AI-Powered Cybercrime Threats to Financial Institutions: Analysis and Prevention Strategies
The Evolution of Cybercrime Targeting Financial Institutions
The article highlights a fundamental shift in cybersecurity: identity has become the new perimeter. This represents a critical evolution in how cybercriminals operate, particularly with AI accelerating these capabilities.
Identity-Based Attack Vectors
Financial institutions face sophisticated identity-based threats that leverage AI in several ways:
- Credential Abuse at Scale: AI has transformed credential stuffing from a manual process to a highly automated one. Attackers can now test millions of stolen credentials across banking platforms in minutes, with machine learning optimizing which combinations to try first.
- Enhanced Social Engineering: Today’s AI-powered social engineering goes far beyond traditional phishing:
- AI generates contextually relevant, grammatically perfect phishing communications
- Deepfake voice technology can convincingly impersonate banking representatives
- Machine learning analyses social media and public data to craft hyper-personalized attacks
- Token and Session Hijacking: Rather than cracking passwords, sophisticated attackers target SSO tokens and active sessions, effectively bypassing traditional authentication controls altogether.
- Vulnerability Exploitation Automation: AI systems can continuously probe for weaknesses in banking infrastructure, identifying and exploiting vulnerabilities faster than security teams can patch them.
Institutional Vulnerability Disparity
A concerning trend emerges when examining vulnerability by institution size:
- Regional banks experience 12% more login-related security incidents than larger institutions
- Credit unions face an alarming 52% higher rate of such incidents
- This disparity exists mainly due to resource limitations in cybersecurity infrastructure
Comprehensive Prevention Strategies
AI-Powered Defence Systems
To counter AI threats, financial institutions must deploy equally sophisticated defences:
- Behavioural Analytics and Anomaly Detection:
- Real-time monitoring of user behaviour patterns
- AI models that establish behavioural baselines for each customer
- Immediate flagging of statistical anomalies in transaction patterns, login locations, or device usage
- Advanced Identity Verification:
- Multi-factor authentication incorporating biometrics
- Continuous authentication that monitors behaviour throughout a session
- Risk-based authentication that adjusts security requirements based on context
- Access Management and Privilege Control:
- AI-driven auditing of user access rights
- Automated identification and deprovisioning of dormant accounts
- Just-in-time access provisioning instead of persistent privileges
- Phishing-Resistant Authentication:
- Implementation of passkeys and FIDO2 standards
- Passwordless authentication solutions like Okta FastPass
- Hardware security keys for high-value transaction authorisation
Organizational Response Requirements
Beyond technological solutions, financial institutions must transform their security operations:
- Automation of Security Processes:
- Security orchestration and automated response (SOAR) systems
- AI-powered security monitoring that operates continuously
- Automated threat intelligence gathering and integration
- Resilience Planning:
- Segregation of critical systems from internet-facing services
- Regular tabletop exercises simulating AI-powered attacks
- Recovery systems are designed to restore operations quickly after a compromise
- Security Culture Development:
- Regular security awareness training incorporating the latest AI threat scenarios
- Development of internal expertise in AI security
- Creation of a cybersecurity-conscious organizational culture
Impact on Singapore’s Financial Sector
While the article doesn’t specifically address Singapore, the city-state’s position as a global financial hub makes it particularly vulnerable to these threats:
Singapore-Specific Vulnerabilities
- High Digital Banking Adoption: Singapore has one of the highest digital banking penetration rates globally (approximately 94% of the population), creating a large attack surface.
- Concentration of Financial Assets: Singapore presents a high-value target as a financial centre, managing roughly $3.5 trillion in assets under management.
- Regional Headquarters Effect: Many financial institutions use Singapore as their APAC headquarters, potentially creating single points of failure for regional operations.
Singapore’s Advantages in Cybersecurity Resilience
- Strong Regulatory Framework: The Monetary Authority of Singapore (MAS) has implemented robust cybersecurity requirements for financial institutions:
- Technology Risk Management Guidelines
- Regular industry-wide security exercises
- Mandatory incident reporting requirements
- Advanced Digital Infrastructure: Singapore’s sophisticated technical infrastructure enables the implementation of advanced security solutions.
- Public-Private Collaboration: Close coordination between government agencies like the CSA (Cyber Security Agency) and financial institutions creates strong threat intelligence sharing.
