Analysis of Arguments Against Using AI for Learning
Fundamental Arguments Against AI in Learning
The article presents several compelling arguments against using generative AI tools like Chatgpt for learning:
1. Hindering Fundamental Skill Development
The article argues that AI shortcuts bypass critical developmental processes. For example, the 5-year-old learning spelling through Chatgpt is merely memorising without understanding letter-sound relationships (phonics). Similarly, asking AI for math solutions circumvents the cognitive development that comes from manipulating physical objects to understand numerical concepts.
2. Skill Atrophy and Dependency
The Microsoft/Carnegie Mellon study cited shows knowledge workers experienced skill deterioration in areas they outsourced to AI. This created a negative feedback loop where reduced confidence led to greater AI dependency, ultimately diminishing self-efficacy in core competencies like writing and analysis.
3. Standardisation of Thinking
The research indicated that AI users produced “a less diverse set of outcomes for the same task,” suggesting that AI might homogenise thinking patterns. This is particularly concerning for the development of creative and divergent thinking.
4. Superficial Learning
The article suggests that AI enables students to bypass the productive struggle necessary for deep learning. The tutor’s frustration with “bland and boring robo essays” highlights how AI might produce adequate but uninspired work that lacks personal voice and depth.
Impact on Critical Thinking Skills
The article identifies several critical thinking impacts:
- Reduced analytical practice: When AI summarises or analyzes, students lose opportunities to develop these skills themselves
- Decreased verification habits: The Microsoft study showed users increasingly accepted AI outputs without verification
- Diminished cognitive resilience: The productive struggle described in the author’s university essay-writing experience builds mental resilience that AI shortcuts circumvent
- Underdeveloped conceptual understanding: Learning fundamentals through AI focuses on answers rather than processes, limiting deeper conceptual grasp
Alternative Approaches to Teaching Children
For Spelling:
- Phonics-based instruction: Teaching letter-sound relationships rather than memorisation
- Word families: Learning patterns across words with similar sounds (dog, log, fog)
- Multisensory approaches: Tracing letters in sand, using letter blocks, singing alphabet songs
- Contextual learning: Learning words through stories and meaningful contexts
For Mathematics:
- Manipulatives: Using physical objects like cubes, counters, or toys
- Visual models: Drawing pictures to represent quantities
- Real-world applications: Counting actual objects in the environment
- Game-based learning: Using board games or card games that involve counting
- Progressive abstraction: Moving from concrete objects to pictorial representations to abstract symbols
Comparing Alternative Perspectives on AI in Education
The article presents a strongly negative view of AI in education, but other perspectives exist:
Potential Benefits Not Addressed in the Article:
- Accessibility: AI can provide learning support for students with disabilities or in resource-limited settings
- Personalisation: AI could potentially customise learning experiences based on student needs and progress
- Feedback efficiency: AI could provide immediate feedback on certain types of practice exercises, allowing teachers to focus on deeper aspects of learning
- Higher-order thinking focus: When used appropriately, AI might free students from lower-level tasks to focus on more complex thinking
- Real-world preparation: Learning to effectively use AI tools may prepare students for a workforce where AI collaboration is increasingly common
Nuanced Middle Ground:
A more balanced perspective might acknowledge that:
- Age-appropriate use matters (what’s unsuitable for a 5-year-old may be beneficial for a college student)
- The educational context is crucial (using AI as a tutor vs. using it to avoid learning)
- Teacher guidance can transform how AI is integrated into learning
- AI might be beneficial for certain aspects of learning while detrimental to others
The “Skipping Steps” Argument: Feature or Bug?
There remains an interesting counterpoint about whether skipping steps is actually a feature that allows learners to focus on higher-level conceptualisation and idea connection.
Arguments for this perspective:
- AI could handle routine, mechanical aspects of learning, freeing cognitive resources for deeper thinking
- Modern education might need to shift focus from fact retention to synthesis and application
- Working with AI could develop new metacognitive skills around prompt crafting and output evaluation
But the article implicitly counters this view by arguing:
- Those “skipped steps” are not just mechanical but developmentally crucial
- Conceptualisation cannot be separated from foundational skill development
- Linking ideas requires a deep understanding of those ideas first
- The cognitive processes involved in “basic steps” build neural pathways necessary for more advanced thinking
The article emphasises that learning is not just about acquiring information but about developing the thinking processes themselves. The grandmother’s concern isn’t just that her granddaughter is getting answers from AI, but that she’s missing the developmental benefits that come from learning to spell through phonemic awareness or understanding numerical relationships through physical manipulation.
