AI TRiSM Market Growth Forecast
The AI Trust, Risk and Security Management market is projected to experience significant growth:
- Current value (2024): $2.34 billion
- Projected value by 2030: $7.44 billion
- CAGR (Compound Annual Growth Rate): 21.60%
Key Market Drivers
- Accelerating AI adoption across industries
- Growing concerns around bias, explainability, and security vulnerabilities
- Increased regulatory focus on responsible AI systems
- Rise of generative AI foundation models creating new security challenges
- Industry collaborations expanding the AI TRiSM ecosystem
Market Segment Highlights
- Components: Solutions segment dominated with 70% of revenue in 2024; services segment expected to grow significantly
- Type: Explainability segment held the largest revenue share in 2024; ModelOps segment showing strong growth potential
- Application: Governance & compliance led in revenue share; bias detection & mitigation segment poised for substantial growth
- Deployment: On-premises deployment captured the largest market share in 2024; the cloud segment is projected for significant growth
- Enterprise Size: Large enterprises accounted for the most significant revenue share; the SME segment is predicted to grow markedly
- Industry: The IT & telecom sector led the market in 2024; the healthcare sector is expected to experience substantial growth
This report highlights how organisations are increasingly investing in tools to ensure their AI systems are trustworthy, secure, and compliant with regulations, particularly as generative AI foundation models create new challenges for data exchange and processing.
AI Trust, Risk and Security Management: Impact on Singapore Banks
Overview of AI TRiSM in Banking
AI Trust, Risk, and Security Management (TRiSM) represents a critical framework for Singapore’s banking sector as it increasingly adopts AI technologies. According to the market report, TRiSM encompasses several key components designed to ensure AI systems are trustworthy, secure, and compliant.
Core Components of AI TRiSM Relevant to Singapore Banks
1. AI Explainability
Singapore banks must ensure their AI systems’ decisions are transparent and explainable, particularly for:
- Credit scoring algorithms
- Fraud detection systems
- Investment recommendations
- Customer service chatbots
This aligns with the Monetary Authority of Singapore’s (MAS) emphasis on transparency in financial services.
2. ModelOps
For Singapore banks, robust ModelOps practices enable:
- Consistent monitoring of AI model performance
- Regular updates to adapt to changing financial conditions
- Proper governance throughout the AI model lifecycle
- Compliance with evolving regulations
3. Governance & Compliance
Particularly critical in Singapore’s highly regulated banking environment:
- Ensuring AI systems adhere to MAS guidelines
- Maintaining compliance with Singapore’s Personal Data Protection Act (PDPA)
- Alignment with international standards like GDPR for global operations
- Documentation of AI decision-making processes
4. Bias Detection & Mitigation
Vital for Singapore’s multicultural context:
- Ensuring fair lending practices across ethnic groups
- Preventing discriminatory financial services
- Maintaining equitable access to banking products
- Regular auditing for potential algorithmic bias
Singapore’s Regulatory Landscape for AI in Banking
Singapore’s approach to AI regulation in banking is particularly relevant to TRiSM implementation:
- MAS AI Guidelines: The Monetary Authority of Singapore has established principles for the responsible use of AI, emphasising fairness, ethics, accountability, and transparency (FEAT).
- Model Governance Framework: MAS provides guidance on effective governance practices for AI deployment in financial institutions.
- Risk Management Requirements: Singapore banks must demonstrate robust risk management processes for AI systems, including regular assessments and controls.
- Cross-Border Considerations: As a global financial hub, Singapore banks must navigate both local and international AI regulations.
Impact on Singapore Banks
Strategic Implications
- Competitive Advantage
- Banks with strong AI TRiSM practices can differentiate themselves in Singapore’s competitive financial market..
- Enhanced customer trust through demonstrable AI safeguards
- Ability to deploy advanced AI capabilities with confidence
- Operational Considerations
- Implementation costs for comprehensive TRiSM solutions
- Need specialised talent in AI governance and risk management
- Integration challenges with legacy banking systems
- Balancing innovation with security requirements
- Risk Mitigation
- Protection against reputational damage from AI failures
- Reduced regulatory penalties by ensuring compliance
- Defence against emerging AI-specific cyber threats
- Mitigation of financial losses from algorithmic errors
Implementation Challenges
- Technical Complexity
- Integration with existing banking security infrastructure
- Real-time monitoring requirements for high-volume transactions
- Model versioning and updating processes
- Regulatory Navigation
- Keeping pace with rapidly evolving AI governance frameworks
- Balancing global standards with Singapore-specific requirements
- Documentation burden for compliance demonstration
- Talent Gap
- Shortage of specialised AI risk management professionals in Singapore
- Training requirements for existing compliance teams
- Need for cross-functional expertise spanning technology and finance
Recommendations for Singapore Banks
- Phased Implementation Approach
- Begin with high-risk AI applications (credit, fraud detection)
- Establish governance frameworks before expanding AI deployment
- Implement continuous monitoring systems
- Cross-Industry Collaboration
- Partner with Singapore FinTech firms specialising in AI governance
- Participate in industry working groups on AI standards
- Share non-competitive insights on TRiSM best practices
- Regulatory Engagement
- Proactive dialogue with MAS on AI risk management expectations
- Participation in regulatory sandboxes for innovative AI applications
- Regular updates to compliance programs as guidelines evolve
- Talent Development
- Investment in specialised AI governance training programs
- Development of cross-functional teams spanning IT and compliance
- Knowledge transfer initiatives with global banking partners
Future Outlook
As the AI TRiSM market grows at a CAGR of 21.60% globally, Singapore banks are positioned to be early adopters given the country’s advanced technology infrastructure and strong regulatory framework. By 2030, the integration of AI TRiSM into banking operations will likely become a standard practice rather than a competitive differentiator.
The rise of generative AI in banking will accelerate the need for sophisticated TRiSM solutions. These technologies introduce novel risks related to content generation, information accuracy, and potentially unforeseen algorithmic behaviours that require specialised monitoring and governance approaches.
A Comprehensive Review of AI TRiSM in Singapore’s Banking Sector
Executive Summary
As Singapore continues to position itself as a global financial technology hub, artificial intelligence (AI) has become increasingly integral to the operations of its banking sector. With this technological evolution comes the critical need for AI Trust, Risk, and Security Management (TRiSM) frameworks. This review examines the current state of AI TRiSM implementation across Singapore’s banking landscape, its impact on major financial institutions, and the challenges and opportunities that lie ahead as the global AI TRiSM market grows from USD 2.34 billion in 2024 to a projected USD 7.44 billion by 2030.
Understanding AI TRiSM in Banking
Definition and Core Components
AI TRiSM encompasses a set of methodologies, tools, and practices designed to ensure that AI systems operate reliably, securely, and in compliance with regulatory requirements. In the banking context, this framework consists of several interconnected components:
- AI Explainability: Mechanisms that make AI decision-making processes transparent and interpretable, crucial for credit decisions and investment recommendations.
- ModelOps: Systematic approaches to AI model development, deployment, monitoring, and maintenance throughout their lifecycle.
- Governance & Compliance: Frameworks ensuring AI systems adhere to regulatory requirements, ethical standards, and organisational policies.
- Bias Detection & Mitigation: Tools and methodologies identifying and addressing algorithmic biases that could lead to discriminatory outcomes.
- AI Security: Measures protecting AI systems from adversarial attacks, data poisoning, and other emerging threats.
Regulatory Context in Singapore
Singapore’s financial sector operates under sophisticated regulatory oversight that has evolved to address AI-specific challenges:
- MAS FEAT Principles: The Monetary Authority of Singapore’s Fairness, Ethics, Accountability, and Transparency principles provide foundational guidance for responsible AI use in financial services.
- Technology Risk Management Guidelines: Updated in 2021, these guidelines include specific provisions for AI governance and risk management.
- Personal Data Protection Act (PDPA) governs the collection, use, and disclosure of personal data, with significant implications for AI systems processing customer information.
- AI Governance Framework: Singapore’s national AI strategy includes a governance framework that financial institutions are encouraged to adopt.
AI TRiSM Implementation Across Singapore’s Banking Sector
DBS Bank
As Singapore’s largest bank, DBS has taken a leadership position in AI adoption and corresponding TRiSM implementation:
- Integrated AI Governance Structure: DBS has established a dedicated AI Ethics Committee reporting directly to the Board Risk Management Committee, creating clear accountability for AI oversight.
- Explainable AI Initiative: The bank has invested significantly in developing explainable AI tools, particularly for credit decision-making systems. Their proprietary “AI Rationalisation Engine” provides customers and regulators with understandable explanations for AI-driven decisions.
- Model Risk Management Framework: DBS employs a comprehensive framework for AI model validation, including stress testing methodologies specifically designed for deep learning algorithms.
- Bias Monitoring System: The bank has implemented ongoing monitoring systems that analyse AI outcomes across different demographic segments to detect potential biases.
- Security Posture: DBS has integrated AI-specific security measures into its broader cybersecurity framework, including protection against model poisoning attacks.
OCBC Bank
OCBC has developed a nuanced approach to AI TRiSM with strengths in specific areas as:
- Federated AI Governance: Rather than centralising AI oversight, OCBC has implemented a federated governance model where business units maintain responsibility for their AI systems within an overarching framework.
- Natural Language Explainability: The bank has focused particularly on making natural language processing systems transparent, which is essential for its extensive deployment of conversational AI.
- Third-Party Validation: OCBC regularly engages external auditors specialising in AI ethics and security to review its systems.
