The piece highlights several key concerns that major banks like JPMorgan and Citigroup are expressing to investors:
- Workforce impacts – AI could lead to “workforce displacement”, affecting employee morale and retention while also increasing competition for tech talent
- Cybersecurity threats – Banks face a balancing act between adopting AI to stay competitive while managing the increased cybercrime risks
- Data quality issues—There is concern about AI systems built on outdated, biased, or inaccurate financial data.
- AI hallucinations – Citigroup specifically mentions risks from analysts working with “ineffective, inadequate or faulty” AI-generated results.
The article emphasizes the crucial role of proper governance mechanisms for AI deployment, with one expert noting, “This is not a plug-and-play technology.”
I find it particularly interesting how financial institutions are navigating this double-edged sword—they need AI to remain competitive and meet customer expectations, but they must simultaneously manage significant risks to their operations, reputation, and security posture.
AI Risks in Banking and Their Solutions
Key AI Risks in Banking
1. Data Quality and Bias Issues
- Banking AI systems rely on historical financial data that may contain inherent biases.
- Poor quality or incomplete datasets can lead to discriminatory lending practices or inaccurate risk assessments.
- AI models may perpetuate existing inequalities in financial services.
2. Security and Cybercrime Vulnerabilities
- Advanced AI tools give cybercriminals more sophisticated attack vectors
- AI-powered social engineering attacks can be more convincing and targeted
- Adversarial attacks can manipulate AI systems into making incorrect decisions
3. Operational and Technical Risks
- “AI hallucinations” producing false or misleading information in critical financial contexts
- Overreliance on AI systems without proper human oversight
- Integration challenges with legacy banking systems
4. Workforce Displacement and Morale
- Automation of traditional banking roles causing employee uncertainty
- Competition for AI talent creating workforce imbalances
- Cultural resistance to AI adoption from established teams
5. Regulatory and Compliance Concerns
- Rapidly evolving AI regulations creating compliance challenges
- Difficulty in explaining AI decisions to regulators (the “black box” problem)
- Cross-border inconsistencies in AI governance frameworks
Solutions and Mitigation Strategies
1. Robust Data Governance
- Implement comprehensive data quality frameworks to ensure AI training data is accurate and representative.
- Regular auditing of datasets for biases and gaps
- Diverse data science teams to catch potential bias issues early
2. Enhanced Security Measures
- AI-powered security systems to detect and respond to threats in real time
- Regular penetration testing specific to AI systems
- Continuous monitoring for unusual AI behavior or outcomes
3. Human-in-the-Loop Approaches
- Maintain human oversight of critical AI decisions
- Clear escalation paths when AI confidence levels are low
- Regular validation of AI outputs against expert human judgment
4. Workforce Transformation
- Reskilling programs to help employees transition to AI-augmented roles
- Creating new positions focused on AI oversight and management
- Clear communication about how AI will complement rather than replace human expertise
5. Explainable AI and Transparency
- Invest in explainable AI techniques that make decision processes clearer
- Documentation of AI model limitations and appropriate use cases
- Client-facing explanations of how AI is used in their financial services
6. Governance Frameworks
- Establish cross-functional AI oversight committees
- Regular risk assessments of AI systems
- Clear policies on AI development, deployment, and monitoring
7. Regulatory Engagement
- Proactive engagement with regulators on AI initiatives
- Participation in industry working groups on AI standards
- Building flexibility into AI systems to adapt to evolving regulations
Banking institutions that balance innovation with thoughtful risk management will be best positioned to leverage AI’s benefits while avoiding its pitfalls. The most successful approach combines technological solutions with organizational change management and strong governance.