In essence, the article suggests that we can’t separate the destination (knowledge) from the journey (learning process) because the journey itself shapes how we think.
Generative AI as a Lever for Higher-Order Thinking: A Counter-Perspective
Beyond Calculation: AI as a Cognitive Extension
My alternate perspective raises a profound counterpoint to the article’s concerns: perhaps AI tools like Chatgpt aren’t simply shortcuts that bypass learning, but cognitive extensions that could transform how we engage with knowledge itself, similar to how calculators changed our relationship with computation.
From Literal to Metaphorical Thinking
The calculator comparison is apt and revealing. When calculators became widespread, many educators worried students would lose fundamental arithmetic skills. Yet calculators ultimately freed mental bandwidth for higher mathematical reasoning. Similarly, AI might free us from specific literal cognitive processes to enable more metaphorical thinking.
Consider how this might work:
- Cognitive offloading: By delegating fact-retrieval and basic synthesis to AI, humans might gain cognitive space for pattern recognition across domains
- Abstraction acceleration: AI handling of literal meaning could push humans toward abstract thinking earlier in development
- Metaphor cultivation: When freed from the burden of literal processing, learners might more readily identify analogies and metaphorical relationships between concepts
Cross-Boundary Cognition
My Framing suggests AI might facilitate what could be called “cross-boundary cognition” across three dimensions:
Cross-Disciplinary Integration
- AI can rapidly synthesise information across traditionally siloed domains
- This could enable learners to see connections between, for example, historical events and mathematical patterns, or literary themes and scientific principles.
- Rather than mastering single domains sequentially, AI could help learners navigate the intersections between fields.s
Cross-Cultural Understanding
- AI’s ability to process vast cultural datasets might help learnerrecogniseze patterns and principles across diverse cultural contexts.
- This could foster thinking that transcends cultural specificity to identify universal human themes and divergent cultural solutions to similar problems.s
- Learners might develop meta-cultural awareness more readily when AI handles the translation of specific cultural references.
Cross-Historical Perspective
- AI can compress historical timelines and highlight evolutionary patterns in thought.
- This might enable learners to see themselves within longer historical arcs rather than isolated in their present moment.
- Historical thinking could become more intuitive when AI handles chronological detail.s
Toward Grand Narratives
The most compelling aspect of an alternate perspective is how AI might enable the construction of “grand narratives”—integrative frameworks that connect disparate knowledge into meaningful wholes. This contrasts sharply with the article’s focus on process-based, incremental learning.
AI might facilitate:
- Systems thinking: Seeing interconnections between seemingly unrelated domains
- Historical pattern recognition: Identifying recurring cycles and evolutionary trajectories
- Meaning-making across scales: Connecting individual experiences to broader social and historical movements
- Integrative knowledge frameworks: Building conceptual scaffolds thaorganiseze diverse information
A Dialectical Perspective
Perhaps the most productive approach is dialectical—recognising both the article’s valid concerns and your compelling counter-vision.
The tension might be resolved by understanding that:
- Developmental stages matter: Young children may indeed need concrete, embodied learning experiences before they can effectively leverage AI for higher-order thinking
- Cognitive foundations remain important: Even metaphorical thinking requires some grounding in literal understanding
- Different learning domains have different requirements: Mathematical reasoning may require more step-by-step foundation than cultural interpretation
- Meta-cognitive scaffolding is essential: Students need guidance in how to use AI as a tool for expanded thinking rather than a replacement for thinking
Implications for Educational Design
This perspective suggests a more nuanced educational approach where:
- Early education emphasises embodied, concrete learning experiences
- Middle education introduces AI as a tool with explicit teaching about its possibilities and limitations
- Higher education leverages AI to facilitate cross-boundary thinking and grand narrative construction
- Metacognitive training becomes central to the curriculum at all levels
Rather than seeing AI as either undermining traditional learning or revolutionising it, we might view it as creating a new cognitive landscape where both process-based understanding and integrative thinking have essential roles to play, with their relative importance shifting across developmental stages and learning domains.
The key question becomes not whether AI helps or hinders learning, but how educational systems can evolve to capitalise on AI’s capacity to facilitate higher-order thinking. At the same time, ensuring learners develop the necessary cognitive foundations to engage meaningfully with AI-generated content.
