Project POET (Protect Older People Every Day)
- Banking staff trained to identify potential scam victims at branches
- Intervention protocols when elderly customers attempt unusual transfers
- Direct hotline to the Anti-Scam Centre for immediate verification
- This prevented an estimated $57 million in scam losses in 2023 alone
- ScamShield Initiative
- AI-powered app blocks likely scam calls and messages
- Crowdsourced reporting feeding machine learning models
- Real-time alert system for emerging scam typologies
- Integration with bank fraud detection systems
Early Intervention Focus
- “Break the Scam” Campaign
- Public education on immediate reporting within the “golden hour”
- Streamlined reporting channels (ScamShield app, 24/7 hotline)
- Automated triage system prioritising cases with recovery potential
- Success stories are publicised to encourage prompt reporting
- Money Mule Disruption Strategy
- Proactive identification of potential money mules
- Warning letters sent to individuals receiving suspicious transfers
- Educational outreach to high-risk demographics
- Bank account monitoring for typical mule transaction patterns
Challenges and Responses
Despite its success, Singapore faces evolving challenges:
- Overseas Jurisdictional Limitations
- Challenge: Scam masterminds often operate from beyond Singapore’s borders
- Response: Development of virtual task forces with key partner countries
- Innovation: Joint operations rooms for simultaneous multi-jurisdictional enforcement
- Technological Evolution
- Challenge: Criminals adopting privacy-enhancing cryptocurrencies
- Response: Investment in advanced blockchain analytics capabilities
- Innovation: Development of proprietary tools for privacy coin tracking
Singapore’s exceptional crime-solving rates, particularly for complex financial crimes, represent the culmination of these integrated advantages—technological, legal, organisational, and social. The money laundering case involving Tan, Jiang, and Wu exemplifies how this sophisticated ecosystem enables authorities to unravel even complex, multi-layered criminal operations with remarkable efficiency and effectiveness.
Methods to Prevent Money Muling in Singapore
Regulatory and Banking Measures
Transaction Monitoring Systems
- Real-time transaction monitoring utilizing AI to detect unusual patterns
- Intelligent systems flagging rapid deposits followed by withdrawals
- Automated alerts for transactions involving high-risk jurisdictions
- Velocity checks that identify unusual account activity rates
Account Opening Procedures
- Enhanced due diligence for new accounts, especially business accounts
- Biometric verification requirements for both in-person and digital onboarding
- Document authentication technology to verify identification documents
- Risk-based approach with additional scrutiny for higher-risk profiles
Banking Controls
- Delayed processing for extensive transactions
- Mandatory cooling periods before new payees can receive large transfers
- Default transaction limits requiring additional verification to increase
- Call-back verification for transactions above certain thresholds
Public Education and Awareness
Targeted Campaigns
- “Don’t Be A Money Mule” awareness programs in schools and universities
- Community outreach focusing on vulnerable populations (students, elderly, job seekers)
- Multi-language campaigns reaching diverse communities
- Specific education on the legal consequences, including jail time
Warning Systems
- SMS alerts about standard money mule recruitment techniques
- In-app banking notifications about the risks of allowing account access
- Digital banking platform pop-up warnings about suspicious transaction patterns
- Community alerts when new money mule recruitment tactics are detected
Law Enforcement Strategies
Proactive Investigation
- Dedicated Anti-Scam Centre resources for money mule network detection
- Collaboration between police and financial intelligence units
- Data analytics to identify connected accounts and transaction patterns
- Regular auditing of newly formed companies with unusual transaction patterns
Deterrence Through Prosecution
- Publicized cases showing severe penalties for money mules
- Prosecution of all participants in the chain, not just ring leaders
- Asset recovery procedures to trace and seize criminal proceeds
- Clear communication that ignorance is not an acceptable legal defense
Industry Collaboration
Information Sharing Networks
- Bank consortium sharing intelligence on suspicious patterns
- Cross-industry collaboration between telcos, banks, and payment providers
- A centralized database of known mule accounts and recruitment techniques
- Public-private partnerships for faster response to emerging threats
Technology Solutions
- Shared fraud detection engines across multiple financial institutions
- Digital footprint analysis (device, location, and behavioral data)
- Blockchain analytics to trace fund movements across platforms
- API-based systems allowing real-time information exchange between institutions
Targeted Vulnerability Reduction
Job Seeker Protection
- Partnership with job platforms to detect and remove suspicious job listings
- Education about legitimate versus suspicious job offers
- Warning systems on employment platforms
- Verification processes for companies advertising “financial agent” positions
Student-Focused Programs
- Campus awareness campaigns highlighting the risks
- Financial literacy modules covering scams and money mule awareness
- University partnerships with financial institutions for education
- Peer-to-peer awareness programs led by students themselves
International Cooperation
Cross-Border Coordination
- Information sharing agreements with other jurisdictions
- Joint investigation teams for transnational cases
- Standardized reporting formats for suspicious transaction reporting
- Rapid freeze mechanisms for cross-border fund movements
Regional Intelligence Framework
- ASEAN-wide cooperation on money laundering typologies
- Coordinated action against known crime groups operating regionally
- Harmonized KYC standards across regional financial institutions
- Shared blacklists of suspicious entities across borders
The most effective prevention strategy combines these approaches with continuous adaptation as criminals evolve their tactics. Singapore’s multi-agency approach involving the MAS, Singapore Police Force, and private sector partners has shown promising results, but ongoing vigilance remains essential.