Economic Implications
The impact of AI-powered cybercrime on Singapore’s financial sector could be significant:
- Direct Financial Losses: Beyond immediate theft, remediation costs and operational disruptions could impact financial performance.
- Reputational Damage: Singapore’s reputation as a secure financial hub could be damaged by high-profile breaches.
- Regulatory Response: Inevitable tightening of regulatory requirements would increase compliance costs across the sector.
- Innovation Impediment: Excessive security concerns could slow the adoption of beneficial AI technologies in the financial sector.
Conclusion: The Arms Race Accelerates
Financial institutions face an unprecedented acceleration in the cybersecurity arms race. AI has shifted the advantage to attackers in terms of speed, scale, and sophistication. The only viable response is equally sophisticated AI-powered defences operating at machine speed.
The stakes couldn’t be higher for Singapore’s financial sector, specifically. While well-positioned with strong regulatory oversight and technical infrastructure, the concentration of financial assets makes it an especially attractive target. Success will require not just technological solutions but organisational transformation—creating security operations that can match the speed and adaptability of AI-powered threats.
Impact of AI-Powered Cybercrime on Singapore’s Banking Sector
Singapore’s Unique Banking Landscape
Singapore stands as a premier global financial hub with distinctive characteristics that shape its cybersecurity risk profile:
Strategic Position and Infrastructure
- Banking Density: With over 200 banks operating in a small geographic area, Singapore has one of the highest concentrations of banking assets per capita globally.
- Digital Banking Evolution:
- Digital-only banks like Trust Bank, GXS Bank, and Maribank launched in recent years
- Traditional players like DBS, OCBC, and UOB have heavily invested in digital transformation
- Nearly complete mobile banking penetration among the working-age population
- Regional Financial Gateway: Singapore processes approximately 15-20% of all ASEAN financial transactions, making it a critical infrastructure node.
Specific Threat Vectors for Singapore Banks
The AI-powered threats mentioned in the article manifest with particular characteristics in Singapore’s context:
Identity-Based Attack Concerns
- National Digital Identity Integration: While secure, Singapore’s SingPass and CorpPass national digital identity systems represent high-value targets. Compromising these systems could potentially provide access across multiple financial services.
- Cross-Border Identity Verification Challenges: Singapore banks serve clients across Southeast Asia, where identity documentation standards vary significantly, creating verification challenges that attackers can exploit.
- Wealth Management Focus: The high concentration of private banking and wealth management services means attackers can target high-net-worth individuals with sophisticated spear-phishing campaigns, potentially yielding greater returns than mass attacks.
Regulatory and Operating Environment Impact
- MAS Technology Risk Management Guidelines: Singapore’s central bank imposes strict cybersecurity requirements, creating a higher baseline of protection but also compliance costs:
- Mandatory technology risk assessments
- Incident reporting requirements
- Business continuity planning specifically for cyber incidents
- Cross-Border Operations Complexity: Most Singapore banks operate across multiple ASEAN countries, creating inconsistent security environments and potential weak points in their defences.
Economic and Operational Impact Assessment
Direct Financial Implications
- Fraud Loss Escalation: Using conservative estimates based on global trends:
- Singapore banks could face AI-enhanced fraud losses increasing 15-25% annually
- Smaller banks would likely experience disproportionately higher losses per asset value
- Security Investment Requirements:
- Banks may need to increase cybersecurity budgets by 30-40% to counter AI threats
- DBS, Singapore’s largest bank, disclosed cybersecurity investments exceeding $650M annually in recent reports
- Smaller institutions face difficult resource allocation decisions
Operational Disruption Scenarios
- Customer Trust Erosion: Singapore’s banking sector has built strong customer trust. AI-powered attacks could rapidly erode this trust through:
- Deepfake scams impersonating bank representatives
- Selective account compromises targeting influential customers
- Mass credential theft incidents affecting thousands simultaneously
- Regulatory Consequences:
- MAS has demonstrated a willingness to impose significant penalties for security lapses
- Beyond financial penalties, MAS can restrict growth activities and new product launches.
- Additional business restrictions could impact competitiveness in the aggressive ASEAN market.t
Competitive Positioning Effects
- Security as Differentiator: Some Singapore banks are repositioning cybersecurity as a customer-facing value proposition rather than just a back-office function.