Patterns in the Digital Garden
Mei Lin stood at the window of her family’s 12th-floor HDB flat in Tampines, watching the late afternoon thunderstorm roll across Singapore. Lightning fractured the darkening sky as she absentmindedly traced equations on the foggy glass with her finger.
“Mei Lin! Dinner in five minutes!” her mother called from the kitchen.
“Coming, Ma,” she replied, but remained transfixed by the storm. At fifteen, Mei Lin had already established herself as Raffles Institution’s most promising mathematics student in a decade. Her teachers had run out of advanced problems to challenge her with, and she’d started auditing university courses at NUS on weekends.
But lately, she’d hit a wall. The problem that consumed her—finding novel patterns in prime number distributions—had been tackled by mathematicians for centuries. Despite her natural talent, she felt like she was merely retracing well-worn paths.
Her phone buzzed with a message from her best friend, Aisha.
Did you try what I suggested?
Mei Lin smiled. Unlike her other classmates, who used AI for homework shortcuts, Aisha had been encouraging Mei Lin to experiment with a different approach: not using AI to solve problems but to help visualize them in new ways.
Not yet, she typed back after dinner.
Later that evening, sitting cross-legged on her bed surrounded by notebooks filled with equations, Mei Lin opened her laptop and navigated to the custom AI interface she’d been building.
Unlike the standard commercial AI models her classmates used for essays, Mei Lin had been experimenting with fine-tuning an open-source model specifically for mathematical visualisation. She’d spent months feeding it mathematical literature, not to generate solutions, but to help her see patterns differently.
“Let’s try something new,” she murmured, typing her prompt carefully.
Visualise the first 10,000 prime numbers not as points on a number line, but as nodes in a three-dimensional space, where their position is determined by their relationship to the three prime numbers that precede them.
The AI began generating a complex 3d rendering. As it took shape on her screen, Mei Lin leaned forward, her breath catching. The visualisation was unlike anything she’d seen in her textbooks—primes weren’t just appearing as random points or following the expected spiral patterns, but forming something that looked almost organic, like the branching structure of neurons.
She adjusted her parameters, asking the AI to highlight specific relationships between clusters of primes. She wasn’t using the AI to solve anything; she was using it as a lens to see the mathematical landscape from perspectives human mathematicians hadn’t traditionally explored.
“That’s interesting,” she whispered, noticing a recurring pattern in one cluster. She quickly jotted down equations in her notebook, testing a hypothesis that was forming in her mind.
For the next three hours, Mei Lin worked in a flow state, switching between AI-generated visualisations and her own mathematical calculations. She wasn’t letting the AI do the mathematical thinking—she was using it to break her out of conventional ways of seeing the problem.
Mr. Tan, the head of Raffles’ mathematics department, looked sceptical as Mei Lin set up her presentation the following week.
“You’re saying you’ve found a new pattern in the distribution of certain prime clusters?” he asked, arms crossed. Behind him sat two professors from NUS who had agreed to review her work.
“Yes, sir,” Mei Lin said, connecting her laptop to the projector. “But first, I want to explain my methodology.”

She began by explaining how she had used AI—not to generate mathematical insights directly but to create unconventional visualizations that helped her see the problem differently. She was transparent about the technology’s role: a tool for perspective-shifting, not a replacement for mathematical reasoning.
“The breakthrough came when I noticed this,” she said, showing one of the 3d visualisations. “This cluster of primes seems random in traditional representations, but when viewed through this relational lens, a pattern emerges.”
She proceeded to walk through her discovery—a subtle but consistent pattern in how certain prime clusters related to each other, which had implications for number theory and potentially for cryptography.
The NUS professors exchanged glances. One of them, Professor Lim, leaned forward.
“This is… quite remarkable, Mei Lin,” she said carefully. “What gave you the idea to visualise primes in this particular three-dimensional relationship?”
Mei Lin smiled. “That’s where the AI helped most. I kept asking it to show me representations that were as far as possible from how humans typically visualise number relationships. I needed to break free from centuries of human mathematical intuition to see something new.”
Six months later, Mei Lin stood nervously in the auditorium of the National Science Challenge, her research displayed on posters behind her. Her paper, co-authored with Professor Lim, had been accepted to a prestigious mathematics journal, and she had been invited to present at conferences both in Singapore and abroad.