AI Risks in Singapore’s Banking Sector and Solutions
Singapore’s Banking Context
Singapore has positioned itself as a global fintech hub with strong regulatory oversight from the Monetary Authority of Singapore (MAS). The city-state’s banking sector has been at the forefront of AI adoption in Asia, with initiatives like:
- DBS Bank’s extensive AI implementation across customer service and operations
- OCBC’s AI-powered fraud detection and Emma chatbot
- UOB’s risk management and personalized banking AI systems
- MAS’s Veritas framework for responsible AI adoption
Key AI Risks for Singapore Banks
1. Data Privacy Concerns in a Strict Regulatory Environment
- Singapore’s Personal Data Protection Act (PDPA) and Banking Act place strict requirements on data handling
- Cross-border data transfers are complicated by Singapore’s role as a regional banking hub
- Cultural expectations of privacy among Singaporean customers
2. Talent Shortage in AI Expertise
- Limited pool of AI specialists in Singapore despite government initiatives
- Competition from other sectors (tech, healthcare) for the same talent
- Immigration policies affecting international recruitment
3. Legacy System Integration Challenges
- Many Singapore banks operate on older core banking systems
- Integration complexity with regional banking operations across ASEAN
- Cost considerations in Singapore’s high-cost business environment
4. Trust and Reputation Management
- Singapore’s banking sector built on reputation for stability and trustworthiness
- AI missteps could damage this reputation more severely than in other markets
- Higher customer expectations for service excellence
Singapore-Specific Solutions
1. Regulatory Alignment and Compliance
- Leverage MAS’s AI Governance Framework and Veritas initiative
- Participate in regulatory sandboxes offered by MAS to test AI systems
- Implement “fairness metrics” as recommended by Singapore’s Model AI Governance Framework
2. Talent Development and Education
- Partner with Singapore universities (NUS, NTU, SMU) for specialized AI banking programs
- Utilize government subsidies through SkillsFuture for employee upskilling
- Develop AI competency centers within banks with knowledge sharing protocols
3. Cultural Integration of AI
- Adopt approaches that reflect Singapore’s multicultural environment
- Design AI systems that can handle Singapore’s multiple official languages
- Balance automation with the high-touch service culture expected in Singapore
4. Regional Leadership Strategy
- Position Singapore-based AI governance as a model for ASEAN
- Develop systems that can adapt to the regulatory environments of neighboring countries
- Create scalable AI solutions that account for Southeast Asian market variations
5. Public-Private Partnerships
- Collaborate with government initiatives like AI Singapore and Singapore Digital (SG)
- Participate in National AI Strategy projects
- Leverage resources from Singapore’s Smart Nation initiative
Implementation Roadmap for Singapore Banks
- Assessment Phase: Evaluate current AI capabilities against MAS guidelines
- Governance Setup: Establish AI ethics committees with Singapore-relevant expertise
- Pilot Programs: Start with low-risk applications aligned with MAS focus areas
- Transparent Communication: Clearly inform Singaporean customers about AI use
- Continuous Monitoring: Regular audits of AI systems for compliance with Singapore standards
Singapore’s advanced digital infrastructure and forward-thinking regulatory approach provide a solid foundation for addressing AI risks in banking. By emphasizing transparency, expertise development, and cultural sensitivity, Singapore banks can implement AI solutions that maintain their customers’ trust and confidence while driving innovation in financial services.
Changing Expectations
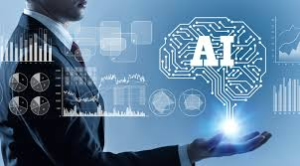
In 2018, 76% of senior banking leaders believed AI would be critical for market differentiation.
Early predictions were dramatic, with some suggesting AI could replace half of bank staff.
The actual impact is turning out to be more nuanced and less disruptive than initially feared.
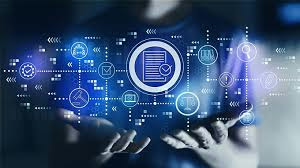
Primary AI Applications in Banking
Customer service improvement
Personalized recommendations based on payment history
Chatbots for handling common customer queries
Seamless handover to human representatives when needed
Optimizing cash flow management
Helping less tech-savvy customers navigate digital banking services
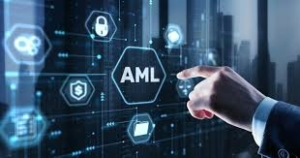
Key Challenges in AI Adoption
Integrating AI with existing legacy banking systems
Accessing and leveraging existing customer data
Avoiding creation of new technological silos
Ensuring smooth, frictionless customer experiences
Innovative Approach Example
The article highlights Auriga’s WinWebServer (WWS) AI module as an innovative solution that:
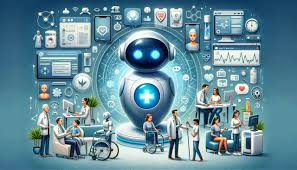
Seamlessly integrates with legacy systems.
Enables enhanced customer experiences
Speeds up strategic decision-making
Helps optimize cash management across bank branches and ATMs
Subtle but Significant Impact
Rather than replacing human workers, AI is primarily being used to augment and improve banking services, making them more personalized, efficient, and accessible.