- Innovation Tension: Banks face competing pressures:
- Need to rapidly deploy AI-powered services to remain competitive
- Requirement to thoroughly secure these same systems against AI-powered attacks
- Finding g balance between innovation speed and security will determine market leaders
Singapore-Specific Mitigation Strategies
Building on the article’s general approaches, Singapore banks require tailored strategies:
Leveraging National Infrastructure
- National Digital Identity Integration: Enhanced integration with Singapore’s national digital identity systems can provide an additional security layer difficult for foreign attackers to compromise.
- SGFinDex Expansion: Singapore’s Financial Data Exchange could be expanded beyond its current scope to include security-relevant data sharing between institutions.
- Cybersecurity Talent Development: Partnering with government initiatives like the TechSkills Accelerator (Tesa) to address the critical shortage of cybersecurity professionals.
Regulatory Collaboration Opportunities
- Industry-Wide Security Exercises: Participation in MAS-led industry-wide cybersecurity exercises that specifically simulate AI-powered attacks.
- Information Sharing Framework: Enhanced participation in the Financial Services Information Sharing and Analysis Centre (FS-ISAC) with AI-specific threat intelligence.
- Regulatory Sandboxes: Working with MAS to test innovative security approaches through regulatory sandboxes before full deployment.
Long-Term Strategic Considerations
Structural Changes to Banking Operations
- Zero-Trust Architecture Implementation: Singapore banks should accelerate the adoption of zero-trust principles, in which no user or system is inherently trusted, regardless of location or network.
- AI Security Operations Centres: Development of specialized AI-powered security operations centres that can match the speed and sophistication of attacks.
- Security-by-Design Mandate: Embedding security requirements into the earliest stages of product development rather than adding them later.
Regional Leadership Opportunity
- ASEAN Cybersecurity Standards Advocacy: Singapore banks can drive the adoption of higher security standards across the region, reducing vulnerability from cross-border operations.
- Security Technology Export: Expertise developed defending Singapore’s banking system could become a service exportable to other ASEAN financial institutions.
Conclusion: Balancing Innovation and Security
Singapore’s banking sector faces a critical inflexion point. The same AI technologies driving competitive innovation also empower increasingly sophisticated attacks. Success will require:
- Defence at Machine Speed: Implementation of autonomous security systems capable of detecting and responding to threats in milliseconds rather than hours.
- Collaborative Defence Ecosystems: No single institution can defend alone—sharing threat intelligence and defence strategies becomes essential.
- Customer Education Evolution: Moving beyond simple awareness to developing true security partnerships with customers.
The banks that most effectively balance aggressive digital transformation with sophisticated AI-powered security will likely emerge as the leaders in Singapore’s highly competitive banking market. However, this requires fundamental rethinking of security as a strategic business function rather than a compliance requirement.
Analysis: How MAS and CSA May Adjust Cybersecurity Policy in Singapore to Counter AI-Powered Financial Threats
Current Regulatory Framework
To understand potential policy adjustments, we must first examine Singapore’s existing cybersecurity governance structure:
Monetary Authority of Singapore (MAS) Current Approach
- Technology Risk Management (TRM) Guidelines:
- Last significantly updated in January 2021
- Focuses on governance, security operations, and system resilience
- Contains specific requirements for access control, cryptography, and vulnerability assessment
- Notice on Cyber Hygiene (MAS 655):
- Legally binding requirements established in 2019
- Mandates technical controls like multi-factor authentication
- Sets baseline security expectations for all financial institutions
- Business Continuity Management Guidelines:
- Requires recovery strategies specifically for cybersecurity incidents
- Mandates regular exercise and testing of recovery capabilities
Cyber Security Agency (CSA) Current Framework
- Cybersecurity Act (2018):
- Establishes a legal framework for protecting Critical Information Infrastructure (CII)
- The banking and finance sector is designated as CII under the Act
- Provides powers for cybersecurity threat monitoring and response
- Singapore Cybersecurity Strategy 2.0 (released 2021):
- Emphasizes building a resilient infrastructure
- Focuses on safeguarding cyberspace
- Encourages international partnerships
- Develops a vibrant cybersecurity ecosystem
Likely Policy Adjustments by MAS
In response to AI-powered financial cyberthreats, MAS is likely to implement several key policy adjustments:
1. Enhanced AI Governance Framework Extension
MAS’s existing AI governance framework primarily focuses on ethical AI use but will likely be expanded to include mandatory AI security requirements:
- AI Security Assessment Requirements: New regulations require financial institutions to assess the security implications of AI deployments before implementation
- AI Security Testing Standards: Formalized methodologies for testing AI system resilience against adversarial attacks
- Explainable AI Mandate: Requirements for critical financial systems to use interpretable AI models that can be audited for security vulnerabilities
2. Real-Time Threat Intelligence Requirements
- Mandatory Participation in Information Sharing: Currently, voluntary participation in threat sharing may become required for licensed financial institutions.s