What had begun as an experiment with AI visualisations had evolved into a genuine mathematical contribution. The “Lin-Lim Pattern,” as some had begun calling it, offered new insights into prime number distribution that had potential applications in several fields.
Minister Chan, there to present the awards, stopped at her booth.
“So you used AI to make this discovery?” he asked, examining her visualisation.
“Not exactly, sir,” Mei Lin explained carefully. “I used AI as a tool to help me see patterns differently. The mathematical insight, the proof, and the implications—those all required human understanding.”
She gestured to her notebooks, filled with handwritten equations. “The AI helped me break out of conventional thinking, but understanding what I was seeing, proving it was significant, and connecting it to other areas of mathematics—that was all human work.”
The Minister nodded thoughtfully. “A partnership, then, rather than a replacement.”
“Exactly, sir,” Mei Lin agreed. “AI helped me see the mathematical forest in a new way, but I still had to identify which trees mattered and why.”
That evening, as Mei Lin’s family celebrated her first-place finish at the Challenge with a dinner at East Coast Park, her father raised his glass.
“To my brilliant daughter,” he said proudly, “who showed us all how to use technology wisely.”
Mei Lin smiled but shook her head. “Actually, Ba, I think what I showed was something simpler. New tools don’t replace thinking—they just give us new ways to think. The important part is still what happens here,” she tapped her temple, “when we use the tools to see beyond what others have seen before.”
Her phone buzzed with a message from Aisha: Congratulations! What are you going to visualise next?
Mei Lin looked up at the night sky, where stars were becoming visible above the city lights.
“I think,” she said slowly, “I’m going to look at the relationship between primes and quantum states. There’s something there I can’t quite see yet.”
She could already imagine the new perspectives AI visualisation might offer—not to find the answers for her, but to help her see the questions that had been invisible before.
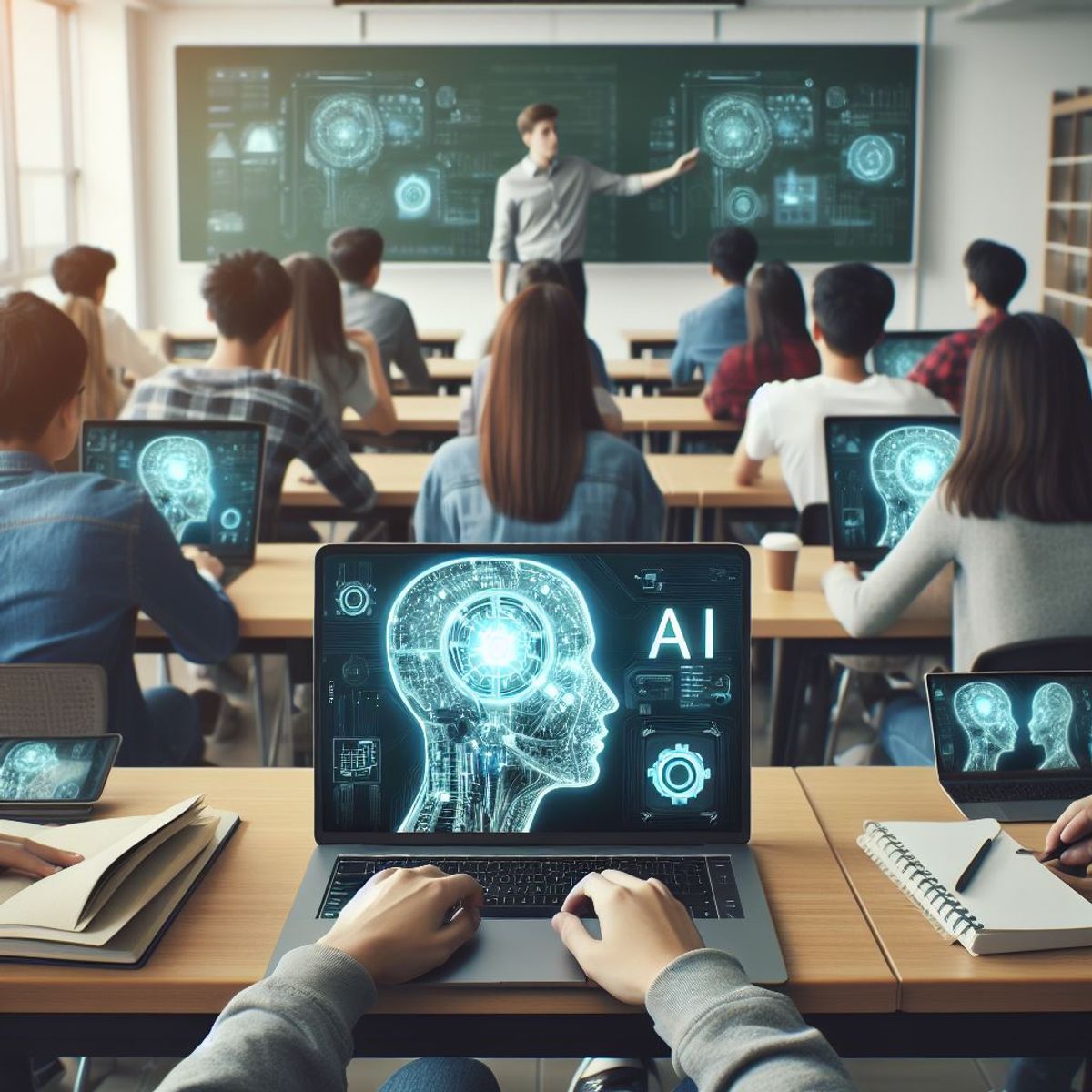
In her mind, mathematics unfolded like the Singapore skyline at night—human ingenuity and technological tools illuminated each other, creating something greater than either could alone.
AI as a Catalyst for Cross-Paradigm Thinking: Countering the Homogenization Concern
Beyond Singular Paradigms
The story of Mei Lin directly challenges one of the article’s core assertions: that AI tools lead to “a less diverse set of outcomes” and homogenised thinking. Instead, it illustrates how AI might function as a paradigm-crossing tool that expands cognitive diversity rather than constraining it.
Breaking Established Mental Models
Mei Lin’s breakthrough comes specifically from using AI to visualise mathematical relationships in ways human mathematicians hadn’t explored—”as far as possible from how humans typically visualise number relationships.” This represents a fundamentally different approach from the article’s fear that AI reinforces limited thinking patterns.