- Collaborative Development: The bank has partnered with local universities to develop bias detection methodologies customised for Singapore’s multicultural context.
- Customer Transparency Initiative: OCBC has pioneered customer-facing explanations of AI use in banking services, enhancing trust through transparency.
United Overseas Bank (UOB)
UOB has taken a methodical approach to AI TRiSM:
- Risk-Based AI Governance: UOB employs a tiered governance structure where the level of oversight corresponds to the risk level of the AI application.
- Strong ModelOps Focus: The bank has invested heavily in automated model monitoring capabilities that continuously evaluate AI performance.
- Regional Adaptation Framework: UOB has developed protocols for adapting its AI systems and corresponding TRiSM controls across different Southeast Asian markets.
- AI Documentation Standards: The bank maintains comprehensive documentation of AI systems, including training data provenance, model architectures, and validation methodologies.
- Regulatory Technology Integration: UOB’s AI TRiSM approach incorporates regulatory technology (RegTech) solutions that automate compliance monitoring.
Smaller Financial Institutions and Digital Banks
Singapore’s smaller banks and digital challengers present varying levels of AI TRiSM maturity:
- Digital Banks (Trust Bank, GXS Bank): These institutions have built AI TRiSM capabilities from scratch, often with more modern approaches but less comprehensive frameworks than established banks.
- International Banks with Singapore Operations: Institutions like Citibank and Standard Chartered implement global AI TRiSM standards with local adaptations to meet MAS requirements.
- Smaller Local Banks: These institutions typically leverage vendor solutions for AI TRiSM rather than developing in-house capabilities, creating potential standardisation benefits but also dependencies.
Technical Implementation Challenges
Singapore banks face several technical challenges in implementing comprehensive AI TRiSM:
- Balancing Explainability and Performance: Banks struggle to maintain high performance while ensuring explainability, particularly with advanced deep learning models.
- Legacy System Integration: Many AI TRiSM tools must interface with legacy banking infrastructure not designed for modern AI applications.
- Real-Time Monitoring Capabilities: Implementing continuous monitoring for high-volume transaction systems presents technical and computational challenges.
- Multi-Model Interactions: As banks deploy multiple AI systems that interact with each other, TRiSM complexity increases exponentially.
- Federated Learning Security: Newer approaches like federated learning (where models are trained across multiple devices) create novel security challenges.
Operational and Strategic Impact
The implementation of AI TRiSM has had significant implications for Singapore banks:
Operational Impacts
- Extended Development Timelines: According to industry sources, the need for robust TRiSM has extended AI project timelines by an estimated 30-40%.
- Modified Deployment Processes: Banks have implemented phased deployment approaches with expanded monitoring during initial release periods.
- Enhanced Documentation Requirements: AI projects now generate substantially more documentation related to risk, testing, and validation.
- Cross-Functional Teams: AI development now typically involves risk, compliance, and security professionals from the outset rather than as later reviewers.
- Increased Computing Resource Requirements: TRiSM processes, particularly for continuous monitoring and explainability, require additional computational resources.
Strategic Impacts
- Risk Appetite Adjustment: Singapore banks have recalibrated their risk appetites for AI innovation, with more precise boundaries for acceptable use cases.
- Competitive Differentiation: Some banks now market their AI governance practices as trust differentiators to consumers and business clients.
- Vendor Management Changes: Banks have developed specialised assessment frameworks for AI vendors, with TRiSM capabilities becoming key selection criteria.
- Talent Acquisition Focus: Financial institutions have created specialised roles focused on AI ethics, security, and governance.
- Innovation Partnership Models: Several banks have established partnerships with TRiSM technology providers rather than building all capabilities in-house.
Market Dynamics and Economic Implications
The growth of AI TRiSM in Singapore’s banking sector has created significant market impacts:
- Vendor Ecosystem Growth: A specialised ecosystem of AI governance and risk management vendors has emerged to serve the banking sector.
- Cost Structures: Banks report that TRiSM-related activities now constitute approximately 15-25% of overall AI project budgets.
- Insurance Market Development: New insurance products specifically covering AI risks have emerged, with premiums influenced by the robustness of TRiSM practices.
- Regulatory Technology Growth: The RegTech segment focused on AI compliance has seen particular growth within Singapore’s fintech ecosystem.
- International Standards Influence: Singapore’s approach to AI TRiSM in banking is increasingly influencing international standards development.
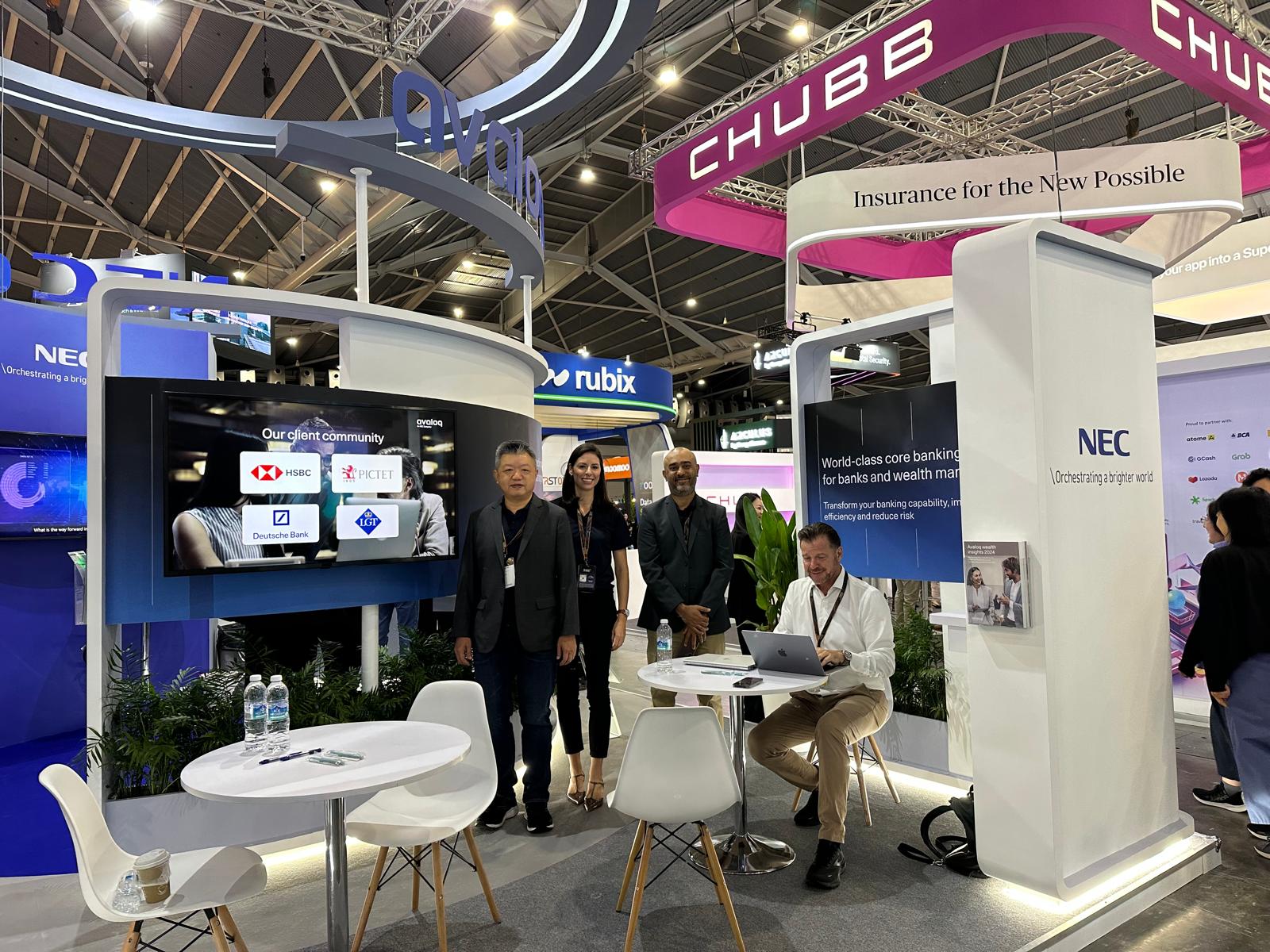
Emerging Trends and Future Directions
Several emerging trends will shape the future of AI TRiSM in Singapore’s banking sector:
- Automated TRiSM: Development of AI systems that automatically monitor other AI systems for risks and vulnerabilities.
- Standardised Audit Frameworks: Movement toward standardised approaches for auditing AI systems in financial services.
- Quantum-Resistant Security: As quantum computing develops, banks are beginning to consider quantum-resistant security measures for AI systems.
- Cross-Border TRiSM Harmonisation: Singapore banks are working toward harmonised TRiSM practices across their international operations.
- Consumer Control Mechanisms: Development of interfaces giving customers greater visibility and control over AI use in their banking relationships.
- Generative AI Governance: Specialised frameworks for managing risks associated with generative AI applications in banking.
Recommendations for Stakeholders
For Banking Institutions
- Develop AI TRiSM Centres of Excellence: Establish dedicated teams with specialised expertise in AI governance and risk management.
- Implement Tiered Governance Approaches: Tailor governance intensity to the risk level and impact potential of different AI applications.
- Invest in Automated Monitoring: Deploy continuous monitoring solutions that can scale with increasing AI deployment.
- Foster Cultural Integration: Ensure TRiSM principles are embedded in organisational culture, not just formal processes.
- Engage with Regulatory Development: Participate actively in the development of AI governance standards and regulatory frameworks.