Essential Fraud Detection Strategies
1. Multi-layered Authentication Systems
Modern authentication must go beyond passwords. A robust system should incorporate:
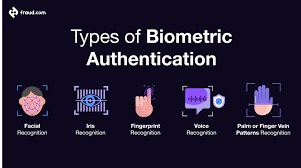
Biometric Verification using fingerprints, facial recognition, or voice authentication adds a physical dimension to security that’s difficult to replicate.
Device Intelligence examines the devices used to access accounts, flagging suspicious logins from unfamiliar devices or locations.
Behavioral Biometrics analyzes patterns in how users interact with devices—how they type, swipe, or navigate—creating a behavioral fingerprint that’s hard for fraudsters to mimic.
2. Machine Learning and AI Detection Systems
Artificial intelligence has transformed fraud detection from rules-based systems to sophisticated pattern recognition:
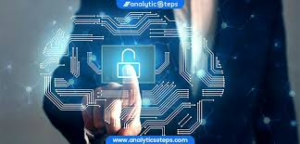
Anomaly Detection algorithms establish baseline behaviours for users and flag deviations from standard patterns. For example, if a user who typically makes small, local purchases suddenly attempts large international transactions, the system can automatically flag this for review.
Predictive Analytics examines historical fraud patterns to forecast potential vulnerabilities. These systems become increasingly accurate over time as they process more data and fraud scenarios.
Adaptive Authentication dynamically adjusts security requirements based on risk assessment. Low-risk transactions might proceed with minimal friction, while high-risk activities trigger additional verification steps.
3. Real-time Transaction Monitoring
Modern fraud detection must operate at the speed of digital transactions:
Velocity Checks look for suspicious patterns in the frequency of activities, such as multiple account creation attempts or rapid-fire transactions.
Network Analysis examines connections between accounts, identifying clusters of potentially fraudulent activity that might indicate organized fraud rings.
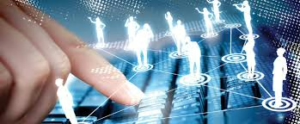
Geolocation Verification checks whether transaction locations make logical sense given a user’s history and profile.
4. Data Integration and Cross-channel Analysis
Effective fraud detection requires a holistic view across all channels and touchpoints:
Unified Customer Profiles combine data from various sources—mobile apps, websites, call centres, and physical locations—to create a comprehensive view of customer behaviour.
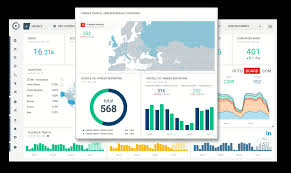
Cross-channel Pattern Recognition identifies suspicious activities that might appear normal when viewed in isolation but reveal fraud patterns when examined across channels.
Third-party Data Enrichment augments internal data with external information such as device reputation databases, known fraud networks, and compromised credential lists.
5. Advanced Analytics Tools: Implementation Examples
Let’s look at how these strategies might be implemented in practice with code examples:
Machine Learning Fraud Detection System
Click to open the code
Tap to open
This code example demonstrates several key concepts in fraud detection:
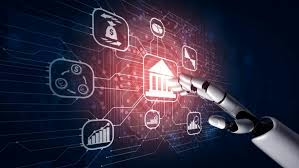
- Feature Engineering: The system calculates derived features that are strong fraud indicators, such as distance from home location, unusual transaction amounts compared to user history, and temporal patterns.
- Risk-based Decision Making: Rather than a binary approve/decline decision, the system implements a spectrum of responses based on both the risk score and transaction context.
- Machine Learning Implementation: The Random Forest model can capture complex, non-linear relationships between features and fraud likelihood, making it practical for detecting sophisticated fraud patterns.
- Explainability: The system analyzes feature importance, providing insight into which factors most strongly indicate fraud—crucial for improving the system and explaining decisions to customers and regulators.
6. Behavioral Analytics
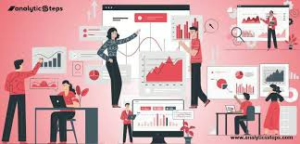
Beyond transaction details, modern fraud systems analyze how users behave:
Session Analysis examines user interaction patterns during a session, such as navigation paths, interaction speed, and hesitation points. Fraudsters often exhibit different behaviours than legitimate users when navigating financial interfaces.
Typing Patterns can reveal when a different person is using familiar credentials. Legitimate users develop consistent typing rhythms and patterns that are difficult to replicate.