The article suggests that AI in banking is less about disruption and more about incremental, strategic improvements to existing banking processes and customer interactions.RetryCanalyse ai as transformation not disruptionEditI’ll provide an analysis of AI as a transformative force in banking, expanding on the themes from the article and drawing insights about how AI is fundamentally reshaping the banking landscape.
AI: A Transformative Force in Banking
Conceptual Shift: From Disruption to Transformation
AI represents a fundamental transformation of banking, not merely a disruptive technology. This transformation is characterized by:
1. Intelligent Personalization
- Beyond traditional customer segmentation
- Real-time, individual-level personalization of financial services
- Predictive understanding of customer needs before they arise
- Customized financial advice and product recommendations tailored to individual financial behaviors and life stages
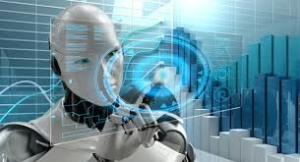
2. Operational Intelligence
- Reimagining banking processes through cognitive automation
- Moving from rule-based systems to adaptive learning systems
- Continuous optimization of internal operations
- Predictive maintenance of financial infrastructure
- Dynamic risk management and compliance monitoring
3. Customer Experience Reimagined
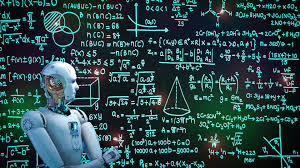
- Shift from transactional interactions to contextual, anticipatory engagement
- 24/7 intelligent support systems
- Seamless omnichannel experiences
- Proactive financial guidance
- Accessibility-driven design that serves diverse customer capabilities
4. Strategic Decision Making
- AI as a strategic partner in executive decision-making
- Advanced scenario modeling
- Real-time market trend analysis
- Enhanced predictive capabilities for investment and risk strategies
- Democratization of sophisticated financial insights
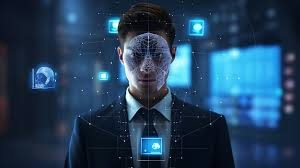
5. Ethical and Inclusive Innovation
- Using AI to address historical banking inequities
- Creating more transparent and fair financial assessment models
- Developing inclusive financial products
- Reducing human bias in financial decision-making
- Supporting underserved financial populations through intelligent design
Transformation Characteristics
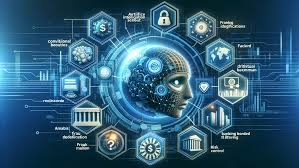
- Evolutionary, Not Revolutionary: Gradual integration that builds upon existing systems
- Augmentative Intelligence: Enhancing human capabilities, not replacing them
- Adaptive Learning: Continuous improvement through sophisticated machine learning
- Holistic Approach: Addressing multiple banking dimensions simultaneously
Future Outlook
The true power of AI in banking lies not in replacing human workers but in creating a symbiotic ecosystem where technological intelligence and human expertise collaborate to deliver unprecedented value.
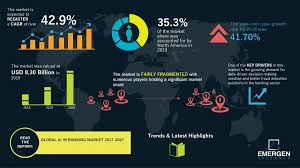
Maxthon
Maxthon has set out on an ambitious journey aimed at significantly bolstering the security of web applications, fueled by a resolute commitment to safeguarding users and their confidential data. At the heart of this initiative lies a collection of sophisticated encryption protocols, which act as a robust barrier for the information exchanged between individuals and various online services. Every interaction—be it the sharing of passwords or personal information—is protected within these encrypted channels, effectively preventing unauthorised access attempts from intruders.
Maxthon private browser for online privacyThis meticulous emphasis on encryption marks merely the initial phase of Maxthon’s extensive security framework. Acknowledging that cyber threats are constantly evolving, Maxthon adopts a forward-thinking approach to user protection. The browser is engineered to adapt to emerging challenges, incorporating regular updates that promptly address any vulnerabilities that may surface. Users are strongly encouraged to activate automatic updates as part of their cybersecurity regimen, ensuring they can seamlessly take advantage of the latest fixes without any hassle.
In today’s rapidly changing digital environment, Maxthon’s unwavering commitment to ongoing security enhancement signifies not only its responsibility toward users but also its firm dedication to nurturing trust in online engagements. With each new update rolled out, users can navigate the web with peace of mind, assured that their information is continuously safeguarded against ever-emerging threats lurking in cyberspace.
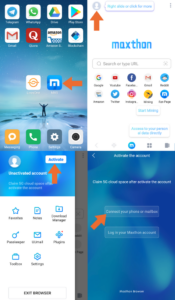