For Regulators
- Develop Clear Assessment Frameworks: Provide clear guidance on how AI TRiSM will be evaluated during regulatory examinations.
- Support Standardisation Efforts: Facilitate industry standardisation of AI risk classification and documentation approaches.
- Enable Regulatory Sandboxes: Create safe spaces for testing innovative AI applications with adapted TRiSM requirements.
- Invest in Supervisory Technology: Develop technical capabilities to effectively supervise AI systems in banking.
- International Harmonisation: Work toward international coordination of AI governance requirements to reduce compliance complexity.
For Technology Providers
- Develop Integrated Solutions: Create TRiSM tools that integrate smoothly with existing banking technology stacks.
- Focus on Automation: Emphasize automation of routine TRiSM processes to improve efficiency and consistency.
- Support Industry Standards: Align product development with emerging industry standards for AI governance.
- Provide Implementation Support: Recognise the complexity of TRiSM implementation and provide appropriate support services.
- Develop Sector-Specific Solutions: Create tools tailored to banking-specific AI applications and regulatory requirements.
Conclusion
As Singapore’s banking sector continues its AI transformation, the importance of robust Trust, Risk, and Security Management cannot be overstated. The current implementations across major banks demonstrate varying approaches and maturity levels, but a clear trend toward comprehensive governance frameworks is evident. The projected growth of the global AI TRiSM market to USD 7.44 billion by 2030 reflects the increasing recognition that effective AI deployment requires sophisticated risk management capabilities.
Singapore’s position as both a financial hub and technology leader creates a unique opportunity to establish global best practices in AI TRiSM for banking. The investment required is substantial, but the potential benefits in terms of trustworthy AI systems, regulatory compliance, and customer confidence make a compelling business case for continued development in this area.
As generative AI and other advanced technologies further transform banking operations, the sophistication of TRiSM approaches will need to evolve in parallel. The banks that establish robust, efficient, and adaptable AI governance frameworks today will be best positioned to leverage AI innovations securely in the future, maintaining Singapore’s reputation for financial stability while embracing technological advancement.
A Day in the Life: AI TRiSM Through a Singaporean’s Eyes
Morning Revelations
Lim Wei Ming sipped his kopi as rain pattered against the windows of his Tanjong Pagar apartment. The notification on his phone interrupted his morning ritual: “Unusual account activity detected. Please verify recent transactions.” As DBS Bank’s Assistant Vice President of AI Governance, he found it ironic to be on the receiving end of the very systems he helped design.
The alert wasn’t from his personal account but from TransTrust, the monitoring platform he’d spent the past eighteen months developing. Just two days ago, it had flagged anomalies in the credit scoring model deployed across DBS’s minor business loan division.
“So much for a quiet Wednesday,” Wei Ming muttered, placing his half-finished breakfast in the sink.
By 8:15 AM, he was navigating through the morning crowd at Raffles Place MRT station. The financial district loomed above as bankers, analysts, and technologists converged on Singapore’s economic heart. Wei Ming’s role bridged these worlds—technical enough to understand the complex AI systems powering modern banking, but with sufficient risk management expertise to anticipate problems before they materialised.
The Morning Challenge
“The model’s rejecting applications it should approve,” explained Priya, the data scientist who’d raised the initial alert. They sat in a glass-walled meeting room on the 23rd floor of DBS Asia Central. “The bias detection module flagged it—approval rates for businesses in Geylang dropped by 22% overnight.”
Wei Ming frowned. “Just Geylang? Not other districts?”
“That’s what makes it unusual,” Priya replied, bringing up a visualisation on her tablet. “The geographic distribution shows a clear boundary effect.”
This was precisely the type of scenario Singapore’s financial regulators had warned about: algorithmic bias manifesting in ways that could disadvantage specific communities. The Monetary Authority of Singapore’s AI Ethics Committee would expect a thorough explanation, especially given DBS’s position as a leading financial institution.
“Let’s run a parallel analysis against the pre-deployment test cases,” Wei Ming suggested. “And get Mohammad from Model Risk Management involved. This could be a drift issue with the new data pipeline.”
The morning dissolved into a flurry of technical investigations, stakeholder communications, and risk assessments. By noon, they’d identified the source: a recent update to Singapore’s Urban Redevelopment Authority zoning classifications had been incorporated into the model, but with inconsistent historical mapping. The AI system had interpreted the change as increased risk rather than a simple administrative reclassification.
Lunchtime Reflections
Over chicken rice at a nearby hawker centre, Wei Ming met with his former university classmate, Sarah Chen, who is now working in UOB’s AI Ethics Office.
“So you caught it before any actual discrimination occurred?” Sarah asked, mixing chilli sauce into her rice.
Wei Ming nodded. “TransTrust flagged it within hours of deployment. But it makes me think about all the models running without this level of oversight.”
“That’s what keeps me up at night,” Sarah admitted. “UOB’s approach is different from yours—we’ve implemented a three-tiered governance structure based on risk levels. But the challenge is the same: how do you monitor systems that make thousands of decisions per minute?”
Their conversation shifted to the broader implications of AI in Singapore’s financial landscape. Both had witnessed the transformation firsthand—from simple rule-based systems to sophisticated machine learning algorithms that could assess creditworthiness, detect fraud, and personalise financial advice.
“My ah ma still doesn’t trust ATMs, let alone AI,” Wei Ming laughed. “But she’s using DBS PayLah! Without realising there’s machine learning behind it.”
“That’s the paradox,” Sarah replied. “The best AI feels invisible until something goes wrong. Then suddenly everyone wants explanations we can’t always provide.”
Afternoon Diplomacy
Back at DBS Asia Central, Wei Ming’s afternoon was consumed by the diplomatically delicate task of communicating the model issue to internal and external stakeholders. A video call with the bank’s Chief Risk Officer was followed by a carefully worded email to the MAS supervisory team.
“We’re applying the fix now,” Wei Ming explained to the CRO. “The model will be retrained with the corrected zone classifications, and we’ll run enhanced fairness testing before redeployment.”
“And what about affected customers?” the CRO asked.
“Twenty-three applications were incorrectly scored. We’ve flagged them for manual review, and our remediation team will contact each applicant directly.”
The conversation shifted to broader governance questions. “MAS has been asking about our model documentation standards,” the CRO noted. “This incident actually demonstrates the strength of our framework. Make sure that comes through in your report.”
After the call, Wei Ming met with his team to implement the remediation plan. The technical fix was straightforward, but rebuilding confidence would take more effort. Singapore’s banking sector operated on trust, both from customers and regulators, and AI systems added new dimensions of complexity to maintaining that trust.
Evening Connections
As evening approached, Wei Ming headed to a Singapore Fintech Association event at the newly completed Keppel Bay Innovation Hub. The networking session featured a panel discussion on “AI Governance in Financial Services: Singapore’s Approach.”
One of thepanellistss was Dr. Ng, his former professor from Nanyang Technological University, who now advises MAS on technology policy.
“Singapore has an opportunity to set global standards for AI governance in banking,” Dr. Ng was saying as Wei Ming slipped into a seat at the back. “Our approach balances innovation with prudence—we’re not trying to eliminate all risk, but to manage it appropriately.”
During the Q&A session, a representative from one of the digital banks challenged this view: “Traditional governance frameworks are too slow for AI development cycles. By the time you’ve documented and tested everything according to MAS guidelines, your competitors have launched three new features.”
Dr. Ng smiled. “Speed and safety aren’t mutually exclusive. The most innovative banks are building governance into their development processes, not treating it as a separate compliance exercise.”
Wei Ming nodded in recognition. This was precisely what he’d been working toward at DBS—integrating TRiSM into the development lifecycle rather than applying it afterwards.
After the panel, he caught up with Dr. Ng over Tiger beers.
“I heard about your incident today,” Dr. Ng said. “Good catch.”
Wei Ming raised an eyebrow. “News travels fast.”
“This is Singapore,” Dr. Ng laughed. “Financial news travels faster than the MRT.”
Their conversation turned to the future of AI governance. “The next frontier is cross-border harmonisation,” Dr. Ng explained. “A Singaporean bank operating in Thailand, Indonesia, and China faces four different regulatory approaches to AI. That’s not sustainable.”
Night Reflections
When Wei Ming returned to his apartment, it was nearly 10 PM. The rain had stopped, leaving the city glistening under the night lights. He opened his laptop one last time to check the status of the model fix. The test results looked promising—the geographic bias had been eliminated, and the system was ready for review before tomorrow’s redeployment.
His phone buzzed with a message from his mother: “Ah, boy, can you help me check why my POSB account is showing a funny message? Is it something about AI checking my transactions? Is my money safe?”
Wei Ming smiled at the irony. As AI became embedded in everyday banking, his professional and personal worlds collided more frequently. He called her immediately.
“Ma, it’s just the bank’s new security system,” he explained. “It’s actually keeping your money safer by looking for unusual patterns.”
“How does the computer know what is unusual for me?” she asked skeptically.
Wei Ming paused, considering how to explain neural networks and behavioural analytics to someone who still preferred passbooks to mobile banking.
“It learns from what you normally do,” he said finally. “Like how you knew I was sick last year just because I didn’t call on Sunday as usual. The system looks for changes in patterns.”
There was silence on the line. “So computer like mother now, is it?” she finally said with a laugh. “Next time computer will call and ask if you are eating properly!”
After the call, Wei Ming stood by his window, looking out at Singapore’s skyline. Tomorrow would bring new challenges—another model to validate, more regulations to interpret, and the endless balance between innovation and caution.