Usage Consistency looks at whether behaviour matches patterns. For example, a user who constantly carefully reviews transaction details before confirming might raise flags if they suddenly rush through multiple high-value transactions.
7. Collaborative Security Approaches
Fraud detection is strengthened through industry cooperation:
Consortium Data Sharing allows financial institutions to pool anonymized fraud data, creating a more comprehensive picture of emerging threats while preserving customer privacy.
Regulatory Cooperation enables institutions to work with government agencies to identify large-scale fraud operations and money laundering networks.
Vendor Integration leverages specialized third-party security services that focus exclusively on specific types of fraud detection, adding another layer of protection.
Implementation Challenges and Solutions
Implementing fraud detection systems comes with significant challenges:
False Positives create friction for legitimate customers and can damage trust. Solutions include:
- Implementing risk-based authentication that adds friction only when necessary
- Using ensemble models that combine multiple detection approaches for greater accuracy
- Continuously tuning systems based on customer feedback and false positive analysis
Data Privacy Regulations such as GDPR and CCPA restrict how customer data can be used. Consider:
- Implementing privacy-by-design principles in fraud systems
- Using anonymization and pseudonymization techniques
- Creating clear data governance frameworks with documented legitimate interest in fraud prevention
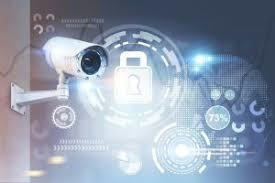
Integration Complexity across legacy and modern systems can impede effectiveness. Address this by:
- Using API-first approaches for system integration
- Implementing data transformation layers to normalize inputs from different systems
- Creating real-time event streams for fraud data rather than batch processing
Building a Fraud Prevention Culture
Technical solutions are only part of effective fraud prevention:
Employee Training should ensure that all staff members understand their role in preventing fraud, recognizing warning signs, and following security protocols.
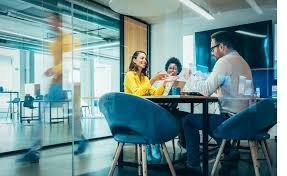
Customer Education helps users protect themselves by recognising phishing attempts, using strong authentication methods, and understanding how to report suspicious activities.
Regular Testing through penetration testing, red team exercises, and fraud simulations helps identify vulnerabilities before criminals can exploit them.
Measuring and Improving Your Fraud Detection System
Continuous improvement requires careful measurement:
Key Performance Indicators should include:
- False positive rate: Legitimate transactions incorrectly flagged
- False negative rate: Fraudulent transactions missed
- Detection speed: Time from fraud attempt to detection
- Customer impact metrics: Authentication success rates and friction points
A/B Testing allows you to compare different detection approaches and fine-tune systems based on real-world results rather than theoretical models.
Post-incident analysis should thoroughly examine confirmed fraud cases to identify how detection could have happened earlier or more efficiently.
Conclusion
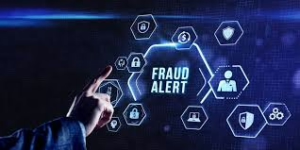
As fintech continues to transform the financial landscape, fraud detection must remain a top priority for businesses. By implementing multi-layered approaches that combine advanced technologies with human expertise, fintech companies can protect both their customers and their bottom line.
Remember that effective fraud prevention is not a static solution but an ongoing process that must continuously evolve to address new threats. By staying vigilant and investing in robust detection systems, your business can build customer trust while minimizing losses in an increasingly digital financial world.
Secure browsing
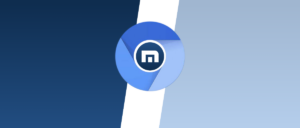
When it comes to staying safe online, using a secure and private browser is crucial. Such a browser can help protect your personal information and keep you safe from cyber threats. One option that offers these features is the Maxthon Browser, which is available for free. It comes with built-in Adblock and anti-tracking software to enhance your browsing privacy.
By utilising the Maxthon Browser, users can browse the internet confidently, knowing that their online activities are shielded from prying eyes. The integrated security features alleviate concerns about potential privacy breaches and ensure a safer browsing environment. Furthermore, the browser’s user-friendly interface makes it easy for individuals to customise their privacy settings according to their preferences.
Maxthon Browser not only delivers a seamless browsing experience but also prioritises the privacy and security of its users through its efficient ad-blocking and anti-tracking capabilities. With these protective measures in place, users can enjoy the internet while feeling reassured about their online privacy.
In addition, the desktop version of Maxthon Browser works seamlessly with their VPN, providing an extra layer of security. By using this browser, you can minimise the risk of encountering online threats and enjoy a safer internet experience. With its combination of security features, Maxthon Browser aims to provide users with peace of mind while they browse.
Maxthon Browser is a reliable choice for users who prioritise privacy and security. With its robust encryption measures and extensive privacy settings, it offers a secure browsing experience that gives users peace of mind. The browser’s commitment to protecting user data and preventing unauthorised access sets it apart in the competitive web browser market.