- Technical Integration Standards: Specifications for automated threat intelligence sharing in machine-readable formats that security systems can act upon immediately
- Cross-Border Intelligence FrameworkFormaliseded processes for sharing financial threat intelligence with partner nations’ financial regulators
3. Enhanced Authentication and Identity Requirements
- Phishing-Resistant Authentication Mandate: Potential phase-out timeline for password-based authentication in favour of FIDO2/WebAuthn standards
- Continuous Authentication Requirements: New guidelines requiring behavioural monitoring throughout customer sessions rather than just at login
- Digital Identity Integration: Deeper integration requirements with Singapore’s National Digital Identity ecosystem
4. AI-Specific Security Testing Regime
- AI Red Team Exercises: Specialised penetration testing requirements specifically targeting AI components of financial systems
- Adversarial Testing Standards: Formal methodologies for testing AI system resilience against model poisoning, prompt injection, and other AI-specific attack vectors
- Synthetic Data Testing: Guidelines for creating and using synthetic data in security testing to protect customer privacy while enabling comprehensive testing
Likely Policy Adjustments by CSA
The Cyber Security Agency will likely adapt its broader national cybersecurity framework in ways that complement MAS’s financial sector focus:
1. Critical AI Infrastructure Classification
- Expansion of CII Definition: Formal designation of specific AI systems within the financial sector as Critical Information Infrastructure
- Enhanced Protection RequirementsSpecialiseded security controls for AI systems designated as critical infrastructure
- Incident Reporting Framework: Specific reporting requirements for incidents involving AI systems in critical infrastructure
2. National-Level Threat Monitoring Capabilities
- AI-Powered National Monitoring: Expansion of National Cyber Security Centre capabilities to incorporate AI-specific threat monitoring
- Centralised Threat Intelligence: Development of financial sector-specific threat feeds distributed to all Singapore financial institutions
- Advanced Persistent Threat (APT) Detection: Enhanced capabilities to identify nation-state actors targeting Singapore’s financial infrastructure
3. Cybersecurity Talent Development Initiatives
- Specialized AI Security Training: New programs explicitly focused on AI security in partnership with universities and industry
- Financial Sector Security Certification: Development of Singapore-specific certification for financial cybersecurity professionals
- Overseas Talent Attraction: Targeted immigration policies to attract experienced cybersecurity professionals with AI expertise
4. International Regulatory Coordination
- ASEAN Cybersecurity Standards Leadership: Singapore positioning as the regional leader in developing binding standards for financial cybersecurity
- International Enforcement Cooperation: Enhanced agreements with international partners for pursuing cross-border financial cybercrime
- Global AI Security Standards Participation: Active engagement in international standard-setting bodies developing AI security frameworks
Implementation Timeline and Approach
Based on historical regulatory patterns, we can project the likely implementation approach:
Phase 1: Immediate Guidance (Next 3-6 Months)
- Joint MAS-CSA Advisory: Issuance of non-binding guidance on AI security best practices
- Vulnerability Disclosure Framework: Updated protocols for reporting AI vulnerabilities
- Industry Consultation Launch: Beginning formal consultation processes on regulatory changes
Phase 2: Interim Requirements (6-12 Months)
- TRM Guidelines Update: Revision incorporating AI-specific security controls
- Notice on AI Security: Potential new legally binding notice similar to MAS 655
- Pilot Monitoring Program: Selected institutions participate in enhanced threat monitoring
Phase 3: Comprehensive Regulation (12-24 Months)
- Full Regulatory Framework: Complete integration of AI security into the regulatory structure
- Compliance Timeline: Phased implementation requirements based on institution size
- Certification Regime: Formal certification requirements for critical AI systems
Potential Challenges and Industry Impact
Implementation Barriers
- Technical Complexity: Developing regulations that address advanced AI threats without stifling innovation will prove challenging
- Global Coordination: Singapore’s financial institutions operate globally, creating compliance challenges when regulations differ across jurisdictions
- Resource Constraints: Smaller financial institutions may struggle with compliance costs, potentially driving consolidation
Economic and Competitive Implications
- Short-Term Cost Burden: Initial compliance costs may impact the profitability of Singapore financial institutions
- Long-Term Competitive Advantage: A Stronger security posture could become a competitive differentiator in the regional market
- Innovation Impact: Careful balance required to prevent security requirements from hampering beneficial AI innovation
Privacy and Civil Liberty Considerations
- Enhanced Monitoring Concerns: Expanded threat monitoring capabilities raise potential privacy questions
- Cross-Border Data Sharing: International threat sharing must navigate complex data sovereignty issues
- Security-Privacy Balance: Maintaining an appropriate balance between security needs and privacy protections
Conclusion: Singapore’s Regulatory Evolution
Singapore’s regulators face the challenge of developing a framework that addresses rapidly evolving AI-powered threats while maintaining the city-state’s status as a financial innovation hub. Based on their historical approach, we can expect a measured, consultative process resulting in comprehensive but pragmatic regulations.