But tonight’s conversation with his mother had crystallised something important. Behind all the technical frameworks, governance committees, and regulatory guidelines was a simple truth: AI TRiSM was fundamentally about trust. Whether it was his mother trusting her bank, small business owners trusting loan decisions, or regulators trusting financial institutions, the human element remained central.
As Singapore’s banking sector continued its AI transformation, Wei Ming reflected that success would depend not just on sophisticated algorithms and monitoring systems but also on maintaining that delicate, human quality of trust—something no algorithm could generate on its own.
AI Trust and Security in Singapore’s Banking Sector: Institution-Specific Analysis and MAS Impact
Introduction
Singapore’s position as Asia’s premier financial hub has been reinforced by its aggressive adoption of artificial intelligence technologies across its banking sector. As AI systems increasingly influence critical financial functions—from credit decision-making to fraud detection and customer service—the importance of robust Trust, Risk, and Security Management (TRiSM) frameworks has moved to the forefront of both institutional strategy and regulatory concern. This review provides a detailed analysis of AI trust and security approaches across Singapore’s major banking institutions. It examines the reciprocal impact on the Monetary Authority of Singapore (MAS) as the nation’s financial regulator.
In the context of Singapore’s Smart Nation initiative and its vision to become a global AI governance leader, the banking sector serves as a crucial testing ground for balancing innovation with prudent risk management. With the global AI TRiSM market projected to grow from USD 2.34 billion in 2024 to USD 7.44 billion by 2030 (21.60% CAGR), Singapore banks are making significant investments in this domain, creating distinctive approaches that reflect their unique market positions, technical capabilities, and risk appetites.
Institution-Specific Analysis
DBS Bank: Pioneer in Integrated AI Governance
As Singapore’s largest bank and a self-proclaimed “technology company with a banking license,” DBS has established itself as a frontrunner in both AI adoption and corresponding governance frameworks.
Trust and Explainability Infrastructure
DBS has developed a proprietary AI Governance Framework that centres on what the bank terms “Responsible AI by Design.” This framework incorporates:
- Explainable AI Platform: DBS’s proprietary “xAI Hub” provides standardised explainability tools across the organisation. The platform uses both model-agnostic techniques (such as SHAP values and LIME) and model-specific interpretability methods to generate explanations for AI-driven decisions. Critically, these explanations are calibrated to different audiences—from technical documentation for regulators to simplified interfaces for customers questioning loan rejections.
- Transparency Gradients: DBS employs a novel approach of “transparency gradients” where the level of explanation provided scales with the potential impact of the AI decision. Low-impact recommendations (such as personalised marketing) receive streamlined explanations, while high-impact decisions (like credit denials) trigger comprehensive justifications.
- Ethical AI Committee: This cross-functional governance body, comprising senior representatives from technology, risk management, compliance, and business units, meets bi-weekly to review high-risk AI applications and resolve emerging ethical questions.
Security Architecture and Threat Management
DBS’s AI security architecture is built on a defence-in-depth strategy tailored explicitly for AI systems:
- Model Protection Framework: The bank has implemented advanced safeguards against adversarial attacks, including input validation layers, anomaly detection systems monitoring model inputs, and redundant systems for critical functions.
- Dedicated AI Red Team: DBS maintains a specialised security team focused solely on probing and testing AI vulnerabilities. This team regularly conducts adversarial testing exercises, including data poisoning attempts, input manipulation, and model extraction attacks.
- AI Supply Chain Security: Recognising the risks in AI development pipelines, DBS has implemented a comprehensive vendor assessment framework for third-party AI components, with regular security audits and contractual provisions for security standards.
Notable Incidents and Responses
DBS’s mature AI governance framework has been tested by several incidents:
- In late 2023, the bank’s fraud detection system generated a spike in false positives affecting approximately 2,300 customers. DBS’s response demonstrated its governance maturity—the issue was publicly disclosed within 24 hours, affected customers were proactively contacted, and a root cause analysis revealed a data drift issue that had bypassed standard monitoring. The bank subsequently enhanced its drift detection capabilities.
- In a move toward greater transparency, DBS published its first “Responsible AI Report” in early 2024, disclosing key metrics including model accuracy rates, fairness assessments across demographic groups, and the number of human overrides of AI decisions.
Regulatory Engagement
DBS maintains particularly close engagement with MAS on AI governance, participating in multiple regulatory sandboxes focused on explainable AI. The bank’s Chief Data Officer sits on MAS’s AI Ethics Advisory Council, contributing to the development of industry-wide standards.
OCBC: Focus on Data-Centric AI Security
OCBC has pursued a distinctive approach to AI trust and security, emphasising data governance as the foundation of trustworthy AI.
Trust and Explainability Infrastructure
OCBC’s approach to AI trust centres on its “AI Confidence Framework” with several notable elements:
- Data Provenance System: OCBC has invested heavily in data lineage and provenance tracking infrastructure that documents the entire journey of data used in AI training and inference. This system allows the bank to trace any potential biases or issues to specific data sources, enhancing accountability.
- Scenario-Based Explainability: Rather than focusing on technical interpretability methods, OCBC has developed a library of counterfactual explanations for common customer scenarios. These pre-validated explanations allow customer-facing staff to provide consistent and accurate information about AI decisions.
- Confidence Scoring: Every AI output is accompanied by a calibrated confidence score visible to internal users, allowing human operators to apply appropriate scepticism to AI recommendations.
Security Architecture and Threat Management
OCBC’s security approach for AI systems emphasises:
- Secure Training Environment: The bank has created an isolated, air-gapped infrastructure for training sensitive AI models, with comprehensive monitoring and access controls.
- Privacy-Preserving Techniques: OCBC has been a relatively early adopter of differential privacy and federated learning approaches, which enhance security by minimising the exposure of raw customer data during AI development.
- Automated Security Testing Pipeline: The bank has developed automated testing processes that scan AI models for common vulnerabilities before deployment approval.
Notable Initiatives and Challenges
OCBC has faced several challenges in its AI TRiSM journey:
- In mid-2024, the bank encountered significant issues when attempting to deploy its first large language model for customer service applications. Initial fairness assessments revealed concerning disparities in response quality across different Singaporean English dialects. The bank delayed deployment by four months to address these issues through targeted data augmentation and bias mitigation techniques.
- OCBC has been remarkably transparent about its model documentation standards, publishing templates and examples that have subsequently been referenced by MAS in its guidelines for AI governance in financial services.
Regulatory Engagement
OCBC actively participates in MAS’s AI Veritas initiative, focusing particularly on fairness metrics and assessment methodologies for credit scoring algorithms. The bank has advocated for standardised approaches to fairness measurement across the industry to create consistency in compliance expectations.
UOB: Decentralized Governance with Centralized Oversight
UOB has implemented a distinctive governance approach that balances business unit autonomy with enterprise-wide standards.
Trust and Explainability Infrastructure
UOB’s AI governance model includes:
- Federated Responsibility Model: Unlike the centralised approaches at DBS and OCBC, UOB has implemented a federated responsibility model where individual business units maintain primary responsibility for AI governance, operating within an enterprise framework of standards and controls.
- Three-Tier Validation System: UOB employs a rigorous validation approach where high-risk AI systems undergo reviews by three separate entities: the developing team, an independent model validation group, and external third-party assessors.
- Customer-Facing Explainability Portal: UOB has developed a dedicated customer portal allowing clients to view explanations of AI-influenced decisions affecting their accounts, enhancing transparency and trust.
Security Architecture and Threat Management
UOB’s security approach for AI systems includes several distinctive elements:
- Component-Level Risk Ratings: The bank has implemented a granular security approach where individual components of AI systems (data pipelines, model architectures, deployment infrastructure) receive separate risk ratings and corresponding security controls.
- Dynamic Security Perimeters: UOB employs adaptive security boundaries for AI systems based on real-time risk assessments, with automated scaling of monitoring intensity and access restrictions.
- AI-Specific Incident Response Plans: The bank has developed dedicated incident response protocols for AI-related issues, with specialised teams explicitly trained for scenarios like model misbehaviour,, data leakage, and adversarial attacks.
Notable Innovations and Incidents
UOB has demonstrated several innovative approaches to AI TRiSM:
- The bank pioneered a “Customer AI Impact Statement” that accompanies new AI-driven services, clearly explaining to customers how AI influences the service, what data is used, and what options customers have for human intervention.
- UOB faced challenges in early 2024 when its wealth management recommendation system demonstrated unexpected behaviour after a routine update. The issue was traced to a subtle interaction between two independently validated model components. In response, the bank enhanced its testing protocols to include integration testing between model components.
Regulatory Engagement
UOB has focused its regulatory engagement on operational aspects of AI governance, contributing technical expertise to MAS working groups on model inventory management, monitoring standards, and documentation requirements. The bank has advocated for principles-based regulation that allows for methodological diversity while maintaining strong governance outcomes.
Citibank Singapore: Global Standards with Local Adaptation
As an international bank with significant operations in Singapore, Citibank presents an interesting case study in adapting global AI governance frameworks to local regulatory expectations.
Trust and Explainability Infrastructure
Citibank’s approach includes:
- Global Standards with Local Overlays: The bank maintains a global AI governance framework that sets minimum standards, supplemented by Singapore-specific controls aligned with MAS expectations. This creates a “regulation-plus” environment where Singapore operations often exceed global requirements.