Key aspects of this cross-paradigm facilitation include:
- Epistemic boundary-crossing: The AI helps Mei Lin transcend traditional mathematical visualisations by generating representations unbound by human cognitive defaults
- Disciplinary hybridisation: Her approach merges computational visualisation with pure mathematics, creating a methodological hybrid
- Tradition disruption: Rather than accepting established mathematical visualisation approaches, she deliberately uses AI to break free from “centuries of human mathematical intuition”
Mechanics of Novel Thinking Generation
The story demonstrates several concrete mechanisms through which AI might foster genuinely novel thinking:
1. Representational Transformation
Mei Lin transforms abstract mathematical relationships into spatial-visual representations. This cognitive transposition—moving from symbolic mathematical notation to 3d visual patterns—allows her to perceive relationships invisible in traditional formats. The AI facilitates this by handling the computational complexity of generating these alternative representations.
2. Defamiliarisation
The AI helps Mei Lin reacquaint her with mathematical objects. By rendering the familiar (prime numbers) in unfamiliar ways (as 3d relational nodes), it creates cognitive dissonance that stimulates new thinking pathways. This process of making the familiar strange is a well-documented catalyst for creative breakthroughs.
3. Perceptual Extension
The story presents AI as extending human perceptual capabilities. Just as telescopes allowed astronomers to see celestial objects invisible to the naked eye, Mei Lin’s AI visualisations reveal mathematical patterns imperceptible through traditional analytical methods.
From Single-Path to Multi-Path Cognition
The article expressed concern that AI usage leads to less diverse thinking outcomes. The story suggests the opposite possibility:
Divergent Rather Than Convergent Thinking
Mei Lin doesn’t use AI to narrow down to a single correct answer (convergent thinking) but to open up multiple possible perspectives (divergent thinking). This directly contradicts the article’s concern about AI homogenising thought.
Cognitive Diversity Amplification
Rather than everyone arriving at similar AI-generated answers, the story suggests that personalised AI tools might amplify cognitive diversity by helping individuals explore unique intellectual territories aligned with their specific interests and abilities.
Metacognitive Development
Perhaps most significantly, the story shows Mei Lin developing enhanced metacognitive awareness—thinking about her own thinking process:
- She explicitly recognises the limitations of traditional human mathematical intuition
- She strategically designs AI prompts to overcome these limitations
- She maintains critical awareness of what the AI can and cannot contribute to her process
This metacognitive dimension represents a sophisticated level of thinking that goes beyond both traditional mathematical education and passive AI consumption.