The most likely outcome is a multi-layered approach combining:
- Principles-Based Requirements: Broad guidance on security outcomes rather than prescriptive technical solutions
- Risk-Based Implementation: Tailored requirements based on institution size and systemic importance
- International Alignment: Coordination with global standards while maintaining Singapore-specific protections
- Public-Private Partnership: Continued emphasis on industry collaboration rather than purely top-down regulation
For Singapore’s financial institutions, preparing for this regulatory evolution means investing in AI security capabilities now, participating actively in consultations, and developing internal expertise in areas like adversarial machine learning and AI model security. Those who move proactively will be well-positioned when formal requirements inevitably arrive.
Countering AI-Powered Cyber Threats with AI-Driven Cybersecurity
The AI Security Arms Race in Financial Services
The emergence of AI-powered cyber threats has fundamentally altered the security landscape for financial institutions. As attackers deploy increasingly sophisticated AI systems to breach defences, financial organizations must respond with equally advanced AI-driven security solutions. This analysis explores comprehensive strategies for implementing effective AI countermeasures.
Understanding the Adversarial AI Landscape
Attacker Capabilities
Modern AI-powered attacks against financial institutions typically leverage:
- Machine Learning for Target Selection
- AI systems analyze financial institutions for vulnerabilities
- Algorithms identify high-value targets based on potential return
- Systems prioritize attack vectors with the highest probability of success
- Natural Language Processing for Social Engineering
- Generation of personalized phishing communications
- Real-time chatbot interactions mimicking support staff
- Voice synthesis for vishing (voice phishing) attacks
- Reinforcement Learning for Adaptive Attacks
- Attack patterns that evolve based on defensive responses
- Progressive probing that adjusts to avoid detection thresholds
- Learning systems that identify and exploit pattern-based defences
- Computer Vision for CAPTCHA/Visual Security Bypass
- Automated solving of visual security challenges
- Manipulation of document verification systems
- Biometric authentication spoofing
Emerging Threat Patterns
Financial institutions face emerging attack methodologies, including:
- Prompt Injection and Model Exploitation: Manipulating financial institutions’ own AI systems through carefully crafted inputs
- Poisoning Attacks: Corrupting training data for security AI systems
- EvasiveBehaviourr: Malware that detects when it’s being analysed and alters behaviour
- Distributed Coordination: Swarm-based attacks from multiple access points with shared intelligence
AI-Powered Defence Architecture
1. Intelligent Threat Detection Systems
Real-Time Pattern Recognition
- Deploy deep learning models trained on financial transaction patterns
- Implement neural networks designed to detect anomalous user behaviour
- Utilisez un supervised learning to identify emerging threat patterns without prior examples
Case Study: Singapore-based DBS Bank implemented deep learning systems that reduced false favourable rates by 80% while increasing fraud detection by 24%, analyzing over 5 million transactions daily for anomalous patterns.