- Centralised Model Repository: Citibank maintains a comprehensive inventory of all AI models deployed in Singapore, with standardised documentation including model cards, data dictionaries, and validation reports accessible to both internal reviewers and regulators.
- Multi-Layered Review Process: AI applications undergo sequential reviews by technology, risk, compliance, legal, and business teams before deployment approval, with escalation paths for resolving conflicting priorities.
Security Architecture and Threat Management
Citibank’s security approach for AI systems reflects its global cybersecurity infrastructure with local enhancements:
- 24/7 AI BehaviouralMonitoring: The bank has implemented continuous monitoring of AI outputs aagainst expected behavioural parameters, with automated alerts for pattern deviations.
- Cross-Border Data Security: Given its international operations, Citibank has developed sophisticated controls for secure cross-border data transfers supporting AI operations, including encryptitokenisationtion, and jurisdictional localisation where required.
- Adversarial Robustness Testing: The bank routinely tests AI systems against sophisticated adversarial techniques, leveraging its global security resources.
Notable Approach to Transparency
Citibank has taken distinctive approaches to transparency and stakeholder engagement:
- The bank publishes quarterly “AI Governance Updates” for institutional clients, sharing anonymised insights from its governance activities and emerging best practices. These publications have become reference points for the broader industry.
- Citibank has implemented gradual rollouts for new AI capabilities, with explicit testing periods communicated to customers and structured feedback collection.
Regulatory Engagement
Citibank actively participates in MAS’s international collaboration initiatives on AI governance, leveraging its global presence to facilitate dialogue between Singapore regulators and their counterparts in other major financial centres. This has contributed to greater alignment between Singapore’s approach and emerging global standards.
Digital Challengers: Trust-First AI Development
Singapore’s digital banks and fintech challengers have approached AI TRiSM from a different starting point compared to established institutions.
Trust by Design Approaches
Digital challengers like Trust Bank and GXS Bank have embraced “trust by design” principles:
- Built-In Explainability: Without legacy systems to integrate, these institutions have built explainability capabilities directly into their core platforms rather than adding them retrospectively.
- Customer-Controlled AI: Digital banks have pioneered approaches allowing customers to set their own parameters for AI personalisation, providing transparency sliders that adjust the influence of various data points on recommendations and decisions.
- Open Model Documentation: Several fintech players have adopted open approaches to model documentation, publishing technical whitepapers describing their algorithms (with appropriate security redactions) to build trust through transparency.
Security Challenges and Solutions
Digital challengers face distinct security challenges:
- Resource Constraints: With smaller security teams than established banks, digital challengers have embraced automated security testing and continuous monitoring to compensate for limited human resources.
- Cloud-Native Security: Operating primarily on cloud infrastructure, these institutions have developed cloud-specific security controls for their AI operations, including enhanced container security, infrastructure-as-code security scanning, and API protection layers.
- Rapid Response Mechanisms: Digital challengers have implemented streamlined incident response protocols that prioritise speed, allowing for rapid model rollbacks and customer communication during incidents.
Regulatory Navigation
New entrants face unique regulatory challenges:
- Digital challengers must demonstrate governance maturity despite their relatively short operational histories. To satisfy regulatory expectations. To satisfy regulatory expectations, they often require more detailed documentation and testing than established players.
- Several digital banks have pursued formal certifications such as ISO/IEC 42001 (AI Management System Standard) to provide independent validation of their governance frameworks.
MAS’s Evolving Role and Impact
Regulatory Framework Development
The Monetary Authority of Singapore has developed a sophisticated and evolving approach to AI governance in banking:
- Principles-Based with Prescriptive Elements: MAS has maintained a primarily principles-based regulatory stance while introducing increasingly specific expectations in high-risk domains like credit decision-making and fraud detection.
- Fairness, Ethics, Accountability and Transparency (FEAT) Principles: These foundational principles continue to guide MAS’s approach, with ongoing refinements to implementation guidance based on industry feedback and international developments.
- Technology Risk Management Guidelines: MAS updated these guidelines in 2021 to incorporate AI-specific controls, creating clearer expectations for the security and operational resilience of AI systems.
- Regular Thematic Inspections: Since 2023, MAS has conducted regular thematic inspections explicitly focused on AI governance, targeting different segments of the banking sector in rotation.
Impact of Singapore Banks’ Practices on MAS
The evolving practices of Singapore banks have influenced MAS’s regulatory approach in several ways:
- Feedback Loop for Standards Development: Innovative practices at DBS, OCBC, and UOB have informed subsequent regulatory guidance, creating a virtuous cycle where bank innovation shapes regulatory expectations.
- Tiered Supervisory Framework: Based on observed variations in AI maturity across institutions, MAS has adopted a risk-based supervisory approach where supervisory intensity scales with both the risk of AI applications and the sophistication of bank governance.
- Examination Methodology Evolution: MAS has developed increasingly sophisticated examination approaches for AI systems, moving beyond documentation reviews to include technical testing, model validation sampling, and scenario-based challenges.
- Regional Leadership Role: Singapore’s experience has positioned MAS as a thought leader in AI governance across ASEAN, with several neighbouring regulators adopting frameworks influenced by the Singapore approach.
International Engagement and Standards Development
MAS has actively engaged with international bodies on AI governance:
- Basel Committee on Banking Supervision: MAS has contributed significantly to the Basel Committee’s work on AI risk management, bringing perspectives from Singapore’s implementation experience.
- Financial Stability Board: MAS representatives have participated in FSB working groups developing principles for AI use in financial services, advocating for approaches that balance innovation with stability.
- Bilateral Cooperation Agreements: MAS has established bilateral cooperation agreements on AI governance withkeywith keyrs,rs inc ling the UK’s Financial Conduct Authority, the Hong Kong Monetary Authority, and the European Banking Authority.
Areas of Ongoing Development
MAS continues to develop its approach in several areas:
- Generative AI Governance: In response to the rapid proliferation of generative AI applications in banking, MAS is developing specific guidance addressing unique risks like hallucination, prompt injection, and copyright concerns.
- Quantitative Fairness Standards: MAS is working toward more specific guidance on measuring and mitigating algorithmic bias, potentially including statistical thresholds for acceptable disparities across demographic groups.
- Consolidated AI Risk Rating Methodology: The regulator is developing a standardised methodology for assessing aggregate AI risk across an institution, similar to the CAMELS rating system for overall bank soundness.
- AI Incident Reporting Framework: MAS is enhancing requirements for reporting significant AI incidents, with more precise definitions of reportable events and standardised information requirements.
Impact on Singapore’s Banking Sector
Competitive Dynamics
The evolution of AI TRiSM in Singapore has influenced competitive dynamics in several ways:
- Trust as Differentiation: Banks with more mature AI governance frameworks have begun marketing this as a competitive advantage, particularly for corporate and institutional clients with sophisticated risk management expectations.
- Innovation Speed vs. Governance Rigour: Different risk appetites for AI deployment have created noticeable variation in innovation speed, with some institutions prioritising rapid deployment while others emphasising governance thoroughness.
- Talent Competition: The specialised skills required for AI governance have created intense competition for qualified professionals, with banks increasingly developing internal talent through structured development programs.
- Ecosystem Development: A vibrant vendor ecosystem has emerged around AI TRiSM, with Singapore becoming a hub for regtech startups specialising in model risk management, explainability tools, and automated testing.
Operational Impacts
The implementation of comprehensive AI TRiSM has had significant operational impacts:
- Extended Development Timelines: Banks report that robust governance requirements have extended AI project timelines by 30-50% compared to pre-governance baselines, though this gap is narrowing as processes mature.
- Resource Allocation: Major Singapore banks now allocate 15-25% of their AI budgets specifically to governance, risk, and security activities, representing a significant investment.
- Organisational Restructuring: Most institutions have created dedicated AI governance teams, typically positioned either within enterprise risk management functions or as independent units reporting directly to senior management.
- Process Standardisation: Initial ad hoc approaches to AI governance have evolved into standardised processes integrated with existing risk management frameworks, creating consistency across different business units.
Client and Public Trust
Enhanced AI TRiSM practices have influenced public perception in notable ways:
- Transparency Initiatives: Several banks have launched customer-facing initiatives explaining their use of AI in everyday banking services, moving beyond regulatory compliance to build active trust.
- Education Programs: Recognising varying levels of AI literacy among customers, banks have developed education initiatives ranging from simple explanatory materials to interactive tools demonstrating how AI influences banking services.
- Trust Metrics: Some institutions have begun measuring customer trust in AI-driven services as a key performance indicator, directing governance resources toward areas with lower trust ratings.
Future Directions and Recommendations
Emerging Trends
Several trends are likely to shape the future of AI TRiSM in Singapore banking:
- Automated Governance: The development of AI systems that automatically monitor other AI systems for compliance, bias, and security vulnerabilities—sometimes called “AI for AI Governance”—is accelerating, with several banks piloting such capabilities.
- Continuous Validation: Moving beyond point-in-time model validations, banks are implementing continuous validation frameworks that assess models throughout their lifecycle, with dynamic adjustments to monitoring intensity based on performance.
- Regulatory Technology Integration: Deeper integration between bank governance platforms and regulatory supervision systems is emerging, potentially enabling more real-time regulatory visibility into AI operations.
- Cross-Border Harmony: With most major Singapore banks operating across Southeast Asia, efforts to harmonise AI governance approaches across different regulatory jurisdictions are gaining momentum.
Recommendations for Financial Institutions
Singapore banks should consider several strategic priorities for AI TRiSM:
- Governance Automation: Invest in automating routine governance tasks to improve efficiency while allowing human experts to focus on complex risk assessment and ethical questions.