A Different Technological Relationship
The story presents a fundamentally different human-AI relationship than the one feared in the article:
Active Direction vs. Passive Consumption
While the article portrays students passively accepting AI outputs, Mei Lin actively directs the AI as a tool. She maintains agency by:
- Designing specific visualisation parameters
- Interpreting the resulting patterns herself
- Using AI-generated visuals as starting points for her own mathematical exploration
Complementary Intelligence vs. Replacement
Rather than allowing AI to replace her thinking, Mei Lin cultivates a complementary relationship where:
- AI excels at computational visualisation and pattern generation
- Human intelligence excels at meaning-making, theoretical interpretation, and mathematical proof
Educational Implications
This alternative model suggests different educational possibilities than those presented in the article:
Teaching AI Literacy vs. Prohibition
Rather than discouraging AI use, educators might focus on teaching students to:
- Understand AI capabilities and limitations
- Design effective prompts that extend thinking rather than replace it
- Critically evaluate AI outputs
- Use AI to explore intellectual terrain beyond conventional boundaries
Cognitive Partnership Development
Education might explicitly foster skills for productive human-AI cognitive partnerships, including:
- Identifying which aspects of intellectual work to delegate vs. retain
- Developing metacognitive awareness of one’s own thinking processes
- Building capacity to translate between different representational systems
Beyond the Dichotomy
The story ultimately suggests moving beyond the false dichotomy of “AI vs. no AI” in education toward a more nuanced understanding of how different types of AI usage might either constrain or expand thinking.
Mei Lin’s approach—using AI as a perceptual tool rather than an answer generator—represents a sophisticated middle path that leverages AI’s computational power while preserving and enhancing uniquely human cognitive abilities.
The critical factor appears to be not whether AI is used, but how it is used—as a replacement for thinking or as a catalyst for novel forms of thinking currently constrained by human cognitive limitations. This suggests that the educational concern should focus less on prohibiting AI use and more on cultivating wisdom in how it is incorporated into learning processes.
Maxthon
In an age where the digital world is in constant flux, and our interactions online are ever-evolving, the importance of prioritizing individuals as they navigate the expansive internet cannot be overstated. The myriad of elements that shape our online experiences calls for a thoughtful approach to selecting web browsers—one that places a premium on security and user privacy. Amidst the multitude of browsers vying for users’ loyalty, Maxthon emerges as a standout choice, providing a trustworthy solution to these pressing concerns, all without any cost to the user.
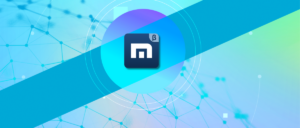
Maxthon, with its advanced features, boasts a comprehensive suite of built-in tools designed to enhance your online privacy. Among these tools are a highly effective ad blocker and a range of anti-tracking mechanisms, each meticulously crafted to fortify your digital sanctuary. This browser has carved out a niche for itself, particularly with its seamless compatibility with Windows 11, further solidifying its reputation in an increasingly competitive market.
In a crowded landscape of web browsers, Maxthon has forged a distinct identity through its unwavering dedication to offering a secure and private browsing experience. Fully aware of the myriad threats lurking in the vast expanse of cyberspace, Maxthon works tirelessly to safeguard your personal information. Utilizing state-of-the-art encryption technology, it ensures that your sensitive data remains protected and confidential throughout your online adventures.
What truly sets Maxthon apart is its commitment to enhancing user privacy during every moment spent online. Each feature of this browser has been meticulously designed with the user’s privacy in mind. Its powerful ad-blocking capabilities work diligently to eliminate unwanted advertisements, while its comprehensive anti-tracking measures effectively reduce the presence of invasive scripts that could disrupt your browsing enjoyment. As a result, users can traverse the web with newfound confidence and safety.
Moreover, Maxthon’s incognito mode provides an extra layer of security, granting users enhanced anonymity while engaging in their online pursuits. This specialized mode not only conceals your browsing habits but also ensures that your digital footprint remains minimal, allowing for an unobtrusive and liberating internet experience. With Maxthon as your ally in the digital realm, you can explore the vastness of the internet with peace of mind, knowing that your privacy is being prioritized every step of the way.