Implementation Strategy:
- Begin with supervised learning systems using labelled threat data
- Progressively incorporate semi-supervised approaches as capabilities mature
- Develop specialised models for different transaction types and customer segments
2. Advanced Identity Verification and Authentication
Continuous Authentication Systems
- Deploy behavioural biometrics that continuously analyse user patterns
- Implement risk-based authentication that adjusts security dynamically
- Utilise federated learning for privacy-preserving authentication models
Multi-Modal Biometric Verification
- Implement facial recognition with liveness detection
- Deploy voice pattern analysis resistant to synthesis attacks
- Utilise keystroke dynamics and mouse movement analysis
Implementation Strategy:
- Layer authentication factors rather than relying on a single modality
- Implement progressive security based on transaction risk level
- Ensure authentication systems maintain usability while enhancing security
3. AI-Powered Security Operations
Autonomous Response Capabilities
- Deploy security orchestration systems with automated response playbooks
- Implement ML-based triage of security alerts
- Utilise reinforcement learning to improve response effectiveness over time
Threat Hunting with Machine Learning
- Deploy unsupervised anomaly detection across the network infrastructure
- Implement graph analytics to identify hidden relationships in security data
- Utilise natural language processing to analyze internal communications for insider threats
Implementation Strategy:
- Begin with human-in-the-loop systems before advancing to fully autonomous responses
- Focus initial automation on high-volume, low-complexity threats
- Develop clear metrics for measuring autonomous system effectiveness
4. Defensive AI Training and Hardening
Adversarial Training
- Subject internal AI systems to simulated attacks
- Implement red team exercises specifically targeting AI components
- Utilise generative adversarial networks to strengthen defences
Security by Design for AI Systems
- Implement formal verification of critical AI components
- Deploy runtime application self-protection for AI systems
- Ensure transparent design with explainable AI approaches
Implementation Strategy:
- Establish dedicated AI security testing teams
- Implement adversarial robustness testing as standard development practice
- Create formal review processes for AI system security
Implementation Framework for Financial Institutions
Phase 1: Foundation Building (0-6 months)
Security Data Infrastructure
- Establish comprehensive data collection across all channels
- Implement data quality controls and governance
- Create segregated environments for testing AI security systems
Talent and Capability Development
- Recruit specialists in AI security and financial fraud
- Develop cross-training programs for existing security personnel
- Establish partnerships with AI security research organisations
Governance Framework
- Create AI security risk assessment methodologies
- Establish ethical guidelines for defensive AI usage
- Develop metrics for measuring AI security effectiveness
Phase 2: Initial Deployment (6-12 months)
Pilot Implementation
- Deploy supervised learning detection systems
- Implement basic behavioural analytics
- Establish human-in-the-loop response automation
Testing and Validation
- Conduct controlled red team exercises against AI systems
- Perform adversarial testing of authentication mechanisms
- Validate detection capabilities against known attack patterns
Integration with Existing Security
- Connect AI systems with SIEM platforms
- Implement orchestration between traditional and AI security
- Establish escalation paths from AI to human analysts
Phase 3: Advanced Capabilities (12-24 months)
Autonomous Security Operations
- Scale automated response capabilities
- Implement cross-channel correlation
- Deploy pre-emptive threat hunting
Ecosystem Defense
- Extend AI security to partner connections
- Implement customer-facing fraud prevention
- Deploy supply chain security monitoring
Continuous Improvement
- Establish feedback loops for model refinement
- Implement automated effectiveness measurement
- Deploy ongoing adversarial testing programs
Measuring Effectiveness
Key Performance Indicators
Detection Metrics
- Actual positive rate for known attack vectors
- Time to detection for novel threats
- False positive reduction compared to traditional systems
Response Metrics
- Mean time to respond to identified threats
- Automation rate for common attack scenarios
- Response effectiveness (measured by outcome)
Business Impact Metrics
- Reduction in fraud losses
- Customer friction measurements
- Security operations efficiency improvements
Return on Investment Calculation
Financial institutions should measure AI security ROI through:
- Direct loss prevention (fraud reduction)
- Operational efficiency gains (analyst time savings)
- Regulatory compliance cost reduction
- Reputational damage avoidance
Case Study: A mid-sized ASEAN bank implemented AI-driven security with an initial investment of $3.2M and achieved:
- 47% reduction in fraud losses
- 35% improvement in analyst efficiency
- 22% reduction in false positives
- ROI breakeven at 14 months
Risk Mitigation Strategies
Technical Risks
AI System Vulnerabilities