- Skills Development: Build comprehensive training programs for both technical teams and business stakeholders to create broader organisational capability for identifying and managing AI risks.
- Graduated Controls: Implement tiered governance frameworks that apply control intensity proportionate to risk, optimising resource allocation while maintaining appropriate oversight.
- Cross-Institutional Collaboration: Participate in industry consortia and knowledge-sharing platforms to develop common approaches to shared challenges, such as fairness metrics and security standards.
Recommendations for MAS
To further enhance the regulatory framework for AI in banking, MAS should consider:
- Harmonised Metrics: Develop standardised metrics for key aspects of AI governance, particularly around fairness, model performance, and explainability, to enable consistent assessment across institutions.
- Supervisory Technology: Invest in supervisory technology capabilities that enable more efficient and effective oversight of increasingly complex AI systems.
- Principles-Based Core with Prescriptive Elements: Maintain a principles-based foundational framework while developing more prescriptive guidance for the highest-risk applications and use cases.
- International Leadership: Continue to advance international dialogue on AI governance for financial services, leveraging Singapore’s experience to influence global standards development.
Conclusion
Singapore’s banking sector has established itself as a global leader in AI trust, risk, and security management, developing sophisticated approaches that balance innovation with prudent risk management. The diversity of approaches across institutions—from DBS’s centralised governance to UOB’s federated model and the “built-from-scratch” approaches of digital challengers—creates a rich ecosystem for experimentation and learning.
As AI technologies continue to evolve, particularly with the rapid advancement of generative AI and foundation models, both banks and regulators will need to adapt their approaches. The fundamental tensions between innovation speed, governance thoroughness, and operational efficiency will persist, requiring ongoing calibration of controls and oversight.
MAS has established a regulatory approach that has gained international recognition for its balance of principles-based foundations with increasingly specific expectations in high-risk domains. This approach has positioned Singapore as an influential voice in global discussions on AI governance for financial services.
For Singapore to maintain its leadership position in this domain, continued investment in governance capabilities, talent development, and international engagement will be essential. The next phase of development will likely focus on greater automation of governance processes, more sophisticated approaches to measuring fairness and bias, and deeper integration of governance considerations throughout the AI development lifecycle.
As the global AI TRiSM market grows to its projected USD 7.44 billion by 2030, Singapore’s banking sector is well-positioned to remain at the forefront of balancing AI innovation with trustworthy, secure implementation, serving as a model for financial institutions worldwide navigating similar challenges.
In-Depth Analysis of Singapore Banks’ Security Measures Against Mobile Wallet Fraud
Current Security Landscape and New Measures
Singapore’s banking sector is implementing progressive security measures to combat the rising threat of mobile wallet fraud. The recent announcements by DBS, POSB, UOB, and OCBC highlight a significant shift in the security architecture:
DBS/POSB Approach
- Default-Off Toggle: Implementation of a “mobile wallets” toggle in their banking app that remains off by default
- Time-Limited Authorization: Users have only a 10-minute window to add cards after enabling the toggle
- Automatic Deactivation: The system automatically reverts to secure mode after the time window expires
- Money Lock Tool: Previously launched feature that prevents specific funds from being transferred digitally
UOB and OCBC Approach
- In-App Authentication: Moving away from SMS OTPS to in-app digital token authentication by July 2025
- Proactive Monitoring: OCBC has been removing suspicious cards linked to multiple wallets since 2023
- SMS OTP Elimination: A Strategic move to phase out the vulnerable SMS OTP verification system
Technical Security Evolution
These measures represent an evolution in security thinking from several perspectives:
- Moving from Reactive to Proactive: Banks are shifting from responding to fraud after detection to preventing the possibility of unauthorized access
- Zero Trust Architecture: The implementation reflects zero trust principles, where no action is authorized by default
- Time-Based Security: The 10-minute window introduces temporal constraints that significantly reduce the attack window for criminals
- Authentication Layer Enhancement: Moving from single-factor (SMS OTP) to more secure authentication methods addresses a critical vulnerability
Impact on Singapore’s Banking Sector
Positive Impacts
- Fraud Reduction: These measures directly address the reported 650+ cases and $1.2 million in losses from Q4 2024
- Consumer Confidence: Enhanced security builds trust in digital payment systems
- Setting Regional Standards: Singapore continues to establish itself as a leader in financial security innovation in Southeast Asia.
- Education Effect: The “deliberate pause” mentioned by DBS executives serves an educational purpose, conditioning users to be more security-conscious
Potential Challenges
- User Friction: Additional security steps may increase transaction friction and potentially frustrate some users
- Digital Divide: Less tech-savvy customers might struggle with new security features
- Adaptation Period: Both banks and customers will need time to adjust to new authentication workflows
- Competitive Pressure: Banks must balance security with user experience to maintain a competitive advantage
Long-Term Implications for Singapore’s Financial Ecosystem
- Regulatory Influence: The Success of these measures may influence the Monetary Authority of Singapore (MAS) guidelines
- Cross-Industry Standards: Could establish new security norms across the entire financial services industry
- Regional Leadership: May position Singapore as a blueprint for other ASEAN nations facing similar threats
- Security Infrastructure Investment: Likely to drive increased investment in security technologies across the sector
- Fraud Displacement: Criminals may shift tactics to target other vulnerabilities, potentially requiring additional security enhancements
- Consumer Behaviour Shift: May condition Singaporean consumers to expect and prefer higher security standards
The orchestrated approach across multiple banks suggests coordinated Action within Singapore’s financial sector, possibly with regulatory guidance. This systemic response demonstrates Singapore’s continued commitment to maintaining its reputation as one of the world’s most secure financial hubs while adapting to evolving digital payment landscapes.
POSB’s Comprehensive Approach to Tackling Mobile Wallet Fraud
Core Security Innovation: The Mobile Wallet Toggle
POSB (in conjunction with parent company DBS) has implemented a groundbreaking security mechanism specifically designed to combat the rising threat of mobile wallet fraud. At the heart of this approach is a seemingly simple but strategically significant feature: the mobile wallet toggle switch within their banking application.
Technical Implementation Details
- Default Security Posture: The toggle is engineered to remain “off” by default, establishing a secure baseline state where card details cannot be added to any mobile wallet.
- Deliberate User Action Requirement: Users must consciously locate and activate this toggle within the banking app interface before attempting to add their card to services like Apple Pay, Google Pay, or Samsung Pay.
- Time-Bound Authorisation Window: Once activated, the system implements a strict 10-minute authorization window during which card details can be added to a mobile wallet.
- Automatic Security Restoration: After this 10-minute period elapses, the toggle automatically reverts to its “off” position, re-establishing the secure baseline state without requiring additional user action.
- Integration with Existing Security Infrastructure: This toggle works in conjunction with POSB’s broader authentication ecosystem, adding an additional proprietary security layer.
Strategic Security Principles Embedded in the Approach
Attack Vector Disruption
The toggle mechanism directly interrupts the typical fraud sequence where criminals obtain card details and SMS one-time passwords (OTPS) through phishing attacks. Even with both pieces of information, fraudsters would face a new barrier: the need to access and activate the toggle within the victim’s authenticated banking application.
Time Constraint as a Security Feature
The 10-minute window introduces a critical temporal security dimension. This narrow timeframe:
- Minimise the opportunity window for unauthorised actions
- Forces potential attackers to coordinate multiple breach attempts simultaneously
- Creates a sense of urgency that heightens user vigilance during the sensitive operation
Psychological Security Design
POSB’s approach leverages psychological principles to enhance security:
- The requirement for Actionable Action increases user awareness
- The time constraint introduces a “security moment” where users are likely to be more attentive
- The automatic deactivation removes the cognitive burden of remembering to re-secure the account
Defense-in-Depth Strategy
This toggle represents one component of POSB’s multi-layered defence architecture:
- Authentication Layer: Initial bank app login security (typically biometric or password-based)
- Authorisation Layer: The toggle mechanism for specific high-risk actions
- Time-Constraint Layer: The 10-minute window limitation
- Automatic Reset Layer: The system-initiated return to the secure state
Operational Implementation Considerations
Customer Education and Adoption
POSB faces the challenge of effectively communicating this security enhancement to its diverse customer base. The bank must balance:
- Clear communication about the new process
- Educational resources explaining the security benefits
- Assistance channels for less tech-savvy customers
- Guidance for customers encountering issues during the transition
Integration with Merchant Ecosystem
The toggle approach requires coordination with the broader payment ecosystem, including:
- Mobile wallet providers (Apple, Google, Samsung)
- Payment processors
- Merchants accepting contactless payments
- Regulatory bodies overseeing payment security standards
Technical Infrastructure Requirements
Supporting this security feature necessitates robust backend systems:
- Real-time toggle state tracking
- Precise timing mechanisms
- Synchronization between the banking app and the card provisioning systems
- Reliable performance across various mobile devices and operating systems
Comparative Advantages Over Previous Approaches
SMS OTP Vulnerability Mitigation
Prior to this implementation, the primary security mechanism for adding cards to mobile wallets relied heavily on SMS one-time passwords, which had several fundamental weaknesses:
- Susceptibility to SIM swapping attacks
- Vulnerability to phishing attempts
- Interception possibilities through malware
- Social engineering vulnerabilities
The toggle approach significantly reduces reliance on this vulnerable channel by adding an independent security gate within POSB’s controlled ecosystem.
Progression Beyond Static Security Models
Traditional banking security often relied on static protective measures:
- Fixed passwords
- Knowledge-based authentication questions
- Card verification values (CVV)
POSB’s approach represents a shift toward dynamic security components that incorporate time constraints and deliberate user actions as security elements.
Future Evolution Potential
Integration with Biometric Verification
The toggle mechanism could potentially be enhanced with:
- Facial recognition confirmation before activation
- Fingerprint verification as an additional authentication factor
- Behavioural biometrics to detect unusual activation patterns
Machine Learning Enhancement
Future iterations might incorporate AI capabilities:
- Anomaly detection for unusual toggle activation patterns
- Risk scoring based on device, location, and behavioural factors
- Adaptive security measures based on calculated risk levels
Customizable Security Profiles
POSB might eventually allow customers to personalize aspects of this security feature:
- Adjustable time windows based on personal risk tolerance
- Pre-author Pre-authorized devices for streamlined experiences
- Scheduled activation periods for planned shopping sessions
Impact on POSB’s Broader Security Ecosystem
This mobile wallet toggle represents one component of POSB’s comprehensive approach to security, complementing other existing measures:
- Money Lock Tool: Previously implemented feature allowing customers to designate funds that cannot be accessed for digital transfers
- Transaction Monitoring Systems: AI-powered systems that analyze to detect suspicious activities
- Customer Education Initiatives: Ongoing programs to increase security awareness among customers
- Notification Systems: Real-time alerts for sensitive account activities
- ScamShield Helpline Integration: Direct access to specialized support through the 1799 helpline
Challenges and Considerations for the Future
Despite its innovative approach, POSB’s toggle mechanism faces several challenges:
User Experience Balance
The additional security step introduces friction into the mobile wallet setup process. POSB must continuously evaluate and refine the balance between security and convenience to maintain customer satisfaction.
Fraud Evolution Response
As with any security measure, fraudsters will likely adapt their tactics. POSB must monitor emerging threat patterns and evolve this mechanism accordingly.
Technical Reliability Requirements
The toggle functionality must maintain near-perfect uptime and performance, as issues could significantly impact customer experience during the critical 10-minute window.
Cross-Platform Consistency
Ensuring consistent implementation across ios, Android, and other potential platforms presents ongoing technical challenges.
Conclusion: A Pioneering Approach in Singapore’s Banking Security Landscape
POSB’s mobile wallet toggle represents a significant advancement in banking security design for Singapore. By incorporating deliberate user action, time constraints, and automatic security restoration, the bank has created a multidimensional mechanism that specifically targets the vulnerabilities exploited in mobile wallet fraud schemes.
The approach demonstrates how seemingly simple interface changes, when strategically implemented with security principles at their core, can substantially enhance protection against sophisticated fraud attempts. As part of Singapore’s broader banking security evolution, this measure positions POSB at the forefront of customer-focused security innovation.
Comprehensive Analysis: How POSB/DBS’s Toggle Mechanism Prevents Mobile Wallet Fraud
The Anatomy of Mobile Wallet Fraud Before the Toggle
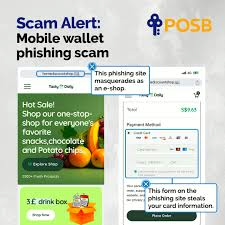
To understand how the toggle mechanism prevents fraud, we must first examine the typical attack sequence that fraudsters employed before this security measure:
- Card Detail Acquisition: Criminals obtained card information through:
- Phishing websites mimic bank login pages
- Data breaches exposing card details
- Skimming devices at ATMS or point-of-sale terminals
- Social engineering tactics
- OTP Interception: Perpetrators gained access to one-time passwords via:
- SMS phishing (smishing) attacks
- SIM swapping to redirect authentication messages
- Malware that intercepted SMS messages
- Social engineering to trick victims into sharing OTPS
- Mobile Wallet Addition: With both card details and OTPS, criminals could:
- Add victims’ cards to their own mobile wallets
- Make contactless payments at merchants
- Purchase goods online using the tokenised card
- Cokenizeduct transactions without physical possession of the card
- Exploitation Window: Victims often remained unaware until:
- Reviewing statements days or weeks later
- Receiving delayed fraud alerts
- Noticing unusual account activity
The Toggle’s Multidimensional Security Mechanisms
The toggle introduces several protective layers that disrupt this attack chain:
1. Authentication Domain Separation
Traditional vulnerability: Previously, SMS OTPS represented a single, vulnerable verification channel outside the bank’s direct control.
Toggle solution: The verification process requires action Action the authenticated banking app environment, which:
- Creates domain separation between verification channels
- Requires attackers to compromise both the mobile banking app and the OTP channel
- Establishes a “two-domain” verification requirement
2. Default-Secure Architecture
Traditional vulnerability: Card addition capability was perpetually enabled by default, requiring no preliminary security action.
Toggle solution: The default-off state means:
- The system assumes a secure posture unless explicitly changed
- No action can occur without deliberate user intervention
- The system maintains a “closed by default” security stance
3. Temporal Security Window
Traditional vulnerability: Once credentials were compromised, attackers had unlimited time to exploit them.
Toggle solution: The 10-minute activation window:
- Forces attackers to operate within a highly narrow timeframe
- Requires synchronized attack vectors simultaneously
- Creates a time-pressure element that increases the likelihood of detection
- Automatically closes the vulnerability without requiring user vigilance
4. Attack Synchronisation Barrier
Traditional vulnerability: Attackers could methodically execute different stages of their attack over extended periods.
Toggle solution: Successful fraud now requires synchronizing:
- Banking app credentials/biometrics
- The toggle activation capability
- Card details
- Any additional verification methods
- All within the same 10-minute window
5. Physical Device Separation
Traditional vulnerability: Remote attackers could add cards to mobile wallets without physical access to any of the victim’s devices.
Toggle solution: The toggle requires:
- Physical access to the victim’s authenticated banking app
- Authorize the device’s own security mechanisms (fingerprint, facial recognition, PIN)
- Creating a physical possession barrier to purely remote attacks
Security Effectiveness Against Common Attack Vectors
Against Phishing Attacks
Pre-toggle vulnerability: Phishers could collect card details and OTPS through fake websites or messages.
Toggle protection mechanism: Even with these credentials, attackers cannot proceed without:
- Access to the victim’s authenticated banking app
- Knowledge that the toggle exists and must be activated
- Ability to activate the toggle within the victim’s secure app environment
Effectiveness rating: Very High – Phishing alone is insufficient without a banking app compromise.
Against Social Engineering
Pre-toggle vulnerability: Criminals could manipulate victims into revealing all necessary credentials.
Toggle protection mechanism: Social engineering becomes significantly more complex as attackers must now:
- Guide victims through a multi-step process within their banking app
- Maintain control throughout the entire 10-minute window
- Overcome the victim’s potential suspicion of being guided to toggle security features
Effectiveness rating: High – Social engineering becomes more complicated and suspicious.
Against Malware
Pre-toggle vulnerability: Malware could harvest credentials and intercept OTPS automatically.
Toggle protection mechanism: Malware would now need to:
- Gain sufficient privileges to interact with the banking app interface
- Identify and activate the toggle programmatically
- Complete the mobile wallet addition within the time window
- All while remaining undetected by the app’s security measures
Effectiveness rating: Moderate to High – Depends on malware sophistication and banking app security.
Against Insider Threats
Pre-toggle vulnerability: Insiders with access to banking systems could potentially extract card details.
Toggle protection mechanism: Internal access to card data is insufficient, as the toggle:
- Requires an authenticated device
- Cannot be bypassed through backend systems alone
- Creates an auditable action trail specific to each customer
Effectiveness rating: High – Significantly reduces insider threat capability.
Technical Implementation Aspects Enhancing Security
API-Level Protection
The toggle likely implements protection at the API level, where:
- Tokenization requests are rejected unless the toggle state is verified
- Digital wallet provisioning servers check the toggle status before processing
- Authorisation servers Authorisation ta oggle state in their decision matrix
Cryptographic Binding
The toggle status is likely cryptographically bound to:
- The specific user account
- The authenticated session
- A timestamp indicating activation time
- Creating a secure, tamper-evident authorisation token
Authorisation enhancement
The toggle creates valuable forensic evidence:
- Toggle activation timestamps
- Device identification data
- Session correlation information
- Geographical metadata at the time of activation
Risk-Based Analysis of Remaining Vulnerabilities
Despite its effectiveness, some attack vectors remain, albeit with increased difficulty:
Sophisticated Mobile Banking App Compromise
Scenario: Advanced malware specifically designed to:
- Operate with banking app privileges
- Manipulate the toggle interface programmatically
- Complete wallet addition automatically
Risk level: Low – Requires highly sophisticated, targeted malware and multiple security bypasses.
Social Engineering Combined with Remote Access
Scenario: Attacker gains remote access to the victim’s device and:
- Guides the victim to activate the toggle while maintaining control
- Quickly adds a card to the attacker’s wallet during the window
Risk level: Low to Moderate – Requires coordinated attack and victim cooperation.
Account Takeover via Banking App Credentials
Scenario: Complete compromise of banking credentials allowing an attacker to:
- Log in to the banking app directly
- Activate the toggle independently
- Add a card to a fraudulent wallet
Risk level: Moderate – Requires complete authentication compromise but remains technically feasible.