- Implement defence-in-depth with traditional security layers
- Regular penetration testing of AI systems
- Maintain manual override capabilities
Data Poisoning Defence
- Implement strict input validation for learning systems
- Deploy anomaly detection for training data
- Utilise federated learning to reduce centralized data exposure
Operational Risks
Overdependence on Automation
- Maintain human oversight capabilities
- Implement progressive automation with careful validation
- Regular “offline” testing of manual processes
Skills Gap Management
- Develop internal talent through specialized training
- Establish partnerships with security service providers
- Create knowledge transfer processes from vendors
Singapore-Specific Implementation Considerations
Regulatory Alignment
MAS Guidelines Compliance
- Ensure AI systems meet Technology Risk Management requirements
- Establish explainability for AI decisions to satisfy regulatory requirements
- Implement comprehensive audit trails for AI security actions
Privacy Compliance
- Design AI security systems in compliance with PDPA requirements
- Implement data minimization in security analytics
- Establish a clear purpose and limitation for the collected data
Local Ecosystem Integration
National Initiatives Participation
- Integrate with CSA’s national monitoring capabilities
- Participate in MAS’s financial threat sharing platforms
- Align with Singapore’s National AI Strategy security components
Industry Collaboration
- Participate in the Association of Banks Singapore security working groups
- Establish information sharing protocols with local financial institutions
- Collaborate with local universities on AI security research
Conclusion: Building Sustainable AI Security
As AI-powered threats continue to evolve, financial institutions must develop sustainable approaches to AI security that can adapt to emerging challenges. Key success factors include:
- Cultural Integration: Security awareness must extend to understanding AI-specific threats throughout the organization
- Technological Flexibility: Security architectures must accommodate rapid evolution in both threats and defensive capabilities
- Ecosystem Approach: No single institution can defend alone against sophisticated AI-powered threats
- Ethical Boundaries: Even defensive AI must operate within clear ethical guidelines
By implementing comprehensive AI security strategies, financial institutions can not only defend against current threats but also build adaptable systems capable of evolving alongside the threat landscape. The financial institutions that most effectively harness AI for defence will likely gain significant competitive advantages in customer trust and operational resilience.
Implementation Strategy
Effective privacy protection requires a layered approach combining multiple solutions:
- Immediate Term: Focus on transparency, consent improvements, and education
- Medium Term: Implement technical safeguards and accountability mechanisms
- Long Term: Develop advanced infrastructure for privacy-preserving AI ecosystems
The most promising approach involves combining technical safeguards with appropriate governance frameworks and empowering individuals through both tools and knowledge. This creates a comprehensive privacy protection system that addresses the unique challenges of AI while enabling beneficial innovation to continue.Retry
Changing Expectations
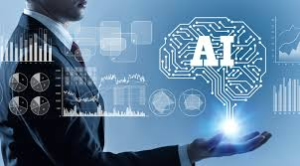
In 2018, 76% of senior banking leaders believed AI would be critical for market differentiation.
Early predictions were dramatic, with some suggesting AI could replace half of bank staff.
The actual impact is turning out to be more nuanced and less disruptive than initially feared.
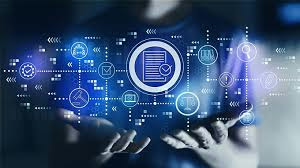
Primary AI Applications in Banking
Customer service improvement
Personalized recommendations based on payment history
Chatbots for handling common customer queries
Seamless handover to human representatives when needed
Optimizing cash flow management
Helping less tech-savvy customers navigate digital banking services
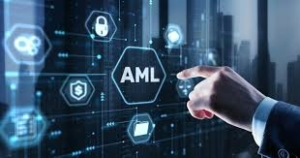
Key Challenges in AI Adoption
Integrating AI with existing legacy banking systems
Accessing and leveraging existing customer data
Avoiding creation of new technological silos
Ensuring smooth, frictionless customer experiences
Innovative Approach Example
The article highlights Auriga’s WinWebServer (WWS) AI module as an innovative solution that:
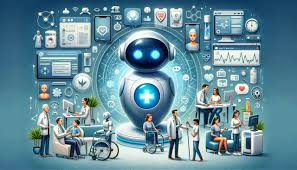
Seamlessly integrates with legacy systems.
Enables enhanced customer experiences
Speeds up strategic decision-making
Helps optimize cash management across bank branches and ATMs
Subtle but Significant Impact
Rather than replacing human workers, AI is primarily being used to augment and improve banking services, making them more personalized, efficient, and accessible.
The article suggests that AI in banking is less about disruption and more about incremental, strategic improvements to existing banking processes and customer interactions.RetryCanalyse ai as transformation not disruptionEditI’ll provide an analysis of AI as a transformative force in banking, expanding on the themes from the article and drawing insights about how AI is fundamentally reshaping the banking landscape.