Ecosystem-Wide Security Enhancement
The toggle mechanism’s benefits extend beyond individual transaction security:
Fraud Intelligence Generation
The toggle creates new data points for fraud detection:
- Unusual toggle activation patterns
- Geographic discrepancies between toggle activation and card addition
- Time analysis between activation and wallet addition
- Frequency of toggle use compared to the customer baseline
Criminal Economics Disruption
The toggle significantly impacts fraud economics by:
- Increasing attack complexity and required resources
- Reducing success rates for automated attacks
- Narrowing the exploitation window substantially
- Forcing attackers to develop more sophisticated (and expensive) techniques
Industry Security Standard Evolution
This approach could influence broader payment security standards:
- Setting precedent for authorization requirements
- Demonstrating the effectiveness of time-limited security windows
- Showcasing customer-controlled security features
Quantifiable Security Improvements
While exact metrics would require proprietary data, we can reasonably project:
- Attack Success Rate Reduction: The toggle likely reduces successful fraud attempts by:
- Creating multiple new points of failure for attackers
- Increasing technical complexity beyond most fraudsters’ capabilities
- Introducing timing constraints that complicate coordination
- Attack Attempt Deflection: Many potential attackers will:
- Recognize the increased difficulty and abandon attempts
- Target banks without such measures instead
- Be forced to attempt more visible and detectable approaches
- Fraud Financial Impact: The $1.2 million in reported losses from Q4 2024 should see a significant reduction as:
- High-volume automated attacks become nearly impossible
- Manual attacks require substantially more resources per attempt
- Detection rates increase through toggle-related anomaly identification
Conclusion: A Transformative Security Paradigm
The mobile wallet toggle represents more than just an incremental security improvement—it fundamentally alters the security architecture of mobile payment systems in Singapore. By implementing a default-off, time-limited, authentication-domain-separated security mechanism, POSB/DBS has creatmultidimensionalional security control specifically targeting the vulnerabilities inherent in mobile wallet provisioning.
This approach moves beyond traditional reactive security measures to establish a proactive security posture that prevents fraud at its inception point. The toggle interface’s elegant simplicity belies the sophisticated security principles embedded within it, demonstrating how thoughtful security design can significantly enhance protection without introducing excessive friction into the customer experience.
As mobile payments continue to grow in Singapore’s increasingly cashless economy, this toggle mechanism provides a robust foundation for secure digital transactions that could serve as a model for financial institutions worldwide.
How the Mobile Wallet Toggle Directly Stops Fraud: A Straightforward Explanation
The Core Problem the Toggle Solves
Before understanding how the toggle stops fraud, it’s essential to identify precisely what problem it addresses:
The previous vulnerability: Scammers who obtained your card details and SMS one-time password (OTP) could add your card to their own mobile phones without you knowing. They could then make purchases using your card through their mobile wallets.
How the Toggle Mechanism Works to Stop This
The toggle introduces a critical new step that directly prevents this type of fraud:
- Mandatory App Access: To add your card to ANY mobile wallet (including on someone else’s phone), the toggle must first be switched on in YOUR authenticated DBS/POSB banking app.
- Direct Physical Control: This toggle can be activated onlwho has someone with access to our phone and can log into your banking app.
- International Action Required: The toggle is OFF by default, meaning no one can add your card to a mobile wallet until you deliberately turn it on.
- Limited Time Window: Once activated, you have only 10 minutes to add the card before the toggle automatically switches off again.
Why This Effectively Blocks Fraudsters
Here’s how this directly stops the fraud in practical terms:
Scenario: A Scammer with Your Card Details and OTP
Without the toggle (old system):
- A scammer gets your card details through phishing
- A scammer gets your OTP through phishing or SMS interception
- A scammer immediately adds your card to their phone’s wallet
- A scammer starts making fraudulent purchases
- You only discover this when you check your statement later
With the toggle (new system):
- A scammer gets your card details through phishing
- A scammer gets your OTP through phishing or SMS interception
- Scammer attempts to add your card to their phone’s wallet
- BLOCKED: The system checks if the toggle is activated in your banking app
- Since the toggle is off by default, the addition fails
- The scammer cannot proceed without access to your physical phone and banking app credentials
- No fraudulent transactions occur
The Critical Security Break in the Attack Chain
The toggle creates a critical break in the fraud chain by:
- Requiring access to something the scammer doesn’t have – your physical phone with your authenticated banking app
- Moralization of authorization into a more secure environment – from vulnerable SMS to your protected banking app
- Creating a “default deny” security posture – nothing happens unless you actively permit it
- Limiting the potential exposure window – even if somehow compromised, the window closes automatically after 10 minutes
Real-World Protection Examples
Protection Against Phishing Attacks
- A scammer sends you a fake bank message asking for your card details and OTP
- Even if you fall for this and provide the information
- The scammer still cannot add your card to their wallet
- Because they cannot activate the toggle in your banking app
Protection Against Data Breaches
- Your card details are exposed in a merchant data breach
- A fraudster obtains these details and attempts to add your card to their wallet
- The attempt fails because the toggle in your banking app is off
- Your money remains safe despite the data breach
Protection Even If Your SMS is Compromised
- A scammer manages to intercept your SMS messages through a SIM swap or malware
- They capture an OTP sent by the bank
- They still cannot add your card to their wallet
- Because they cannot activate the toggle in your banking app
The Technical Security Chain
The toggle creates a multi-step security process that must be followed in exact sequence:
- User must authenticate into their banking app (requiring device access + biometrics/PIN)
- The user must locate and activate the toggle (requiring knowledge of the feature)
- The user must complete the card addition within 10 minutes (creating time pressure)
- Toggle automatically deactivating future unauthorized additions)
For a fraudster to bypass this, they would need simultaneous access to:
- Your physical phone
- Your banking app login credentials or biometrics
- Knowledge of how to use the toggle feature
- All within a narrow 10-minute window
Why This Is More Effective Than Previous Solutions
The toggle mechanism is particularly effective because:
- It’s simple: No complex technologies that users need to understand
- It’s under your direct control: You physically control when cards can be added
- It separates authentication channels: Even if one security channel (like SMS) is compromised, the fraudster still needs access to a separate channel (your banking app)
- It requires no ongoing vigilance: The default-off state means you’re protected without having to remember to do anything.
- It creates an unambiguous security checkpoint: Either the toggle is on or off, with no grey areas or ways to social engineer around it.
Conclusion: A Direct Block Against Mobile Wallet Fraud
The toggle acts as a simple but highly effective gatekeepeunauthorizedtly prevents unauthorised mobile wallet additions. By requiring physical access to your authenticated banking app before any card can be added to any mobile wallet, it creates a security barrier that most fraudsters cannot overcome.
This single feature directly addresses the specific vulnerability that led to over 650 fraud cases and $1.2 million in losses in late 2024, providing a straightforward but powerful protection mechanism for Singapore’s banking customers.
Maxthon
When it comes to staying safe online, using a secure and private browser is crucial. Such a browser can help protect your personal information and keep you safe from cyber threats. One option that offers these features is the Maxthon Browser, which is available for free. It comes with built-in AdBlock and anti-tracking software to enhance your browsing privacy.
Maxthon Browser is dedicated to providing a secure and private browsing experience for its users. With a strong focus on privacy and security, Maxthon implements rigorous measures to protect user data and online activities from potential threats. The browser utilises advanced encryption protocols to ensure that user information remains protected during internet sessions.
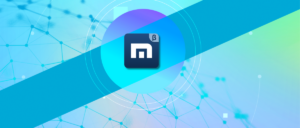
Additionally, Maxthon incorporates features such as ad blockers, anti-tracking tools, and incognito mode to enhance users’ privacy. By blocking unwanted ads and preventing tracking, the browser helps maintain a secure environment for online activities. Furthermore, incognito mode enables users to browse the web without leaving any trace of their history or activity on the device.
Maxthon’s commitment to prioritising the privacy and security of its users is exemplified through regular updates and security enhancements. These updates are designed to address emerging vulnerabilities and ensure that the browser maintains its reputation as a safe and reliable option for those seeking a private browsing experience. Overall, Maxthon Browser provides a comprehensive suite of tools and features designed to deliver a secure and private browsing experience.
Maxthon Browser, a free web browser, offers users a secure and private browsing experience through its built-in AdBlock and anti-tracking features. These features help to protect users from intrusive ads and prevent websites from tracking their online activities. The browser’s AdBlock functionality blocks annoying pop-ups and banners, allowing for an uninterrupted browsing session. Additionally, the anti-tracking software safeguards user privacy by preventing websites from collecting personal data without consent.
By utilising Maxthon Browser, users can browse the internet confidently, knowing that their online activities are shielded from prying eyes. The integrated security features alleviate concerns about potential privacy breaches, ensuring a safer browsing environment. Furthermore, the browser’s user-friendly interface makes it easy for individuals to customise their privacy settings according to their preferences.
Maxthon Browser not only delivers a seamless browsing experience but also prioritises the privacy and security of its users through its efficient ad-blocking and anti-tracking capabilities. With these protective measures in place, users can enjoy the internet with confidence, knowing their online privacy is protected.
Additionally, the desktop version of Maxthon Browser integrates seamlessly with their VPN, providing an extra layer of security. By using this browser, you can minimise the risk of encountering online threats and enjoy a safer internet experience. With its combination of security features, Maxthon Browser aims to provide users with peace of mind while they browse.
Maxthon Browser stands out as a reliable choice for users who prioritise privacy and security. With its robust encryption measures and extensive privacy settings, it offers a secure browsing experience that gives users peace of mind. The browser’s commitment to protecting user data and preventing unauthorised access sets it apart in the competitive market of web browsers.