AI: A Transformative Force in Banking
Conceptual Shift: From Disruption to Transformation
AI represents a fundamental transformation of banking, not merely a disruptive technology. This transformation is characterized by:
1. Intelligent Personalization
- Beyond traditional customer segmentation
- Real-time, individual-level personalization of financial services
- Predictive understanding of customer needs before they arise
- Customized financial advice and product recommendations tailored to individual financial behaviors and life stages
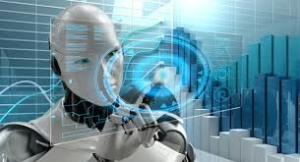
2. Operational Intelligence
- Reimagining banking processes through cognitive automation
- Moving from rule-based systems to adaptive learning systems
- Continuous optimization of internal operations
- Predictive maintenance of financial infrastructure
- Dynamic risk management and compliance monitoring
3. Customer Experience Reimagined
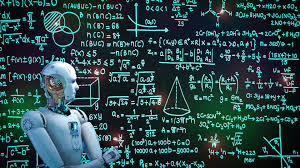
- Shift from transactional interactions to contextual, anticipatory engagement
- 24/7 intelligent support systems
- Seamless omnichannel experiences
- Proactive financial guidance
- Accessibility-driven design that serves diverse customer capabilities
4. Strategic Decision Making
- AI as a strategic partner in executive decision-making
- Advanced scenario modeling
- Real-time market trend analysis
- Enhanced predictive capabilities for investment and risk strategies
- Democratization of sophisticated financial insights
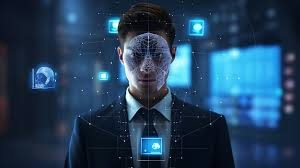
5. Ethical and Inclusive Innovation
- Using AI to address historical banking inequities
- Creating more transparent and fair financial assessment models
- Developing inclusive financial products
- Reducing human bias in financial decision-making
- Supporting underserved financial populations through intelligent design
Transformation Characteristics
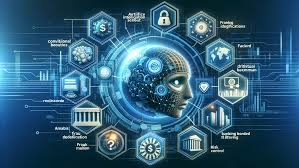
- Evolutionary, Not Revolutionary: Gradual integration that builds upon existing systems
- Augmentative Intelligence: Enhancing human capabilities, not replacing them
- Adaptive Learning: Continuous improvement through sophisticated machine learning
- Holistic Approach: Addressing multiple banking dimensions simultaneously
Future Outlook
The true power of AI in banking lies not in replacing human workers but in creating a symbiotic ecosystem where technological intelligence and human expertise collaborate to deliver unprecedented value.
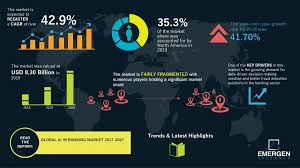
Maxthon
Maxthon has set out on an ambitious journey aimed at significantly bolstering the security of web applications, fueled by a resolute commitment to safeguarding users and their confidential data. At the heart of this initiative lies a collection of sophisticated encryption protocols, which act as a robust barrier for the information exchanged between individuals and various online services. Every interaction—be it the sharing of passwords or personal information—is protected within these encrypted channels, effectively preventing unauthorised access attempts from intruders.
Maxthon private browser for online privacyThis meticulous emphasis on encryption marks merely the initial phase of Maxthon’s extensive security framework. Acknowledging that cyber threats are constantly evolving, Maxthon adopts a forward-thinking approach to user protection. The browser is engineered to adapt to emerging challenges, incorporating regular updates that promptly address any vulnerabilities that may surface. Users are strongly encouraged to activate automatic updates as part of their cybersecurity regimen, ensuring they can seamlessly take advantage of the latest fixes without any hassle.
In today’s rapidly changing digital environment, Maxthon’s unwavering commitment to ongoing security enhancement signifies not only its responsibility toward users but also its firm dedication to nurturing trust in online engagements. With each new update rolled out, users can navigate the web with peace of mind, assured that their information is continuously safeguarded against ever-emerging threats lurking in cyberspace.
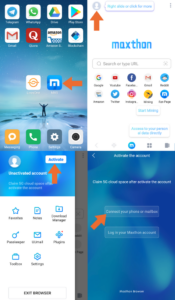